np.linalg.lstsq和solve区别
时间: 2023-10-24 21:09:22 浏览: 79
`numpy.linalg.lstsq` 和 `numpy.linalg.solve` 都是用于求解线性方程组的函数,但它们的使用场景略有不同。
`numpy.linalg.solve` 用于解决形如 Ax = b 的线性方程组,其中 A 是一个 n×n 的矩阵,x 和 b 都是长度为 n 的向量。solve 函数会返回一个长度为 n 的向量,表示方程组的解。
`numpy.linalg.lstsq` 则用于求解形如 Ax = b 的最小二乘问题。当 A 不是方阵时,无法使用 solve 直接求解。此时,我们可以使用 lstsq 函数求出 Ax 与 b 之间的最小二乘解 x,使得两者之间的误差最小。lstsq 函数返回一个元组,其中第一个元素表示最小二乘解 x,第二个元素表示 Ax 与 b 之间的残差平方和。
因此,当需要求解线性方程组时,我们应该使用 solve 函数;当需要求解最小二乘问题时,我们应该使用 lstsq 函数。
相关问题
用C++实现np.linalg.lstsq
np.linalg.lstsq是numpy库中的一个函数,用于求解最小二乘问题。如果要在C++中实现类似的功能,可以使用Eigen库中的LeastSquares类。
以下是一个简单的C++代码示例,演示如何使用LeastSquares类来实现最小二乘问题的求解:
```cpp
#include <Eigen/Dense>
using namespace Eigen;
int main()
{
MatrixXd A(3, 2);
VectorXd b(3);
// 构造矩阵A和向量b
A << 1, 2, 3, 4, 5, 6;
b << 1, 2, 3;
// 构造最小二乘对象
LeastSquares<MatrixXd> ls(A);
ls.solve(b);
// 输出结果
std::cout << "x = " << ls.x() << std::endl;
std::cout << "residual = " << ls.residual() << std::endl;
return 0;
}
```
在这个示例中,我们首先构造了一个3x2的矩阵A和一个长度为3的向量b。然后,我们使用LeastSquares类构造了一个最小二乘对象,并调用solve()方法来求解最小二乘问题。最后,我们输出了解向量x和残差(residual)的值。
需要注意的是,这个示例中使用了Eigen库中的MatrixXd和VectorXd类来表示矩阵和向量,如果要使用其他类型的矩阵和向量,需要根据实际情况进行调整。另外,LeastSquares类还提供了一些其他的方法,如rank()、nullspace()等,可以用来进行矩阵分解和求解相关的问题。
a=np.mat('2,3,1;1,-2,4;3,8,-2;4,-1,9') b=np.mat('4;-5;13;-6') c=np.linalg.solve(a,b) c
根据您提供的代码,您想要求解线性方程组a*x = b的解。在这种情况下,由于矩阵a不是一个方阵(即不是一个正方形矩阵),无法使用`np.linalg.solve`函数直接求解。
解决此问题的一种方法是使用最小二乘法来近似求解。您可以使用`np.linalg.lstsq`函数来实现。以下是修改后的代码:
```python
import numpy as np
a = np.mat('2,3,1;1,-2,4;3,8,-2;4,-1,9')
b = np.mat('4;-5;13;-6')
c, residuals, rank, singular_values = np.linalg.lstsq(a, b, rcond=None)
print("线性方程组的近似解为:")
print(c)
```
运行这段代码将会打印出线性方程组的近似解。
请注意,`np.linalg.lstsq`函数返回一个元组,其中包含近似解c、残差residuals、矩阵a的秩rank和奇异值singular_values。
如果您有任何其他问题,请随时提问。
相关推荐
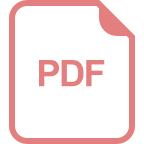
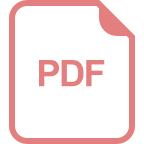












