torch.arange()参数中requires_grad的作用是什么
时间: 2024-06-05 22:08:51 浏览: 6
`torch.arange()` 函数用于创建一个一维张量,其中包含一个等差数列,它的参数 `requires_grad` 是用来指定张量是否需要求导的。如果设置为 True,那么在这个张量上的操作会被记录在计算图中,从而可以进行自动求导。如果设置为 False,则不会进行求导。
举个例子,如果我们想要对一个张量进行梯度下降优化,那么就需要将 `requires_grad` 设置为 True。在训练过程中,每次计算损失函数时,PyTorch 会自动构建计算图,并计算张量的梯度,最后通过反向传播算法更新模型参数,从而使损失函数最小化。如果不需要求导,那么就可以将 `requires_grad` 设置为 False,这样可以提高计算效率。
相关问题
Variable._execution_engine.run_backward( # Calls into the C++ engine to run the backward pass RuntimeError: element 0 of tensors does not require grad and does not have a grad_fn : class CustomLoss(nn.Module): def __init__(self): super(CustomLoss, self).__init__() def forward(self, predicted_tokens, target_tokens): # 设置允许梯度计算 predicted_tokens.requires_grad = True target_tokens.requires_grad = True scores = torch.zeros_like(target_tokens, dtype=torch.float32) for i in range(target_tokens.size(1)): target_token = target_tokens[:, i] max_score = torch.max(torch.eq(predicted_tokens, target_token.unsqueeze(dim=1)).float(), dim=1)[0] scores[:, i] = max_score loss = 1 - torch.mean(scores) return loss
这个错误通常是因为在计算损失函数时,某个张量没有设置梯度计算,但在反向传播时却尝试计算其梯度。为了解决这个问题,您可以将张量的`requires_grad`属性设置为`True`,以确保其梯度可以被计算。
请修改您的代码如下:
```python
class CustomLoss(nn.Module):
def __init__(self):
super(CustomLoss, self).__init__()
def forward(self, predicted_tokens, target_tokens):
# 设置允许梯度计算
predicted_tokens = predicted_tokens.requires_grad_()
target_tokens = target_tokens.requires_grad_()
scores = torch.zeros_like(target_tokens, dtype=torch.float32)
for i in range(target_tokens.size(1)):
target_token = target_tokens[:, i]
max_score = torch.max(torch.eq(predicted_tokens, target_token.unsqueeze(dim=1)).float(), dim=1)[0]
scores[:, i] = max_score
loss = 1 - torch.mean(scores)
return loss
```
在上述代码中,我们使用了`.requires_grad_()`方法来将`predicted_tokens`和`target_tokens`的`requires_grad`属性设置为`True`,以确保它们的梯度可以被计算。这样,在反向传播时就不会出现上述错误。
希望这个修改能够解决您遇到的问题!如果还有其他问题,请随时提问。
x = torch.arange(-8.0, 8.0, 0.1, requires_grad=True)
我可以帮助你解答这个问题。这段代码使用了 PyTorch 库中的 arange 函数,生成了一个张量 x,其中包含了从 -8.0 到 8.0 的所有数值,每个数值之间的差为 0.1。 requires_grad=True 表示这个张量是可导的,可以被用于梯度下降等机器学习算法中。需要注意的是,这个张量在生成时并没有进行任何运算,因此在现阶段它的梯度值为 None。
相关推荐
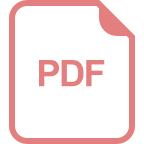
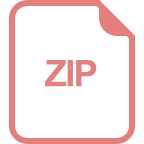
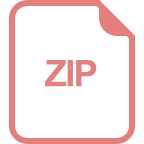










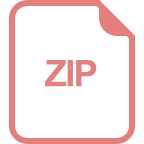
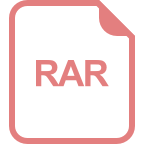