mtcnn代码实现
时间: 2023-08-11 20:06:44 浏览: 205
MTCNN(Multi-task Cascaded Convolutional Networks)是一种用于人脸检测的深度神经网络。MTCNN使用了一个三级级联的CNN进行人脸检测。以下是MTCNN的代码实现。
首先,需要导入必要的库和模型:
```python
import cv2
import numpy as np
from keras.models import load_model
PNet = load_model('PNet.h5')
RNet = load_model('RNet.h5')
ONet = load_model('ONet.h5')
```
接下来,定义一个函数来进行人脸检测:
```python
def detect_faces(image):
img = image.copy()
img = cv2.cvtColor(img, cv2.COLOR_BGR2RGB)
height, width = img.shape[:2]
# 图像预处理
img_resized = cv2.resize(img, (int(width/2), int(height/2)))
img_resized = (img_resized - 127.5) / 128.0
img_resized = np.expand_dims(img_resized, axis=0)
# PNet 预测
threshold = 0.7
scale_factor = 0.709
scales = []
bounding_boxes = []
while min(img_resized.shape[:2]) > 12:
scales.append(np.array([img_resized.shape[1]/width, img_resized.shape[0]/height]))
output = PNet.predict(img_resized)
cls_prob = output[0][:,:,1]
bbox_pred = output[1]
indices = np.where(cls_prob > threshold)
indices = np.stack(indices, axis=1)
if indices.shape[0] == 0:
img_resized = cv2.resize(img_resized, (int(img_resized.shape[1]*scale_factor), int(img_resized.shape[0]*scale_factor)))
continue
for index in indices:
xmin = index[1] * 2
ymin = index[0] * 2
xmax = index[1] * 2 + 12
ymax = index[0] * 2 + 12
score = cls_prob[index[0], index[1]]
offset = bbox_pred[index[0], index[1]]
bounding_boxes.append([xmin, ymin, xmax, ymax, score, offset])
img_resized = cv2.resize(img_resized, (int(img_resized.shape[1]*scale_factor), int(img_resized.shape[0]*scale_factor)))
# NMS 处理
bounding_boxes = np.array(bounding_boxes)
keep = nms(bounding_boxes[:, :5], 0.5)
bounding_boxes = bounding_boxes[keep]
# RNet 预测
threshold = 0.7
scales = []
for b in bounding_boxes:
w = b[2] - b[0]
h = b[3] - b[1]
size = max(w, h)
img_sub = img[b[1]:b[3], b[0]:b[2]]
img_resized = cv2.resize(img_sub, (24, 24))
img_resized = (img_resized - 127.5) / 128.0
img_resized = np.expand_dims(img_resized, axis=0)
scales.append(size/24)
output = RNet.predict(img_resized)
cls_prob = output[0][:,1]
bbox_pred = output[1]
if cls_prob > threshold:
score = cls_prob
offset = bbox_pred
xmin = int(b[0] + offset[0]*w)
ymin = int(b[1] + offset[1]*h)
xmax = int(b[2] + offset[2]*w)
ymax = int(b[3] + offset[3]*h)
bounding_boxes.append([xmin, ymin, xmax, ymax, score])
# NMS 处理
bounding_boxes = np.array(bounding_boxes)
keep = nms(bounding_boxes[:, :5], 0.7, 'iom')
bounding_boxes = bounding_boxes[keep]
# ONet 预测
threshold = 0.7
faces = []
for b in bounding_boxes:
w = b[2] - b[0]
h = b[3] - b[1]
size = max(w, h)
img_sub = img[b[1]:b[3], b[0]:b[2]]
img_resized = cv2.resize(img_sub, (48, 48))
img_resized = (img_resized - 127.5) / 128.0
img_resized = np.expand_dims(img_resized, axis=0)
output = ONet.predict(img_resized)
cls_prob = output[0][:,1]
bbox_pred = output[1]
landmark_pred = output[2]
if cls_prob > threshold:
score = cls_prob
offset = bbox_pred
landmark = landmark_pred
xmin = int(b[0] + offset[0]*w)
ymin = int(b[1] + offset[1]*h)
xmax = int(b[2] + offset[2]*w)
ymax = int(b[3] + offset[3]*h)
x1 = xmin + landmark[0]*w
y1 = ymin + landmark[1]*h
x2 = xmin + landmark[2]*w
y2 = ymin + landmark[3]*h
x3 = xmin + landmark[4]*w
y3 = ymin + landmark[5]*h
x4 = xmin + landmark[6]*w
y4 = ymin + landmark[7]*h
faces.append([xmin, ymin, xmax, ymax, score, x1, y1, x2, y2, x3, y3, x4, y4])
return faces
```
其中,nms函数实现了非极大值抑制:
```python
def nms(dets, thresh, method='union'):
x1 = dets[:, 0]
y1 = dets[:, 1]
x2 = dets[:, 2]
y2 = dets[:, 3]
scores = dets[:, 4]
areas = (x2 - x1 + 1) * (y2 - y1 + 1)
order = scores.argsort()[::-1]
keep = []
while order.size > 0:
i = order[0]
keep.append(i)
xx1 = np.maximum(x1[i], x1[order[1:]])
yy1 = np.maximum(y1[i], y1[order[1:]])
xx2 = np.minimum(x2[i], x2[order[1:]])
yy2 = np.minimum(y2[i], y2[order[1:]])
w = np.maximum(0.0, xx2 - xx1 + 1)
h = np.maximum(0.0, yy2 - yy1 + 1)
inter = w * h
if method == 'union':
ovr = inter / (areas[i] + areas[order[1:]] - inter)
elif method == 'min':
ovr = inter / np.minimum(areas[i], areas[order[1:]])
else:
print('Unknown nms method!')
inds = np.where(ovr <= thresh)[0]
order = order[inds + 1]
return keep
```
最后,可以使用以下代码来进行人脸检测:
```python
image = cv2.imread('test.jpg')
faces = detect_faces(image)
for face in faces:
xmin, ymin, xmax, ymax = face[:4]
cv2.rectangle(image, (xmin, ymin), (xmax, ymax), (0, 0, 255), 2)
for i in range(5, 13, 2):
x, y = int(face[i]), int(face[i+1])
cv2.circle(image, (x, y), 2, (0, 0, 255), -1)
cv2.imshow('image', image)
cv2.waitKey(0)
cv2.destroyAllWindows()
```
阅读全文
相关推荐
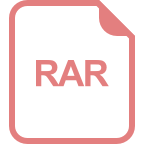
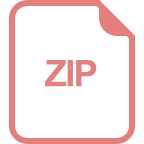
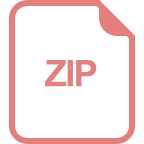
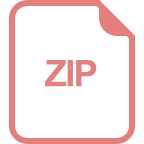
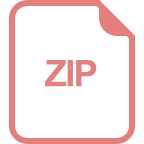
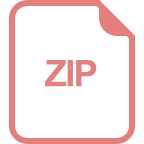
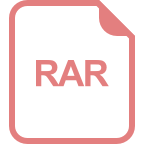
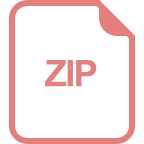
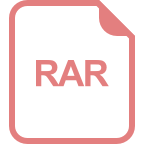
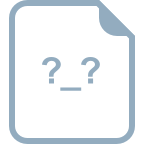
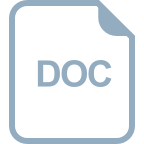
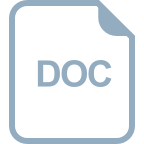




