# walk-forwardvalidation for univariate data defwalk_forward_validation(data, n_test): predictions = list() # split dataset train, test = train_test_split(data,n_test) # seed history with training dataset history = [x for x in train] # step over each time-step in the testset for i in range(len(test)): # split test row into input andoutput columns testX, testy = test[i, :-1],test[i, -1] # fit model on history and make aprediction yhat = xgboost_forecast(history,testX) # store forecast in list ofpredictions predictions.append(yhat) # add actual observation tohistory for the next loop history.append(test[i]) # summarize progress print('>expected=%.1f,predicted=%.1f' % (testy, yhat)) # estimate prediction error error = mean_absolute_error(test[:, 1],predictions) return error, test[:, 1], predictions
时间: 2024-02-14 19:35:23 浏览: 23
这是一个用于单变量数据的走步验证(walk-forward validation)函数,其中包括以下步骤:
1. 将数据集分为训练集和测试集
2. 在训练集上训练模型,并在测试集上进行预测
3. 将预测结果存储在一个列表中
4. 为下一次循环将实际观测值添加到历史记录中
5. 计算预测误差并返回误差、测试集真实值和预测值
在该函数中,使用了 XGBoost 模型进行预测。
相关问题
> ts_data_interp <- na.interp(ts_data_with_na) Error in na.interp(ts_data_with_na) : The time series is not univariate.
非常抱歉,我再次犯了错误。`na.interp()`函数只能用于处理单变量时间序列,而`ts_data_with_na`是一个多变量时间序列。
如果要处理多变量时间序列的缺失值,可以使用`imputeTS`包中的`na_kalman()`函数、`na_ma()`函数或者`na_seadec()`函数等方法。下面是使用`na_kalman()`函数进行缺失值插值的示例代码:
```R
# 加载需要用到的包
library(forecast)
library(imputeTS)
# 生成一个带有异常值的时间序列
set.seed(123)
ts_data <- rnorm(50)
ts_data[25] <- 10
# 绘制时间序列图
plot(ts_data)
# 使用3σ原则检测异常值
sd_value <- sd(ts_data)
mean_value <- mean(ts_data)
threshold_upper <- mean_value + 3 * sd_value
threshold_lower <- mean_value - 3 * sd_value
outliers <- which(ts_data > threshold_upper | ts_data < threshold_lower)
print(outliers)
# 删除异常值
ts_data_no_outliers <- ts_data[-outliers]
plot(ts_data_no_outliers)
# 使用线性插值填补缺失值
ts_data_with_na <- ts_data_no_outliers
ts_data_with_na[c(10, 20)] <- NA
ts_data_interp <- na_kalman(ts_data_with_na)
plot(ts_data_interp)
```
以上代码中,我们使用`imputeTS`包中的`na_kalman()`函数对缺失值进行插值。需要注意的是,`na_kalman()`函数默认使用卡尔曼滤波进行插值,可以根据需要选择其他方法。
Error in adf.test(data) : x is not a vector or univariate time series
这个错误提示意味着你传递给 `adf.test` 函数的数据 `data` 不是一个向量或者单变量的时间序列。
这个问题可能有多种原因,下面列举一些可能的情况和解决方法:
1. `data` 不是一个向量。你可以使用 `is.vector` 函数检查一下数据是否为向量。如果不是,可以使用 `as.vector` 函数将其转换为向量。
2. `data` 是一个矩阵或数据框,且包含多列数据。`adf.test` 函数只能接受单变量的时间序列作为输入。你需要从数据框中选择一列数据作为输入,例如:
```R
# 假设 data 是一个数据框,其中包含多列数据
x <- data$col1 # 选择其中一列作为输入
adf.test(x) # 进行单位根检验
```
3. `data` 是一个多维数组或列表。`adf.test` 函数只能接受一维的向量作为输入。你需要使用 `as.vector` 函数将其转换为向量,例如:
```R
# 假设 data 是一个二维数组
x <- as.vector(data)
adf.test(x) # 进行单位根检验
```
如果以上方法都无法解决问题,你可以尝试在 R 中使用 `str` 函数查看数据的结构,以确定问题的具体原因。
相关推荐
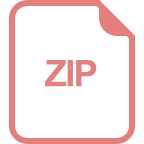
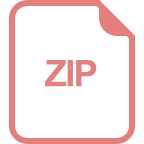
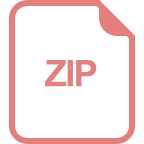












