def UNet_wiener(height, width, initial_psf, initial_K, encoding_cs=[24, 64, 128, 256, 512, 1024], center_cs=1024, decoding_cs=[512, 256, 128, 64, 24, 24], skip_connections=[True, True, True, True, True, True]): inputs = tf.keras.Input((height, width, 1)) x = inputs # Multi-Wiener deconvolutions x = WienerDeconvolution(initial_psf, initial_K)(x) skips = [] # Contracting path for c in encoding_cs: x, x_skip = encoder_block(x, c, kernel_size=3, padding='same', dilation_rate=1, pooling='average') skips.append(x_skip) skips = list(reversed(skips)) # Center x = residual_block(x, center_cs, kernel_size=3, padding='same') # Expansive path for i, c in enumerate(decoding_cs): if skip_connections[i]: x = decoder_block_resize(x, skips[i], c, kernel_size=3, padding='same', dilation_rate=1) else: x = decoder_block(x, None, c, kernel_size=3, padding='same', dilation_rate=1) # Classify x = layers.Conv2D(filters=1, kernel_size=1, use_bias=True, activation='relu')(x) outputs = tf.squeeze(x, axis=3) model = tf.keras.Model(inputs=[inputs], outputs=[outputs]) return model
时间: 2023-12-07 14:04:04 浏览: 74
这段代码看起来像是一个基于 UNet 的神经网络模型,用于图像去噪。其中包括了一个 Multi-Wiener Deconvolution 模块,用于去除图像中的模糊和噪声。模型的编码器和解码器都使用了卷积层和反卷积层,并且采用了 skip connections 技术,以帮助信息传递和提高模型性能。最后的输出是一个二维图像,经过了 squeeze 操作,去掉了 channel 维度,变成了一个灰度图像。
相关问题
def UNet_wiener(height, width, initial_psf, initial_K, encoding_cs=[24, 64, 128, 256, 512, 1024,], center_cs=1024, decoding_cs=[512, 256, 128, 64, 24, 24], skip_connections=[True, True, True, True, True, True]):
这是一个基于U-Net结构的图像去模糊网络实现,其中包含编码和解码路径。编码路径包含多个卷积层,每个卷积层都包含卷积、批归一化和激活函数。解码路径也包含多个卷积层,每个卷积层也包含卷积、批归一化和激活函数。在解码路径中,还包含跳跃连接,用于将编码路径的特征图与解码路径的特征图合并。此外,该网络还包含一个Wiener滤波层,用于估计图像的PSF和噪声水平。整个网络的输出是重建的图像。该网络可用于图像去模糊等任务中。
def UNet_multiwiener_resize(height, width, initial_psfs, initial_Ks, encoding_cs=[24, 64, 128, 256, 512, 1024], center_cs=1024, decoding_cs=[512, 256, 128, 64, 24, 24], skip_connections=[True, True, True, True, True, True])
这是一个函数定义,它的作用是创建一个基于 UNet 架构的多维维纳滤波器调整大小模型。具体来说,它使用给定的初始 PSFs 和 Ks 对图像进行维纳滤波器处理,然后使用 UNet 架构进行图像调整大小。该函数接受的参数包括图像的高度和宽度、初始 PSFs 和 Ks、编码器和解码器中每个层的通道数、中心层的通道数以及是否使用跳跃连接。
阅读全文
相关推荐
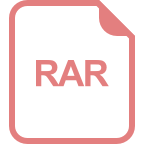
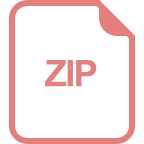


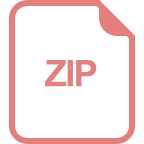
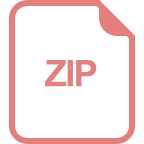
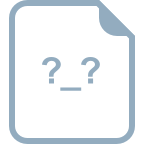
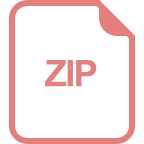
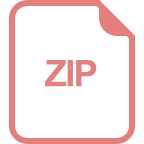
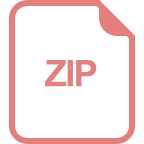
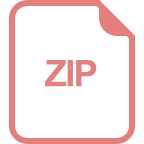
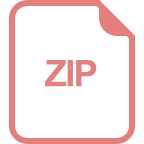
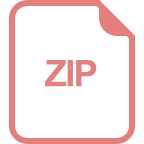
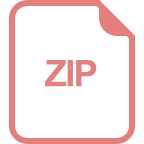
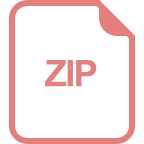