# Mapping to [c], cmean self.fc1_m = nn.Linear(512, 256) self.fc2_m = nn.Linear(256, 128) self.fc3_m = nn.Linear(128, zdim) self.fc_bn1_m = nn.BatchNorm1d(256) self.fc_bn2_m = nn.BatchNorm1d(128)是什么意思
时间: 2024-04-09 18:30:43 浏览: 143
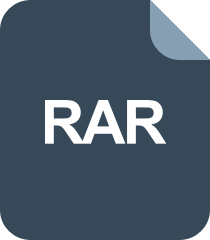
PUSCH_mapping.rar_LTE PUSCH_M_PUSCH_PUSCH 资源映射_lte mapping_resou

这段代码是一个神经网络模型的定义,用于进行特征映射(Mapping)和计算均值(Mean)。具体解释如下:
- `self.fc1_m = nn.Linear(512, 256)`:这一行代码定义了一个线性层(Linear Layer),将输入维度为512的特征映射到维度为256的特征空间。
- `self.fc2_m = nn.Linear(256, 128)`:这一行代码定义了另一个线性层,将输入维度为256的特征映射到维度为128的特征空间。
- `self.fc3_m = nn.Linear(128, zdim)`:这一行代码定义了第三个线性层,将输入维度为128的特征映射到维度为zdim的特征空间。其中,zdim是一个变量,表示输出特征的维度。
- `self.fc_bn1_m = nn.BatchNorm1d(256)` 和 `self.fc_bn2_m = nn.BatchNorm1d(128)`:这两行代码定义了两个批标准化层(Batch Normalization Layer),用于对输入进行标准化处理,加速训练过程和提高模型性能。
总之,这段代码定义了一个神经网络模型,通过多个线性层和批标准化层对输入特征进行映射和处理,最终得到一个具有zdim维度的特征表示。这样的操作常用于深度学习中的特征提取和降维任务。
阅读全文
相关推荐
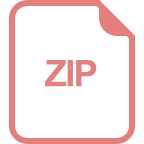
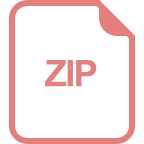

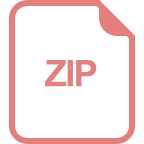
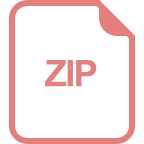
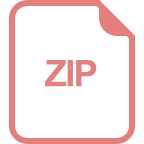
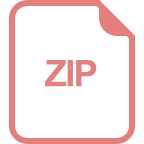