pointnet++点云识别算法代码
时间: 2023-08-06 16:01:42 浏览: 49
PointNet++是一种用于点云识别的神经网络算法,它包含基于点云数据的多个层次,可以适应不同大小和分辨率的点云。以下是PointNet++的一个简单实现示例。
```
import tensorflow as tf
from tensorflow.keras import layers
def pointnet2_model(num_classes, input_shape):
inputs = layers.Input(shape=input_shape)
# Pointnet++ MSG
x = layers.Conv1D(64, 1, activation='relu')(inputs)
x = layers.BatchNormalization()(x)
x = layers.Conv1D(64, 1, activation='relu')(x)
x = layers.BatchNormalization()(x)
# Set Abstraction Block 1
x0 = x
x = layers.Conv1D(64, 1, activation='relu')(x)
x = layers.BatchNormalization()(x)
x = layers.Conv1D(64, 1, activation='relu')(x)
x = layers.BatchNormalization()(x)
x1 = layers.Conv1D(64, 1)(x)
x = layers.Conv1D(128, 1, activation='relu')(x)
x = layers.BatchNormalization()(x)
x = layers.Conv1D(128, 1, activation='relu')(x)
x = layers.BatchNormalization()(x)
x2 = layers.Conv1D(128, 1)(x)
x = layers.Conv1D(256, 1, activation='relu')(x)
x = layers.BatchNormalization()(x)
x = layers.Conv1D(256, 1, activation='relu')(x)
x = layers.BatchNormalization()(x)
x3 = layers.Conv1D(256, 1)(x)
# Set Abstraction Block 2
x = layers.Concatenate()([x1, x2, x3])
x = layers.Conv1D(256, 1, activation='relu')(x)
x = layers.BatchNormalization()(x)
x = layers.Conv1D(512, 1, activation='relu')(x)
x = layers.BatchNormalization()(x)
x = layers.Conv1D(1024, 1, activation='relu')(x)
x = layers.BatchNormalization()(x)
x4 = layers.Conv1D(1024, 1)(x)
# Global Feature Extraction
x = layers.MaxPooling1D(pool_size=input_shape[0])(x4)
x = layers.Dense(512, activation='relu')(x)
x = layers.BatchNormalization()(x)
x = layers.Dense(256, activation='relu')(x)
x = layers.BatchNormalization()(x)
# Classification
x = layers.Flatten()(x)
outputs = layers.Dense(num_classes, activation='softmax')(x)
# Model Definition
model = tf.keras.Model(inputs=inputs, outputs=outputs, name='pointnet2')
return model
```
在这个示例中,我们定义了一个名为`pointnet2_model`的函数,该函数接受两个参数:`num_classes`是输出类别的数量,`input_shape`是输入点云数据的形状。函数返回一个Keras模型对象。
该模型包含两个Set Abstraction Block和一个Global Feature Extraction层,以及一个分类器层。每个Set Abstraction Block包含一个MSG层和三个PointNet++层,用于对点云数据进行多个分辨率的特征提取。Global Feature Extraction层将所有点的特征向量池化为一个全局特征向量,用于分类器。
在训练过程中,我们可以使用类似于其他神经网络模型的训练方法,如随机梯度下降(SGD)或自适应矩估计(Adam)。
```
model = pointnet2_model(num_classes=10, input_shape=(1024, 3))
model.compile(optimizer='adam', loss='categorical_crossentropy', metrics=['accuracy'])
model.fit(x_train, y_train, batch_size=32, epochs=100, validation_data=(x_val, y_val))
```
在这个示例中,我们使用了Adam优化器和交叉熵损失函数进行训练。我们还将训练数据分为批次,以便更有效地训练模型,并记录模型的准确性。
相关推荐
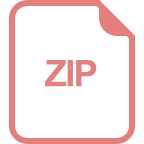
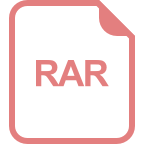














