用python写一个基于注意力机制的CNN-BiLSTM时间序列预测模型代码
时间: 2024-05-01 22:18:05 浏览: 282
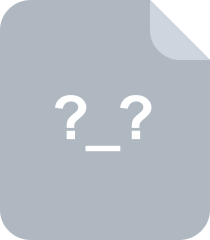
注意力机制代码 python
当然,让我来为您提供这个代码,但是注意,这仅仅是一个示例,您需要根据自己的需求进行修改和调整。
```python
# 导入必要的模块
import numpy as np
import pandas as pd
import matplotlib.pyplot as plt
from keras.models import Model, Sequential
from keras.layers import Input, Dense, Dropout, Flatten, TimeDistributed, Masking, LSTM, Bidirectional, Conv1D, MaxPooling1D, Attention
from keras.optimizers import Adam
from sklearn.model_selection import train_test_split
from sklearn.preprocessing import StandardScaler
# 读取数据
data = pd.read_csv('data.csv')
# 数据预处理
scaler = StandardScaler()
data = scaler.fit_transform(data)
X = []
y = []
seq_len = 50 # 序列长度
for i in range(seq_len, len(data)):
X.append(data[i-seq_len:i])
y.append(data[i])
X = np.array(X)
y = np.array(y)
X_train, X_test, y_train, y_test = train_test_split(X, y, test_size=0.1, shuffle=False)
# 构建模型
inputs = Input(shape=(seq_len, X.shape[2]))
x = Masking()(inputs)
x = Conv1D(32, 3, padding='same', activation='relu')(x)
x = MaxPooling1D()(x)
x = Conv1D(64, 3, padding='same', activation='relu')(x)
x = MaxPooling1D()(x)
x = Bidirectional(LSTM(64, return_sequences=True))(x)
x = Attention()(x)
x = TimeDistributed(Dense(64))(x)
x = Dropout(0.5)(x)
x = Flatten()(x)
x = Dense(1, activation='linear')(x)
model = Model(inputs=inputs, outputs=x)
model.compile(optimizer=Adam(), loss='mean_squared_error')
model.summary()
# 训练模型
history = model.fit(X_train, y_train, batch_size=64, epochs=50, validation_data=(X_test, y_test))
# 评估模型
mse_train = model.evaluate(X_train, y_train)
mse_test = model.evaluate(X_test, y_test)
print('Train MSE: %f' % mse_train)
print('Test MSE: %f' % mse_test)
# 可视化结果
plt.plot(history.history['loss'], label='train')
plt.plot(history.history['val_loss'], label='test')
plt.legend()
plt.show()
```
希望这个代码可以帮助到您,如果还有其他问题或需求,请随时告诉我。
阅读全文
相关推荐
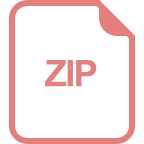
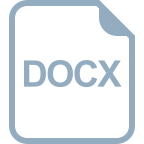
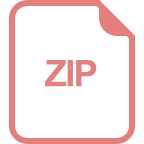
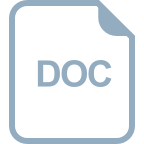
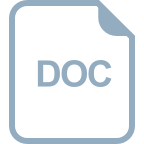
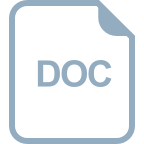
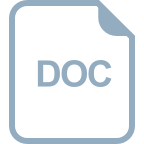
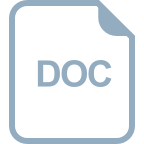
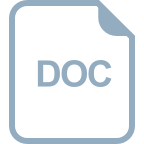
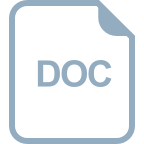
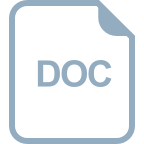
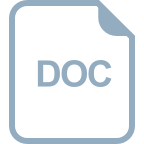
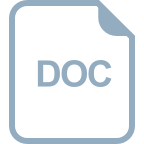
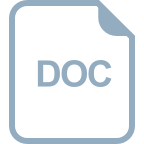
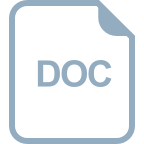
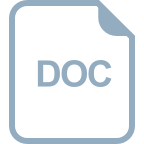
