基于深度学习的汽车检测的代码
时间: 2023-10-10 11:10:16 浏览: 47
以下是基于深度学习的汽车检测的Python代码示例:
```python
import cv2
# 加载车辆检测模型
car_cascade = cv2.CascadeClassifier('car_detection.xml')
# 加载图像
img = cv2.imread('cars.jpg')
# 将图像转换为灰度图像
gray = cv2.cvtColor(img, cv2.COLOR_BGR2GRAY)
# 检测车辆
cars = car_cascade.detectMultiScale(gray, 1.1, 1)
# 在图像中绘制矩形框来标识检测到的车辆
for (x, y, w, h) in cars:
cv2.rectangle(img, (x, y), (x+w, y+h), (0, 0, 255), 2)
# 显示图像
cv2.imshow('img', img)
cv2.waitKey()
```
在这个示例中,我们使用OpenCV库中的CascadeClassifier类来加载已经训练好的汽车检测模型。然后,我们加载一张汽车图像,并将其转换为灰度图像。接下来,我们使用detectMultiScale方法来检测图像中的车辆。最后,我们在图像中绘制矩形框来标识检测到的车辆,并显示结果图像。
相关问题
基于深度学习的汽车检测代码
这里提供一个基于开源框架TensorFlow和目标检测API的汽车检测代码示例,代码主要分为两部分:模型训练和模型测试。
1. 模型训练代码:
```
import tensorflow as tf
from object_detection.utils import dataset_util
# 定义数据集路径
data_dir = '/path/to/data'
# 定义训练集和验证集的路径
train_record = '/path/to/train.record'
val_record = '/path/to/val.record'
# 定义标签映射
label_map = {'car': 1}
# 定义训练集和验证集的样本数
num_train = 1000
num_val = 200
# 定义输入数据格式
image_format = b'jpg'
# 定义输出数据格式
def create_tf_example(example):
# 读取图像和标注信息
image_path = example['image_path']
with tf.gfile.GFile(image_path, 'rb') as fid:
encoded_image = fid.read()
image_format = b'jpg'
width = example['width']
height = example['height']
xmins = [example['xmin']]
xmaxs = [example['xmax']]
ymins = [example['ymin']]
ymaxs = [example['ymax']]
classes_text = [b'car']
classes = [1]
# 构建tf.Example对象
tf_example = tf.train.Example(features=tf.train.Features(feature={
'image/height': dataset_util.int64_feature(height),
'image/width': dataset_util.int64_feature(width),
'image/filename': dataset_util.bytes_feature(image_path.encode('utf8')),
'image/source_id': dataset_util.bytes_feature(image_path.encode('utf8')),
'image/encoded': dataset_util.bytes_feature(encoded_image),
'image/format': dataset_util.bytes_feature(image_format),
'image/object/bbox/xmin': dataset_util.float_list_feature(xmins),
'image/object/bbox/xmax': dataset_util.float_list_feature(xmaxs),
'image/object/bbox/ymin': dataset_util.float_list_feature(ymins),
'image/object/bbox/ymax': dataset_util.float_list_feature(ymaxs),
'image/object/class/text': dataset_util.bytes_list_feature(classes_text),
'image/object/class/label': dataset_util.int64_list_feature(classes),
}))
return tf_example
# 定义训练集和验证集的tfrecord文件路径
train_tfrecord = '/path/to/train.tfrecord'
val_tfrecord = '/path/to/val.tfrecord'
# 构建训练集和验证集的tfrecord文件
train_examples = get_examples(data_dir, num_train)
val_examples = get_examples(data_dir, num_val)
write_tfrecord(train_tfrecord, train_examples)
write_tfrecord(val_tfrecord, val_examples)
# 定义模型配置
num_classes = len(label_map)
batch_size = 32
learning_rate = 0.001
num_steps = 10000
num_eval_steps = 1000
# 加载模型配置文件
pipeline_config = '/path/to/pipeline.config'
config = tf.estimator.RunConfig(model_dir='/path/to/model_dir')
train_and_eval(pipeline_config, train_tfrecord, val_tfrecord, config, num_classes, batch_size, learning_rate, num_steps, num_eval_steps)
```
2. 模型测试代码:
```
import tensorflow as tf
import cv2
import numpy as np
import time
# 加载模型
model_path = '/path/to/saved_model'
model = tf.saved_model.load(model_path)
# 定义标签映射
label_map = {'car': 1}
# 定义输入图像大小
input_size = (416, 416)
# 定义检测阈值
score_threshold = 0.5
# 定义NMS阈值
nms_threshold = 0.5
# 定义颜色
colors = [(255, 0, 0), (0, 255, 0), (0, 0, 255), (255, 255, 0), (0, 255, 255), (255, 0, 255)]
# 加载图像
image_path = '/path/to/image.jpg'
image = cv2.imread(image_path)
# 缩放图像
image_size = image.shape[:2]
resized_image = cv2.resize(image, input_size)
# 归一化图像
normalized_image = resized_image / 255.0
# 转换图像格式
input_image = np.expand_dims(normalized_image, axis=0)
# 运行模型
start_time = time.time()
detections = model(input_image)
end_time = time.time()
# 处理检测结果
scores = detections['detection_scores'][0].numpy()
boxes = detections['detection_boxes'][0].numpy()
classes = detections['detection_classes'][0].numpy().astype(np.int32)
# 过滤掉低置信度的检测结果
valid_mask = scores > score_threshold
scores = scores[valid_mask]
boxes = boxes[valid_mask]
classes = classes[valid_mask]
# 应用非最大值抑制
nms_boxes, nms_scores, nms_classes = tf.image.combined_non_max_suppression(
boxes=tf.expand_dims(boxes, axis=0),
scores=tf.expand_dims(scores, axis=0),
max_output_size_per_class=100,
max_total_size=100,
iou_threshold=nms_threshold,
score_threshold=score_threshold
)
# 绘制检测结果
for i in range(nms_scores.shape[0]):
class_id = nms_classes[i].numpy()
score = nms_scores[i].numpy()
box = nms_boxes[i].numpy()
x1, y1, x2, y2 = box
x1 = int(x1 * image_size[1])
y1 = int(y1 * image_size[0])
x2 = int(x2 * image_size[1])
y2 = int(y2 * image_size[0])
color = colors[class_id % len(colors)]
cv2.rectangle(image, (x1, y1), (x2, y2), color, thickness=2)
cv2.putText(image, f'{label_map[class_id]}: {score:.2f}', (x1, y1 - 5), cv2.FONT_HERSHEY_SIMPLEX, 0.5, color, thickness=2)
# 显示检测结果
cv2.imshow('image', image)
cv2.waitKey(0)
cv2.destroyAllWindows()
```
以上代码仅供参考,实际应用中需要根据具体情况进行调整和优化。
深度学习实践汽车检测
深度学习实践汽车检测一般可以使用卷积神经网络(CNN)进行训练和预测。下面是基于TensorFlow和Keras的汽车检测代码示例:
1. 数据准备
首先需要准备一组车辆图像和对应的标签,标签可以是二元变量(0表示无车,1表示有车)或者边界框变量(包含车辆的坐标和大小)。可以从公开数据集中下载或者自己收集数据。
2. 模型搭建
使用Keras搭建卷积神经网络模型,一般可以使用卷积层、池化层、全连接层等网络结构,如下所示:
```python
from keras.models import Sequential
from keras.layers import Conv2D, MaxPooling2D, Flatten, Dense
model = Sequential()
model.add(Conv2D(32, (3, 3), activation='relu', input_shape=(img_width, img_height, 3)))
model.add(MaxPooling2D((2, 2)))
model.add(Conv2D(64, (3, 3), activation='relu'))
model.add(MaxPooling2D((2, 2)))
model.add(Conv2D(128, (3, 3), activation='relu'))
model.add(MaxPooling2D((2, 2)))
model.add(Flatten())
model.add(Dense(64, activation='relu'))
model.add(Dense(1, activation='sigmoid'))
```
在这个示例中,我们使用了3个卷积层和3个最大池化层来提取图像的特征,然后使用两个全连接层来进行分类。最后一层使用sigmoid函数作为激活函数,输出一个二元变量。
3. 模型编译和训练
使用compile函数对模型进行编译,定义损失函数、优化器和评价指标,然后使用fit函数对模型进行训练。如下所示:
```python
model.compile(loss='binary_crossentropy',
optimizer='rmsprop',
metrics=['accuracy'])
model.fit(train_generator,
steps_per_epoch=nb_train_samples // batch_size,
epochs=epochs,
validation_data=validation_generator,
validation_steps=nb_validation_samples // batch_size)
```
在这个示例中,我们使用了二元交叉熵作为损失函数,RMSprop作为优化器,以及准确率作为评价指标。使用fit函数对模型进行训练,设置了训练数据生成器、验证数据生成器、批量大小和训练轮数等参数。
4. 模型预测
使用predict函数对新的图像进行预测,得到二元变量或者边界框变量。如下所示:
```python
from keras.preprocessing import image
import numpy as np
img_path = 'car.jpg'
img = image.load_img(img_path, target_size=(img_width, img_height))
x = image.img_to_array(img)
x = np.expand_dims(x, axis=0)
preds = model.predict(x)
```
在这个示例中,我们首先读取一张新的汽车图像(car.jpg),然后使用load_img函数将图像大小调整为模型的输入大小,使用img_to_array函数将图像转换为张量,最后使用predict函数对模型进行预测,得到一个二元变量。
相关推荐
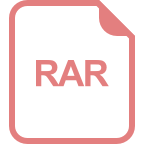
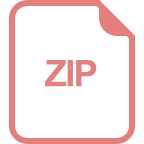












