基于hadoop mapreduce实现TF-IDF。
时间: 2023-11-14 20:13:00 浏览: 87
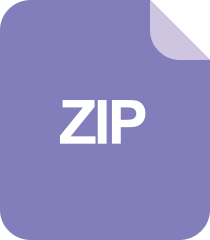
基于MapReduce实现的TFIDF计算
TF-IDF(Term Frequency-Inverse Document Frequency)是一种用于信息检索和文本挖掘的常用技术,它能够评估一个词语在一个文档集合中的重要程度。
在Hadoop MapReduce框架下实现TF-IDF,需要完成以下步骤:
1. 计算每个文档中每个单词出现的次数(Term Frequency,即TF)。
2. 计算每个单词在整个文档集合中出现的文档数(Inverse Document Frequency,即IDF)。
3. 计算每个单词在每个文档中的TF-IDF值。
下面是一个基于Hadoop MapReduce实现TF-IDF的示例:
1. 计算每个文档中每个单词出现的次数
首先,我们需要将文档集合分成若干个小文件,每个小文件包含若干个文档。在Map阶段,我们需要将每个小文件中的每个文档转换成键值对形式,其中键为文档ID,值为文档内容。然后,在Reduce阶段,我们需要对每个文档进行分词,并计算每个单词在该文档中出现的次数。
Map阶段:
```java
public class TFMapper extends Mapper<LongWritable, Text, Text, Text> {
private Text docID = new Text();
private Text wordCount = new Text();
public void map(LongWritable key, Text value, Context context) throws IOException, InterruptedException {
String[] parts = value.toString().split("\\t");
String docContent = parts[1];
String[] words = docContent.split(" ");
Map<String, Integer> wordCounts = new HashMap<String, Integer>();
for (String word : words) {
if (wordCounts.containsKey(word)) {
wordCounts.put(word, wordCounts.get(word) + 1);
} else {
wordCounts.put(word, 1);
}
}
for (String word : wordCounts.keySet()) {
docID.set(parts[0]);
wordCount.set(word + ":" + wordCounts.get(word));
context.write(docID, wordCount);
}
}
}
```
Reduce阶段:
```java
public class TFReducer extends Reducer<Text, Text, Text, Text> {
private Text wordCount = new Text();
public void reduce(Text key, Iterable<Text> values, Context context) throws IOException, InterruptedException {
Map<String, Integer> wordCounts = new HashMap<String, Integer>();
for (Text value : values) {
String[] parts = value.toString().split(":");
String word = parts[0];
int count = Integer.parseInt(parts[1]);
if (wordCounts.containsKey(word)) {
wordCounts.put(word, wordCounts.get(word) + count);
} else {
wordCounts.put(word, count);
}
}
StringBuilder sb = new StringBuilder();
for (String word : wordCounts.keySet()) {
sb.append(word + ":" + wordCounts.get(word) + " ");
}
wordCount.set(sb.toString());
context.write(key, wordCount);
}
}
```
2. 计算每个单词在整个文档集合中出现的文档数
在Map阶段,我们需要将每个文档中的单词转换成键值对形式,其中键为单词,值为文档ID。然后,在Reduce阶段,我们需要对每个单词进行统计,得到每个单词在多少个文档中出现过。
Map阶段:
```java
public class IDFMapper extends Mapper<LongWritable, Text, Text, Text> {
private Text word = new Text();
private Text docID = new Text();
public void map(LongWritable key, Text value, Context context) throws IOException, InterruptedException {
String[] parts = value.toString().split("\\t");
String[] words = parts[1].split(" ");
for (String w : words) {
word.set(w);
docID.set(parts[0]);
context.write(word, docID);
}
}
}
```
Reduce阶段:
```java
public class IDFReducer extends Reducer<Text, Text, Text, DoubleWritable> {
private DoubleWritable idf = new DoubleWritable();
public void reduce(Text key, Iterable<Text> values, Context context) throws IOException, InterruptedException {
Set<String> docs = new HashSet<String>();
for (Text value : values) {
docs.add(value.toString());
}
double df = docs.size();
double N = context.getConfiguration().getLong("totalDocs", 1L);
double idfValue = Math.log(N / df);
idf.set(idfValue);
context.write(key, idf);
}
}
```
3. 计算每个单词在每个文档中的TF-IDF值
在Map阶段,我们需要将每个文档中的单词转换成键值对形式,其中键为文档ID和单词,值为单词在该文档中出现的次数和该单词的IDF值。然后,在Reduce阶段,我们需要对每个文档中的所有单词进行统计,得到每个单词在该文档中的TF-IDF值。
Map阶段:
```java
public class TFIDFMapper extends Mapper<LongWritable, Text, Text, Text> {
private Text docID = new Text();
private Text wordCountIDF = new Text();
public void map(LongWritable key, Text value, Context context) throws IOException, InterruptedException {
String[] parts = value.toString().split("\\t");
String[] wordCounts = parts[1].split(" ");
for (String wc : wordCounts) {
String[] subParts = wc.split(":");
String word = subParts[0];
int count = Integer.parseInt(subParts[1]);
double idf = Double.parseDouble(subParts[2]);
docID.set(parts[0] + ":" + word);
wordCountIDF.set(count + ":" + idf);
context.write(docID, wordCountIDF);
}
}
}
```
Reduce阶段:
```java
public class TFIDFReducer extends Reducer<Text, Text, Text, DoubleWritable> {
private DoubleWritable tfidf = new DoubleWritable();
public void reduce(Text key, Iterable<Text> values, Context context) throws IOException, InterruptedException {
int count = 0;
double idf = 0.0;
for (Text value : values) {
String[] parts = value.toString().split(":");
count += Integer.parseInt(parts[0]);
idf = Double.parseDouble(parts[1]);
}
tfidf.set(count * idf);
context.write(key, tfidf);
}
}
```
最后,在Driver中将上述三个阶段串联起来,即可完成TF-IDF的计算。
```java
public class TFIDFDriver {
public static void main(String[] args) throws Exception {
Configuration conf = new Configuration();
Job job1 = Job.getInstance(conf, "TF");
job1.setJarByClass(TFIDFDriver.class);
job1.setInputFormatClass(TextInputFormat.class);
job1.setOutputFormatClass(TextOutputFormat.class);
job1.setMapperClass(TFMapper.class);
job1.setCombinerClass(TFReducer.class);
job1.setReducerClass(TFReducer.class);
job1.setOutputKeyClass(Text.class);
job1.setOutputValueClass(Text.class);
FileInputFormat.addInputPath(job1, new Path(args[0]));
FileOutputFormat.setOutputPath(job1, new Path(args[1]));
job1.waitForCompletion(true);
Job job2 = Job.getInstance(conf, "IDF");
job2.setJarByClass(TFIDFDriver.class);
job2.setInputFormatClass(TextInputFormat.class);
job2.setOutputFormatClass(TextOutputFormat.class);
job2.setMapperClass(IDFMapper.class);
job2.setReducerClass(IDFReducer.class);
job2.setOutputKeyClass(Text.class);
job2.setOutputValueClass(DoubleWritable.class);
FileInputFormat.addInputPath(job2, new Path(args[1]));
FileOutputFormat.setOutputPath(job2, new Path(args[2]));
job2.getConfiguration().setLong("totalDocs", job2.getCounters().findCounter("org.apache.hadoop.mapred.Task$Counter", "MAP_INPUT_RECORDS").getValue());
job2.waitForCompletion(true);
Job job3 = Job.getInstance(conf, "TF-IDF");
job3.setJarByClass(TFIDFDriver.class);
job3.setInputFormatClass(TextInputFormat.class);
job3.setOutputFormatClass(TextOutputFormat.class);
job3.setMapperClass(TFIDFMapper.class);
job3.setReducerClass(TFIDFReducer.class);
job3.setOutputKeyClass(Text.class);
job3.setOutputValueClass(DoubleWritable.class);
FileInputFormat.addInputPath(job3, new Path(args[1]));
FileOutputFormat.setOutputPath(job3, new Path(args[3]));
job3.waitForCompletion(true);
}
}
```
以上就是基于Hadoop MapReduce实现TF-IDF的方法。
阅读全文
相关推荐
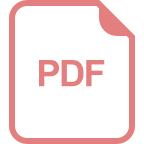

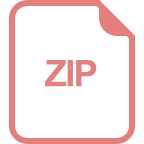
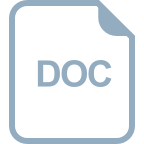
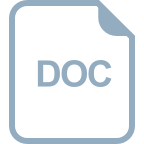
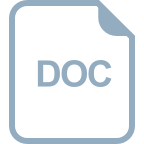
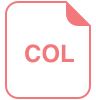
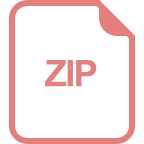
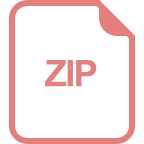
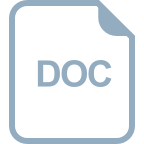
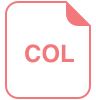
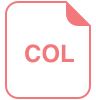

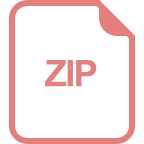
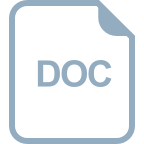