写一段程序,基于seq2seq的对二值图像进行去噪声处理,图像在minst数据集读取
时间: 2023-06-15 08:03:42 浏览: 99
以下是使用seq2seq模型进行二值图像去噪声处理的示例代码,使用的数据集为MNIST:
```python
from keras.models import Model
from keras.layers import Input, LSTM, Dense
import numpy as np
from keras.datasets import mnist
# 加载MNIST数据集
(x_train, _), (x_test, _) = mnist.load_data()
# 将像素值归一化到0到1之间
x_train = x_train.astype('float32') / 255.
x_test = x_test.astype('float32') / 255.
# 将图像转换成二值图像
threshold = 0.5
x_train[x_train < threshold] = 0.
x_train[x_train >= threshold] = 1.
x_test[x_test < threshold] = 0.
x_test[x_test >= threshold] = 1.
# 添加噪声
noise_factor = 0.5
x_train_noisy = x_train + noise_factor * np.random.normal(loc=0.0, scale=1.0, size=x_train.shape)
x_test_noisy = x_test + noise_factor * np.random.normal(loc=0.0, scale=1.0, size=x_test.shape)
x_train_noisy = np.clip(x_train_noisy, 0., 1.)
x_test_noisy = np.clip(x_test_noisy, 0., 1.)
# 将图像转换为序列
seq_length = x_train.shape[1] * x_train.shape[2]
x_train_noisy_seq = x_train_noisy.reshape((len(x_train_noisy), seq_length))
x_test_noisy_seq = x_test_noisy.reshape((len(x_test_noisy), seq_length))
x_train_seq = x_train.reshape((len(x_train), seq_length))
x_test_seq = x_test.reshape((len(x_test), seq_length))
# 定义编码器模型
latent_dim = 32
encoder_inputs = Input(shape=(seq_length,))
encoder = LSTM(latent_dim)(encoder_inputs)
# 定义解码器模型
decoder_inputs = Input(shape=(seq_length,))
decoder = LSTM(latent_dim, return_sequences=True)(decoder_inputs)
decoder_outputs = Dense(seq_length, activation='sigmoid')(decoder)
# 定义seq2seq模型
sequence_autoencoder = Model([encoder_inputs, decoder_inputs], decoder_outputs)
sequence_autoencoder.compile(optimizer='adam', loss='binary_crossentropy')
# 训练模型
sequence_autoencoder.fit([x_train_noisy_seq, x_train_seq], x_train_seq,
epochs=50,
batch_size=128,
shuffle=True,
validation_data=([x_test_noisy_seq, x_test_seq], x_test_seq))
# 对测试集进行去噪声处理
decoded_imgs = sequence_autoencoder.predict([x_test_noisy_seq, x_test_seq])
# 显示去噪后的图像
import matplotlib.pyplot as plt
n = 10 # 显示前10个图像
plt.figure(figsize=(20, 4))
for i in range(n):
# 显示原始图像
ax = plt.subplot(2, n, i + 1)
plt.imshow(x_test_noisy[i].reshape(28, 28))
plt.gray()
ax.get_xaxis().set_visible(False)
ax.get_yaxis().set_visible(False)
# 显示去噪后的图像
ax = plt.subplot(2, n, i + 1 + n)
plt.imshow(decoded_imgs[i].reshape(28, 28))
plt.gray()
ax.get_xaxis().set_visible(False)
ax.get_yaxis().set_visible(False)
plt.show()
```
在这个示例代码中,我们首先将图像转换为二值图像,并添加噪声。然后将图像转换为序列,并将序列输入到seq2seq模型中进行训练。最后,我们对测试集进行去噪声处理,并将去噪后的图像与原始图像进行比较。
阅读全文
相关推荐
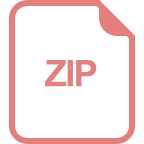
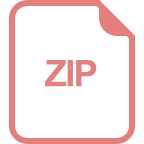
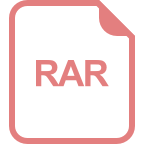

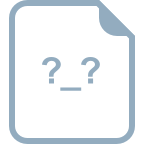
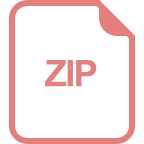
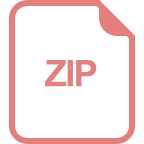
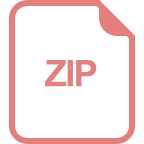
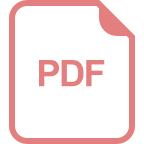
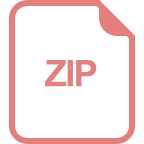
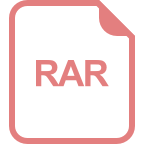
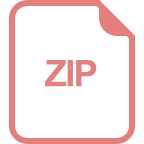
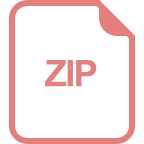
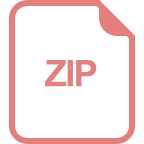
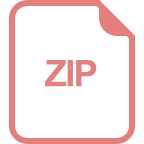