给你更多的上下文信息: for t in range(input.size(0)): x = input[t] i = torch.sigmoid(torch.matmul(x, self.W_i_forward) + torch.matmul(h_forward_new[-1], self.U_i_forward) + self.b_i_forward) f = torch.sigmoid(torch.matmul(x, self.W_f_forward) + torch.matmul(h_forward_new[-1], self.U_f_forward) + self.b_f_forward) c_tilde = torch.tanh(torch.matmul(x, self.W_c_forward) + torch.matmul(h_forward_new[-1], self.U_c_forward) + self.b_c_forward) c_forward_new[-1] = f * c_forward_new[-1] + i * c_tilde o = torch.matmul(x, self.W_o_forward) + torch.matmul(h_forward_new[-1], self.U_o_forward) + self.b_o_forward o = torch.sigmoid(o) h_forward_new[-1] = o * torch.tanh(c_forward_new[-1]) outputs_forward.append(h_forward_new[-1])
时间: 2024-03-03 20:51:23 浏览: 83
根据你提供的代码,我认为报错是因为在进行`c_forward_new[-1] = f * c_forward_new[-1] + i * c_tilde`操作时,`c_forward_new[-1]`的大小和`f * c_forward_new[-1] + i * c_tilde`的大小不一致,导致无法进行赋值操作。具体来说,可能是`c_forward_new[-1]`的大小为`[16]`,而`f * c_forward_new[-1] + i * c_tilde`的大小为`[64, 16]`。
解决这个问题的方法是,先将`c_forward_new[-1]`的大小扩展为`[64, 16]`,然后再进行赋值操作。具体代码如下:
```
c_forward_new[-1] = c_forward_new[-1].expand_as(f * c_forward_new[-1]) # 将c_forward_new[-1]扩展为与f * c_forward_new[-1]相同的大小
c_forward_new[-1] = f * c_forward_new[-1] + i * c_tilde # 进行赋值操作
```
这样就可以解决报错问题。
相关问题
请在这个DeepCFD的网络添加attention机制,并给出示例代码:import paddle import paddle.nn as nn class Attention(nn.Layer): def __init__(self, input_size, hidden_size): super(Attention, self).__init__() self.input_size = input_size self.hidden_size = hidden_size self.attn = nn.Linear(self.input_size + self.hidden_size, 1) self.softmax = nn.Softmax(axis=1) def forward(self, input, hidden): max_len = input.shape[0] attn_energies = paddle.zeros([max_len, 1]) for i in range(max_len): attn_energies[i] = self.score(input[i], hidden) attn_weights = self.softmax(attn_energies) context = paddle.sum(attn_weights * input, axis=0) return context.unsqueeze(0) def score(self, input, hidden): energy = self.attn(paddle.concat([input, hidden], axis=1)) return energy class DeepCFD(nn.Layer): def __init__(self, input_size, hidden_size, output_size): super(DeepCFD, self).__init__() self.input_size = input_size self.hidden_size = hidden_size self.output_size = output_size self.lstm = nn.LSTM(input_size, hidden_size, num_layers=2, batch_first=True) self.attention = Attention(input_size, hidden_size) self.fc = nn.Linear(hidden_size, output_size) def forward(self, input): output, (hidden, cell) = self.lstm(input) context = self.attention(output, hidden[-1]) output = self.fc(context) return output
好的,下面是添加了Attention机制的DeepCFD网络的示例代码:
import paddle
import paddle.nn as nn
class Attention(nn.Layer):
def __init__(self, input_size, hidden_size):
super(Attention, self).__init__()
self.input_size = input_size
self.hidden_size = hidden_size
self.attn = nn.Linear(self.input_size + self.hidden_size, 1)
self.softmax = nn.Softmax(axis=1)
def forward(self, input, hidden):
max_len = input.shape[1]
attn_energies = paddle.zeros([input.shape[0], max_len, 1])
for i in range(max_len):
attn_energies[:, i] = self.score(input[:, i, :], hidden)
attn_weights = self.softmax(attn_energies)
context = paddle.sum(attn_weights * input, axis=1)
return context
def score(self, input, hidden):
energy = self.attn(paddle.concat([input, hidden], axis=1))
return energy
class DeepCFD(nn.Layer):
def __init__(self, input_size, hidden_size, output_size):
super(DeepCFD, self).__init__()
self.input_size = input_size
self.hidden_size = hidden_size
self.output_size = output_size
self.lstm = nn.LSTM(input_size, hidden_size, num_layers=2, batch_first=True)
self.attention = Attention(input_size, hidden_size)
self.fc = nn.Linear(hidden_size, output_size)
def forward(self, input):
output, (hidden, cell) = self.lstm(input)
context = self.attention(output, hidden[-1])
output = self.fc(context)
return output
在这个示例代码中,我们将Attention机制应用到了LSTM的输出上。在Attention中,我们计算了每个时间步的注意力能量,然后使用softmax函数计算注意力权重。然后,我们将这些权重与LSTM输出相乘并求和,得到上下文向量作为Attention机制的输出。
在DeepCFD中,我们使用了两层LSTM,然后将LSTM输出和最后一个时刻的隐藏状态作为Attention机制的输入。最后,我们将Attention机制的输出传递到一个全连接层中,得到最终的输出。
import numpy as np import math import tensorflow as tf import matplotlib.pyplot as plt # 生成数据 dataset = [] for data in np.arange(0, 3, .01): data = math.sin(data * math.pi) dataset.append(data) dataset = np.array(dataset) dataset = dataset.astype('float32') max_value = np.max(dataset) min_value = np.min(dataset) scalar = max_value - min_value dataset = list(map(lambda x: x / scalar, dataset)) # 创建数据集 look_back = 5 trainX, trainY = [], [] for i in range(len(dataset) - look_back): trainX.append(dataset[i:i+look_back]) trainY.append(dataset[i+look_back]) trainX = np.array(trainX) trainY = np.array(trainY) # 转换为张量 trainX = tf.convert_to_tensor(trainX) trainY = tf.convert_to_tensor(trainY) # 定义模型 model = tf.keras.Sequential([ tf.keras.layers.SimpleRNN(32, input_shape=(look_back, 1)), tf.keras.layers.Dense(1) ]) # 编译模型 model.compile(optimizer=tf.keras.optimizers.Adam(), loss=tf.keras.losses.MeanSquaredError()) # 训练模型 history = model.fit(trainX, trainY, epochs=100, batch_size=16, verbose=2) # 预测结果 predicted = [] for i in range(len(dataset) - look_back): x = np.array(dataset[i:i+look_back]) x = np.reshape(x, (1, look_back, 1)) y = model.predict(x) predicted.append(y[0][0]) # 反归一化 predicted = np.array(predicted) predicted = predicted * scalar # 绘图 plt.plot(dataset[look_back:], label='True Data') plt.plot(predicted, label='Predicted Data') plt.legend() plt.show()生成的真实值只有一半大小
根据您提供的代码,我猜测您可能是在对预测结果进行反归一化时出现了问题。在代码中,您将预测结果乘以了一个名为scalar的标量值,但是scalar的计算方式并没有给出。如果scalar的计算方式不正确,可能导致反归一化后的预测结果不准确。
另外,您在代码中没有给出任何关于预测结果大小的具体描述。如果您说生成的真实值只有一半大小,可能需要更多上下文信息才能理解您的意思。
阅读全文
相关推荐
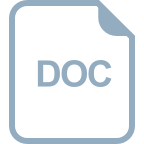
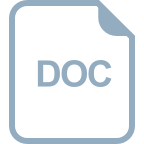
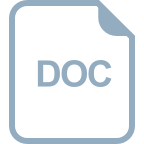














