python调用gpu进行计算
时间: 2024-01-25 18:04:14 浏览: 96
要使用GPU进行计算,需要安装相应的GPU驱动和CUDA库。一般来说,可以使用NVIDIA官方提供的CUDA Toolkit来安装驱动和CUDA库。安装完成后,可以使用Python中的一些深度学习框架(如PyTorch、TensorFlow等)来调用GPU进行计算。
以下是一个使用PyTorch调用GPU的示例代码:
```python
import torch
# 检查是否有可用的GPU
if torch.cuda.is_available():
# 将模型和数据放到GPU上
device = torch.device("cuda")
model.to(device)
data.to(device)
# 在GPU上进行计算
output = model(data)
else:
# 在CPU上进行计算
output = model(data)
```
在这个示例中,首先检查是否有可用的GPU。如果有,将模型和数据放到GPU上并使用`torch.device`指定设备,然后在GPU上进行计算。如果没有可用的GPU,则在CPU上进行计算。
相关问题
python调用GPU
要在Python中使用GPU,需要使用一个支持GPU加速的深度学习框架,例如TensorFlow、PyTorch等。这些框架会自动检测并利用可用的GPU来加速计算。
以下是使用TensorFlow在Python中调用GPU的示例代码:
```python
import tensorflow as tf
# 检查是否有可用的GPU
if tf.test.gpu_device_name():
print('Default GPU Device: {}'.format(tf.test.gpu_device_name()))
else:
print("Please install GPU version of TF")
# 创建一个TensorFlow会话
with tf.Session() as sess:
# 构建一个简单的计算图
a = tf.constant(10)
b = tf.constant(20)
c = tf.add(a, b)
# 运行计算图并打印结果
print(sess.run(c))
```
该代码首先检查是否有可用的GPU,然后创建一个TensorFlow会话并构建一个简单的计算图。在运行计算图时,TensorFlow会自动检测并利用可用的GPU来加速计算。
Python 调用GPU
在Python中使用GPU进行计算通常涉及到使用特定的库,如NumPy、Pandas(它们默认是CPU加速的),或是专为GPU编程设计的库如TensorFlow、PyTorch等。如果你想要利用GPU,你需要确保你的系统已经安装了支持GPU的版本,并且正确配置了环境。
首先,你需要安装CUDA和cuDNN(对NVIDIA GPU的支持库):
```bash
# 对于NVIDIA GPU
sudo apt-get install nvidia-cuda-toolkit # Ubuntu
# 或者 yum install nvidia-cuda # CentOS/RHEL
```
然后安装Python GPU库,例如:
- TensorFlow: `pip install tensorflow-gpu`
- PyTorch: `pip install torch torchvision torchaudio -f https://download.pytorch.org/whl/cpu/torch_stable.html`
为了使用GPU,你需要在代码中明确指定:
```python
import tensorflow as tf
physical_devices = tf.config.list_physical_devices('GPU')
if physical_devices:
tf.config.experimental.set_memory_growth(physical_devices, True)
print("Using GPU:", tf.test.is_gpu_available())
# 或者 PyTorch
import torch
device = torch.device("cuda" if torch.cuda.is_available() else "cpu")
print("Using GPU:", device.type == 'cuda')
```
阅读全文
相关推荐
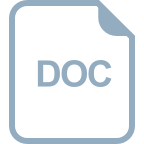
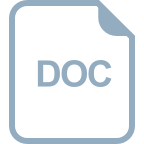
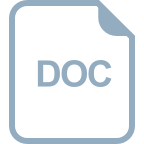


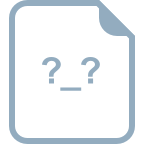










