tf.contrib.memory_stats.MaxBytesInUse
时间: 2024-05-20 19:16:07 浏览: 139
As an AI language model, I do not have access to the latest TensorFlow version. However, based on my understanding, `tf.contrib.memory_stats.MaxBytesInUse` is a TensorFlow function used for tracking the maximum number of bytes used in the current session. It is a part of the `tf.contrib.memory_stats` module which provides statistics related to memory usage in TensorFlow. The function returns a scalar tensor representing the maximum number of bytes used by tensors in the current session. This can be useful for monitoring memory usage and optimizing the performance of TensorFlow applications.
相关问题
tf.contrib.memory_stats.MaxBytesInUse有什么用
tf.contrib.memory_stats.MaxBytesInUse是TensorFlow的一个函数,用于返回当前TensorFlow进程所使用的最大内存(以字节为单位)。它可以用于监测TensorFlow程序的内存使用情况,有助于发现内存泄漏等问题。可以在程序的某个关键点调用该函数,以获取最大内存使用量的信息。
详细分析代码“conv2 = tf.contrib.layers.convolution2d(pool1 ,N_FILTERS ,FILTER_SHAPE2 ,padding='VALID') # 抽取特征 pool2 = tf.squeeze(tf.reduce_max(conv2, 1), squeeze_dims=[1]) # 全连接层 logits = tf.contrib.layers.fully_connected(pool2, 15, activation_fn=None) loss = tf.losses.softmax_cross_entropy(target, logits) #多分类交叉熵损失 # 优化器 train_op = tf.contrib.layers.optimize_loss(loss 每一句代码的详细作用,用了什么函数什么参数有什么作用,什么含义,并添加详细注释 ,tf.contrib.framework.get_global_step() ,optimizer='Adam' ,learning_rate=0.01) return ({ 'class': tf.argmax(logits, 1), 'prob': tf.nn.softmax(logits) }, loss, train_op)”
这段代码是一个基于卷积神经网络的分类器模型,以下是每一行代码的详细解释:
```
conv2 = tf.contrib.layers.convolution2d(pool1, N_FILTERS, FILTER_SHAPE2, padding='VALID')
```
使用 `tf.contrib.layers.convolution2d` 函数对 `pool1` 进行卷积操作,其中 `N_FILTERS` 表示卷积核的数量,`FILTER_SHAPE2` 是一个 2 维的列表,表示卷积核的大小,`padding='VALID'` 表示边缘不填充。
```
pool2 = tf.squeeze(tf.reduce_max(conv2, 1), squeeze_dims=[1])
```
对 `conv2` 进行池化操作,使用 `tf.reduce_max` 函数对每个卷积核的输出进行最大化,再使用 `tf.squeeze` 函数将第二维的大小为 1 的维度去掉。
```
logits = tf.contrib.layers.fully_connected(pool2, 15, activation_fn=None)
```
使用 `tf.contrib.layers.fully_connected` 函数连接一个全连接层,其中 `pool2` 是输入,`15` 是输出的类别数,`None` 表示没有激活函数。
```
loss = tf.losses.softmax_cross_entropy(target, logits)
```
计算损失,使用 `tf.losses.softmax_cross_entropy` 函数计算多分类交叉熵损失,其中 `target` 是目标标签,`logits` 是模型的输出。
```
train_op = tf.contrib.layers.optimize_loss(loss, tf.contrib.framework.get_global_step(), optimizer='Adam', learning_rate=0.01)
```
使用 `tf.contrib.layers.optimize_loss` 函数进行优化,其中 `loss` 是损失函数,`tf.contrib.framework.get_global_step()` 获取全局步数,`optimizer='Adam'` 使用 Adam 优化器,`learning_rate=0.01` 设置学习率。
```
return ({
'class': tf.argmax(logits, 1),
'prob': tf.nn.softmax(logits)
}, loss, train_op)
```
返回一个字典,包含了模型的输出和损失,其中 `'class'` 表示预测的类别,使用 `tf.argmax` 函数取最大值的索引作为预测结果,`'prob'` 表示预测的概率,使用 `tf.nn.softmax` 函数进行归一化处理。
阅读全文
相关推荐








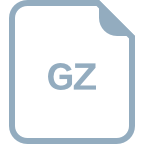
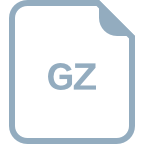
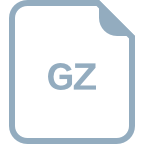
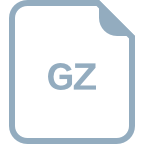


