用python基于神经网络的THUCNews数据集文本分类
时间: 2024-06-09 13:08:27 浏览: 121
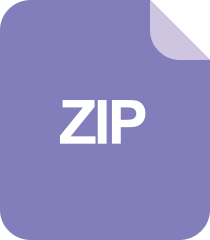
NLP实战之基于tensorflow+字符级CNN进行THUCNews文本分类python
好的,我可以给你提供一个基于神经网络的THUCNews数据集文本分类的Python实现。
首先,我们需要下载THUCNews数据集。可以在清华大学开源软件镜像站上下载,链接为:https://mirror.tuna.tsinghua.edu.cn/thulac/data/THUCNews.zip。
接下来,我们需要对数据进行预处理。我们可以使用Python中的jieba库进行中文分词,然后将分词结果转化成数字形式。代码如下所示:
```python
import jieba
import os
import numpy as np
# 加载停用词
stopwords = []
with open('stopwords.txt', 'r', encoding='utf-8') as f:
for line in f:
stopwords.append(line.strip())
# 对文本进行分词和数字化处理
def preprocess_text(text, word_to_id, max_length):
words = jieba.cut(text)
words = [word for word in words if word not in stopwords]
words = [word_to_id[word] if word in word_to_id else 0 for word in words]
if len(words) < max_length:
words += [0] * (max_length - len(words))
else:
words = words[:max_length]
return np.array(words)
```
接下来,我们需要将文本数据转化成数字形式。我们可以先将所有文本中的单词统计出来,然后根据单词出现次数进行排序,将出现频率最高的前N个单词作为我们的词汇表。代码如下所示:
```python
# 构建词汇表
def build_vocab(data_path, vocab_path, vocab_size):
word_to_count = {}
with open(data_path, 'r', encoding='utf-8') as f:
for line in f:
line = line.strip().split('\t')
if len(line) != 2:
continue
words = jieba.cut(line[1])
for word in words:
if word not in word_to_count:
word_to_count[word] = 0
word_to_count[word] += 1
sorted_words = sorted(word_to_count.items(), key=lambda x: x[1], reverse=True)
# 取出现频率最高的前vocab_size个单词
vocab = ['<PAD>', '<UNK>'] + [x[0] for x in sorted_words[:vocab_size - 2]]
with open(vocab_path, 'w', encoding='utf-8') as f:
f.write('\n'.join(vocab))
```
接下来,我们可以将所有文本数据转化成数字形式。代码如下所示:
```python
# 将数据转化成数字形式
def convert_data_to_id(data_path, vocab_path, max_length):
with open(vocab_path, 'r', encoding='utf-8') as f:
vocab = [line.strip() for line in f]
word_to_id = {word: i for i, word in enumerate(vocab)}
labels = []
texts = []
with open(data_path, 'r', encoding='utf-8') as f:
for line in f:
line = line.strip().split('\t')
if len(line) != 2:
continue
label = int(line[0])
text = preprocess_text(line[1], word_to_id, max_length)
labels.append(label)
texts.append(text)
return np.array(labels), np.array(texts)
```
接下来,我们可以定义神经网络模型。这里我们使用一个简单的卷积神经网络,代码如下所示:
```python
import tensorflow as tf
# 定义卷积神经网络模型
def cnn_model(inputs, num_classes, vocab_size, embedding_size, filter_sizes, num_filters):
# Embedding Layer
with tf.name_scope("embedding"):
W = tf.Variable(tf.random_uniform([vocab_size, embedding_size], -1.0, 1.0), name="W")
embedded_chars = tf.nn.embedding_lookup(W, inputs)
embedded_chars_expanded = tf.expand_dims(embedded_chars, -1)
# Convolution and Max Pooling Layers
pooled_outputs = []
for i, filter_size in enumerate(filter_sizes):
with tf.name_scope("conv-maxpool-%s" % filter_size):
# Convolution Layer
filter_shape = [filter_size, embedding_size, 1, num_filters]
W = tf.Variable(tf.truncated_normal(filter_shape, stddev=0.1), name="W")
b = tf.Variable(tf.constant(0.1, shape=[num_filters]), name="b")
conv = tf.nn.conv2d(embedded_chars_expanded, W, strides=[1, 1, 1, 1], padding="VALID", name="conv")
# Activation Function
h = tf.nn.relu(tf.nn.bias_add(conv, b), name="relu")
# Max Pooling Layer
pooled = tf.nn.max_pool(h, ksize=[1, inputs.shape[1] - filter_size + 1, 1, 1], strides=[1, 1, 1, 1], padding="VALID", name="pool")
pooled_outputs.append(pooled)
# Combine All Pooled Features
num_filters_total = num_filters * len(filter_sizes)
h_pool = tf.concat(pooled_outputs, 3)
h_pool_flat = tf.reshape(h_pool, [-1, num_filters_total])
# Dropout Layer
with tf.name_scope("dropout"):
keep_prob = tf.placeholder(tf.float32, name="keep_prob")
h_drop = tf.nn.dropout(h_pool_flat, keep_prob)
# Output Layer
with tf.name_scope("output"):
W = tf.Variable(tf.truncated_normal([num_filters_total, num_classes], stddev=0.1), name="W")
b = tf.Variable(tf.constant(0.1, shape=[num_classes]), name="b")
scores = tf.nn.xw_plus_b(h_drop, W, b, name="scores")
return scores, keep_prob
```
接下来,我们可以定义训练函数。代码如下所示:
```python
# 训练函数
def train(data_path, vocab_path, model_path, num_classes, vocab_size, max_length, embedding_size, filter_sizes, num_filters, batch_size, num_epochs, learning_rate):
# 加载数据
labels, texts = convert_data_to_id(data_path, vocab_path, max_length)
# 划分训练集和测试集
num_samples = len(labels)
indices = np.random.permutation(num_samples)
train_indices = indices[:int(num_samples * 0.8)]
test_indices = indices[int(num_samples * 0.8):]
train_labels = labels[train_indices]
test_labels = labels[test_indices]
train_texts = texts[train_indices]
test_texts = texts[test_indices]
# 定义模型
inputs = tf.placeholder(tf.int32, [None, max_length], name="inputs")
labels = tf.placeholder(tf.int32, [None], name="labels")
logits, keep_prob = cnn_model(inputs, num_classes, vocab_size, embedding_size, filter_sizes, num_filters)
# 定义损失函数和优化器
with tf.name_scope("loss"):
loss = tf.reduce_mean(tf.nn.sparse_softmax_cross_entropy_with_logits(logits=logits, labels=labels))
with tf.name_scope("optimizer"):
optimizer = tf.train.AdamOptimizer(learning_rate)
grads_and_vars = optimizer.compute_gradients(loss)
train_op = optimizer.apply_gradients(grads_and_vars)
# 定义评估指标
with tf.name_scope("accuracy"):
correct_predictions = tf.equal(tf.argmax(logits, 1), tf.cast(labels, tf.int64))
accuracy = tf.reduce_mean(tf.cast(correct_predictions, tf.float32))
# 训练模型
with tf.Session() as sess:
sess.run(tf.global_variables_initializer())
for epoch in range(num_epochs):
train_loss = 0.0
train_acc = 0.0
num_batches = int(len(train_labels) / batch_size)
for i in range(num_batches):
batch_labels = train_labels[i * batch_size:(i + 1) * batch_size]
batch_texts = train_texts[i * batch_size:(i + 1) * batch_size]
_, batch_loss, batch_acc = sess.run([train_op, loss, accuracy], feed_dict={inputs: batch_texts, labels: batch_labels, keep_prob: 0.5})
train_loss += batch_loss
train_acc += batch_acc
train_loss /= num_batches
train_acc /= num_batches
test_loss, test_acc = sess.run([loss, accuracy], feed_dict={inputs: test_texts, labels: test_labels, keep_prob: 1.0})
print("Epoch %d: train_loss=%.4f, train_acc=%.4f, test_loss=%.4f, test_acc=%.4f" % (epoch + 1, train_loss, train_acc, test_loss, test_acc))
# 保存模型
saver = tf.train.Saver()
saver.save(sess, model_path)
```
最后,我们可以调用训练函数进行训练。代码如下所示:
```python
data_path = 'THUCNews/THUCNews_train.txt'
vocab_path = 'vocab.txt'
model_path = 'model.ckpt'
num_classes = 14
vocab_size = 50000
max_length = 200
embedding_size = 128
filter_sizes = [2, 3, 4]
num_filters = 128
batch_size = 64
num_epochs = 10
learning_rate = 1e-3
# 构建词汇表
build_vocab(data_path, vocab_path, vocab_size)
# 训练模型
train(data_path, vocab_path, model_path, num_classes, vocab_size, max_length, embedding_size, filter_sizes, num_filters, batch_size, num_epochs, learning_rate)
```
这样,我们就完成了一个基于神经网络的THUCNews数据集文本分类的Python实现。
阅读全文
相关推荐
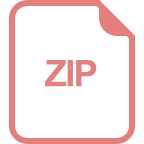
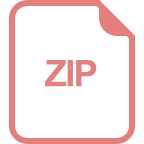





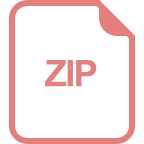
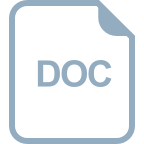


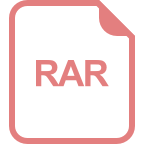
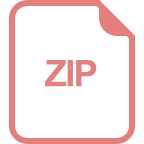
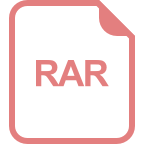
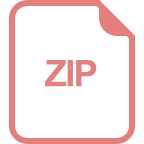
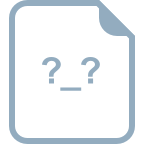
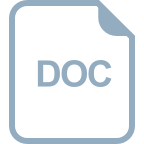
