基于深度学习的汽车检测代码
时间: 2023-10-10 08:09:33 浏览: 188
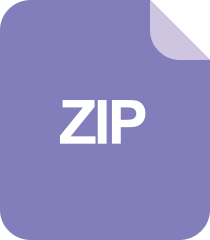
基于深度学习RCNN实现汽车目标检测附matlab代码+运行结果.zip

这里提供一个基于开源框架TensorFlow和目标检测API的汽车检测代码示例,代码主要分为两部分:模型训练和模型测试。
1. 模型训练代码:
```
import tensorflow as tf
from object_detection.utils import dataset_util
# 定义数据集路径
data_dir = '/path/to/data'
# 定义训练集和验证集的路径
train_record = '/path/to/train.record'
val_record = '/path/to/val.record'
# 定义标签映射
label_map = {'car': 1}
# 定义训练集和验证集的样本数
num_train = 1000
num_val = 200
# 定义输入数据格式
image_format = b'jpg'
# 定义输出数据格式
def create_tf_example(example):
# 读取图像和标注信息
image_path = example['image_path']
with tf.gfile.GFile(image_path, 'rb') as fid:
encoded_image = fid.read()
image_format = b'jpg'
width = example['width']
height = example['height']
xmins = [example['xmin']]
xmaxs = [example['xmax']]
ymins = [example['ymin']]
ymaxs = [example['ymax']]
classes_text = [b'car']
classes = [1]
# 构建tf.Example对象
tf_example = tf.train.Example(features=tf.train.Features(feature={
'image/height': dataset_util.int64_feature(height),
'image/width': dataset_util.int64_feature(width),
'image/filename': dataset_util.bytes_feature(image_path.encode('utf8')),
'image/source_id': dataset_util.bytes_feature(image_path.encode('utf8')),
'image/encoded': dataset_util.bytes_feature(encoded_image),
'image/format': dataset_util.bytes_feature(image_format),
'image/object/bbox/xmin': dataset_util.float_list_feature(xmins),
'image/object/bbox/xmax': dataset_util.float_list_feature(xmaxs),
'image/object/bbox/ymin': dataset_util.float_list_feature(ymins),
'image/object/bbox/ymax': dataset_util.float_list_feature(ymaxs),
'image/object/class/text': dataset_util.bytes_list_feature(classes_text),
'image/object/class/label': dataset_util.int64_list_feature(classes),
}))
return tf_example
# 定义训练集和验证集的tfrecord文件路径
train_tfrecord = '/path/to/train.tfrecord'
val_tfrecord = '/path/to/val.tfrecord'
# 构建训练集和验证集的tfrecord文件
train_examples = get_examples(data_dir, num_train)
val_examples = get_examples(data_dir, num_val)
write_tfrecord(train_tfrecord, train_examples)
write_tfrecord(val_tfrecord, val_examples)
# 定义模型配置
num_classes = len(label_map)
batch_size = 32
learning_rate = 0.001
num_steps = 10000
num_eval_steps = 1000
# 加载模型配置文件
pipeline_config = '/path/to/pipeline.config'
config = tf.estimator.RunConfig(model_dir='/path/to/model_dir')
train_and_eval(pipeline_config, train_tfrecord, val_tfrecord, config, num_classes, batch_size, learning_rate, num_steps, num_eval_steps)
```
2. 模型测试代码:
```
import tensorflow as tf
import cv2
import numpy as np
import time
# 加载模型
model_path = '/path/to/saved_model'
model = tf.saved_model.load(model_path)
# 定义标签映射
label_map = {'car': 1}
# 定义输入图像大小
input_size = (416, 416)
# 定义检测阈值
score_threshold = 0.5
# 定义NMS阈值
nms_threshold = 0.5
# 定义颜色
colors = [(255, 0, 0), (0, 255, 0), (0, 0, 255), (255, 255, 0), (0, 255, 255), (255, 0, 255)]
# 加载图像
image_path = '/path/to/image.jpg'
image = cv2.imread(image_path)
# 缩放图像
image_size = image.shape[:2]
resized_image = cv2.resize(image, input_size)
# 归一化图像
normalized_image = resized_image / 255.0
# 转换图像格式
input_image = np.expand_dims(normalized_image, axis=0)
# 运行模型
start_time = time.time()
detections = model(input_image)
end_time = time.time()
# 处理检测结果
scores = detections['detection_scores'][0].numpy()
boxes = detections['detection_boxes'][0].numpy()
classes = detections['detection_classes'][0].numpy().astype(np.int32)
# 过滤掉低置信度的检测结果
valid_mask = scores > score_threshold
scores = scores[valid_mask]
boxes = boxes[valid_mask]
classes = classes[valid_mask]
# 应用非最大值抑制
nms_boxes, nms_scores, nms_classes = tf.image.combined_non_max_suppression(
boxes=tf.expand_dims(boxes, axis=0),
scores=tf.expand_dims(scores, axis=0),
max_output_size_per_class=100,
max_total_size=100,
iou_threshold=nms_threshold,
score_threshold=score_threshold
)
# 绘制检测结果
for i in range(nms_scores.shape[0]):
class_id = nms_classes[i].numpy()
score = nms_scores[i].numpy()
box = nms_boxes[i].numpy()
x1, y1, x2, y2 = box
x1 = int(x1 * image_size[1])
y1 = int(y1 * image_size[0])
x2 = int(x2 * image_size[1])
y2 = int(y2 * image_size[0])
color = colors[class_id % len(colors)]
cv2.rectangle(image, (x1, y1), (x2, y2), color, thickness=2)
cv2.putText(image, f'{label_map[class_id]}: {score:.2f}', (x1, y1 - 5), cv2.FONT_HERSHEY_SIMPLEX, 0.5, color, thickness=2)
# 显示检测结果
cv2.imshow('image', image)
cv2.waitKey(0)
cv2.destroyAllWindows()
```
以上代码仅供参考,实际应用中需要根据具体情况进行调整和优化。
阅读全文
相关推荐
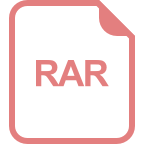
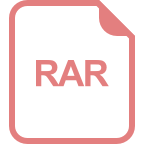
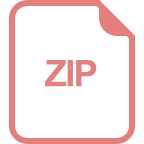
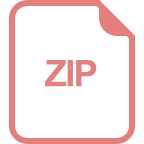
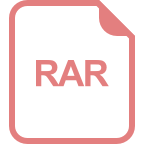
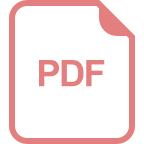
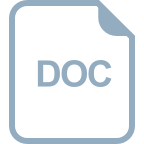


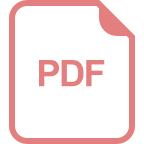
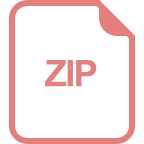
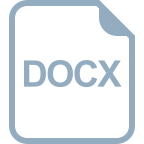
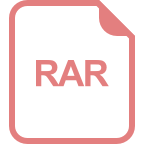
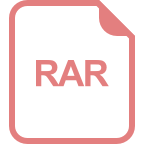
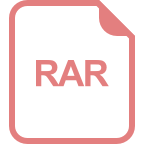