cor.test结果怎么可视化
时间: 2024-04-30 16:19:46 浏览: 12
要可视化 `cor.test()` 的结果,可以使用 `plot()` 函数。具体来说,可以使用以下步骤:
1. 使用 `cor.test()` 函数计算相关性的 p 值和 t 值。
2. 使用 `plot()` 函数绘制散点图,其中一个变量在 x 轴上,另一个变量在 y 轴上。
3. 使用 `abline()` 函数在散点图上添加一条直线,表示回归线。
4. 使用 `text()` 函数在图上添加文本标签,包括相关性系数和 p 值。
以下是一个示例代码:
```R
# 生成两个变量
x <- c(1, 2, 3, 4, 5)
y <- c(2, 4, 5, 7, 8)
# 计算相关性
cor.test(x, y)
# 绘制散点图
plot(x, y, main = "Scatterplot with regression line")
# 添加回归线
abline(lm(y ~ x))
# 添加文本标签
text(1, 8, paste("cor =", round(cor(x, y), 2)))
text(1, 7, paste("p =", round(cor.test(x, y)$p.value, 4)))
```
这将生成一个散点图,其中包括回归线和文本标签,显示相关性系数和 p 值。可以根据需要修改标签位置和样式。
相关问题
用R语言复现https://www.kaggle.com/lelexy/titanic-data-science-solutions/edit的数据处理,可视化与建模过程
好的,我会尽力为您解答。首先,您需要先下载 Titanic 数据集,可以在 Kaggle 上下载。然后,我们按照以下步骤进行数据处理,可视化与建模:
1. 导入所需的 R 包:
```
library(tidyverse)
library(survival)
library(rpart)
library(rpart.plot)
library(ranger)
```
2. 读取数据集,并将训练集和测试集合并:
```
train <- read.csv("train.csv", stringsAsFactors = F)
test <- read.csv("test.csv", stringsAsFactors = F)
full <- bind_rows(train, test)
```
3. 数据预处理:
```
# 用平均值填充 Age 中的缺失值
full$Age[is.na(full$Age)] <- mean(full$Age, na.rm = T)
# 用众数填充 Embarked 中的缺失值
full$Embarked[is.na(full$Embarked)] <- mode(full$Embarked, na.rm = T)
# 用中位数填充 Fare 中的缺失值
full$Fare[is.na(full$Fare)] <- median(full$Fare, na.rm = T)
# 将 Cabin 中的缺失值替换为 "Unknown"
full$Cabin[is.na(full$Cabin)] <- "Unknown"
# 创建新的变量 FamilySize 和 Alone
full$FamilySize <- full$SibSp + full$Parch + 1
full$Alone <- ifelse(full$FamilySize == 1, "Alone", "Not Alone")
# 将 Name 中的称谓提取出来
full$Title <- gsub('(.*, )|(\\..*)', '', full$Name)
full$Title[full$Title %in% c('Mlle', 'Ms')] <- 'Miss'
full$Title[full$Title == 'Mme'] <- 'Mrs'
full$Title[full$Title %in% c('Capt', 'Don', 'Major', 'Sir')] <- 'Sir'
full$Title[full$Title %in% c('Dona', 'Lady', 'the Countess', 'Jonkheer')] <- 'Lady'
```
4. 可视化:
```
# 柱状图:Survived 和 Sex
full %>%
filter(!is.na(Survived)) %>%
ggplot(aes(x = factor(Survived), fill = Sex)) +
geom_bar(position = "dodge") +
labs(x = "Survived", y = "Count", fill = "Sex")
# 箱线图:Survived 和 Age
full %>%
filter(!is.na(Survived)) %>%
ggplot(aes(x = factor(Survived), y = Age, fill = factor(Survived))) +
geom_boxplot() +
labs(x = "Survived", y = "Age", fill = "Survived")
# 散点图:Survived 和 Fare
full %>%
filter(!is.na(Survived)) %>%
ggplot(aes(x = factor(Survived), y = Fare, color = factor(Survived))) +
geom_jitter(alpha = 0.4) +
labs(x = "Survived", y = "Fare", color = "Survived")
# 热力图:特征之间的相关性
corr <- full %>%
select(-PassengerId, -Survived) %>%
cor()
corrplot::corrplot(corr, method = "color", type = "upper", order = "hclust")
```
5. 建模:
```
# 划分数据集
train <- full[1:891, ]
test <- full[892:1309, ]
# 决策树模型
tree <- rpart(Survived ~ Pclass + Sex + Age + SibSp + Parch + Fare + Embarked + Title, data = train, method = "class")
rpart.plot::rpart.plot(tree)
# 随机森林模型
rf <- ranger(Survived ~ Pclass + Sex + Age + SibSp + Parch + Fare + Embarked + Title, data = train, num.trees = 1000)
importance <- importance(rf)
varImpPlot(importance)
# 提交结果
test$Survived <- predict(rf, test)$predictions
submission <- test %>%
select(PassengerId, Survived) %>%
write.csv("submission.csv", row.names = F)
```
以上就是复现 Titanic 数据处理、可视化与建模过程的 R 代码,希望能对您有所帮助。
gam r语言结果分析
对于R语言的结果分析,一般可以按照以下步骤进行:
1. 数据预处理:将数据导入R语言中,并进行数据清洗、变量选择、变量转换等预处理工作。
2. 描述性统计分析:通过R语言中的summary()、describe()、cor()等函数,对数据进行描述性统计分析,得出数据的中心趋势、离散程度、相关性等信息。
3. 可视化分析:通过R语言中的ggplot2、plot()等函数,绘制直方图、散点图、线图等图形,对数据进行可视化分析,直观地了解数据的分布、趋势等特征。
4. 假设检验:通过R语言中的t.test()、anova()等函数,对数据进行假设检验,判断不同组之间是否存在显著差异。
5. 模型建立:通过R语言中的lm()、glm()、randomForest()等函数,建立回归模型、分类模型、机器学习模型等,对数据进行预测和分析。
以上是R语言结果分析的一般步骤,具体分析方法和函数根据具体数据和问题而定。
相关推荐
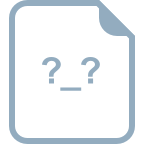
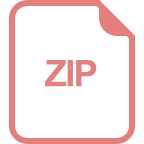












