with pd.option_context('max_colwidth', 200): display(twitter_archive_clean[twitter_archive_clean['text'].str.contains(r"(\d+\.\d*\/\d+)")] [['tweet_id', 'text', 'rating_numerator', 'rating_denominator']])
时间: 2023-06-12 11:03:01 浏览: 115
这段代码是用来显示包含特定数字格式的推特文本信息的数据,其中包括推特ID、文本、分子评分和分母评分。通常这种数字格式是“数字.数字/数字”的形式,其中分子评分是小数,分母评分是整数。这段代码使用了 Pandas 库中的 option_context() 方法来设置显示文本列的最大宽度为 200,以便更好地查看文本信息。如果你运行这段代码,将会得到符合条件的推特数据的表格展示。
相关问题
with pd.option_context('max_colwidth', 200)
这是一个 pandas 库的函数,用于设置打印 DataFrame 时每列的最大宽度。具体来说,使用该函数可以临时地将 DataFrame 每列的最大宽度设置为指定的值,以便更好地展示数据。例如:
```
import pandas as pd
df = pd.DataFrame({
'name': ['Alice', 'Bob', 'Charlie'],
'description': ['A person who likes reading books and watching movies',
'A person who enjoys playing video games',
'A person who loves hiking and traveling']
})
with pd.option_context('max_colwidth', 50):
print(df)
```
输出结果为:
```
name description
0 Alice A person who likes reading books and watchin...
1 Bob A person who enjoys playing video...
2 Charlie A person who loves hiking and traveling
```
可以看到,`max_colwidth` 参数被设置为 50,因此 `description` 列的最大宽度被限制在 50 个字符以内。使用 `with` 语句可以临时地设置该参数,不影响其他代码。
import numpy as np import pandas as pd from sklearn.cluster import KMeans from sklearn.preprocessing import StandardScaler from scipy.spatial.distance import cdist import matplotlib.pyplot as plt from pandas import DataFrame from sklearn.decomposition import PCA plt.rcParams['font.sans-serif']=['SimHei'] #用来正常显示中文标签 plt.rcParams['axes.unicode_minus']=False #用来正常显示负号 pd.set_option('display.max_rows', None)#显示全部行 pd.set_option('display.max_columns', None)#显示全部列 np.set_printoptions(threshold=np.inf) pd.set_option('display.max_columns', 9000) pd.set_option('display.width', 9000) pd.set_option('display.max_colwidth', 9000) df = pd.read_csv(r'附件1.csv',encoding='gbk') X = np.array(df.iloc[:, 1:]) X=X[0:,1:] k=93 kmeans_model = KMeans(n_clusters=k, random_state=123) fit_kmeans = kmeans_model.fit(X) # 模型训练 #查看聚类结果 kmeans_cc = kmeans_model.cluster_centers_ # 聚类中心 print('各类聚类中心为:\n', kmeans_cc) kmeans_labels = kmeans_model.labels_ # 样本的类别标签 print('各样本的类别标签为:\n', kmeans_labels) r1 = pd.Series(kmeans_model.labels_).value_counts() # 统计不同类别样本的数目 print('最终每个类别的数目为:\n', r1) # 输出聚类分群的结果 # cluster_center = pd.DataFrame(kmeans_model.cluster_centers_, # columns=[ str(x) for x in range(1,94)]) # 将聚类中心放在数据框中 # cluster_center.index = pd.DataFrame(kmeans_model.labels_). \ # drop_duplicates().iloc[:, 0] # 将样本类别作为数据框索引 # print(cluster_center)代码解释
这段代码主要是使用KMeans算法对一个数据集进行聚类分析,并输出聚类结果。下面是代码的解释:
1. 导入所需的库
```python
import numpy as np
import pandas as pd
from sklearn.cluster import KMeans
from sklearn.preprocessing import StandardScaler
from scipy.spatial.distance import cdist
import matplotlib.pyplot as plt
from pandas import DataFrame
from sklearn.decomposition import PCA
```
2. 读取数据集
```python
df = pd.read_csv(r'附件1.csv',encoding='gbk')
X = np.array(df.iloc[:, 1:])
X=X[0:,1:]
```
3. 使用KMeans算法进行聚类分析
```python
k=93
kmeans_model = KMeans(n_clusters=k, random_state=123)
fit_kmeans = kmeans_model.fit(X)
```
4. 输出聚类结果
```python
kmeans_cc = kmeans_model.cluster_centers_
print('各类聚类中心为:\n', kmeans_cc)
kmeans_labels = kmeans_model.labels_
print('各样本的类别标签为:\n', kmeans_labels)
r1 = pd.Series(kmeans_model.labels_).value_counts()
print('最终每个类别的数目为:\n', r1)
```
上述代码中,kmeans_cc表示各个类别的聚类中心,kmeans_labels表示每个样本所属的类别,r1表示每个类别的样本数目。
5. 将聚类中心放在数据框中
```python
# cluster_center = pd.DataFrame(kmeans_model.cluster_centers_,
# columns=[ str(x) for x in range(1,94)])
# 将聚类中心放在数据框中
# cluster_center.index = pd.DataFrame(kmeans_model.labels_). \
# drop_duplicates().iloc[:, 0]
# 将样本类别作为数据框索引
# print(cluster_center)
```
这段代码是将聚类中心放在数据框中,并以样本类别作为索引。但是,这段代码被注释掉了,因此不会被执行。
阅读全文
相关推荐
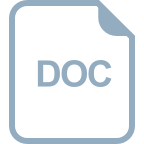
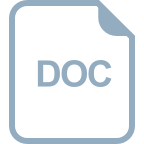
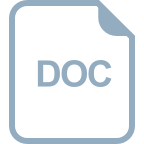









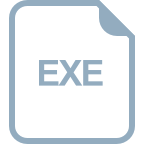



