dataframe GBDT模型怎么测试数据然后预测数据
时间: 2023-12-03 19:44:53 浏览: 32
在使用GBDT模型进行测试数据和预测数据时,可以按照以下步骤进行操作:
1. 将测试数据和预测数据分别存储在DataFrame中。
2. 从sklearn库中导入GradientBoostingClassifier或GradientBoostingRegressor,根据任务类型选择合适的模型。
3. 使用fit方法对训练数据进行拟合训练,建立GBDT模型。
4. 使用predict方法对测试数据进行预测,得到预测结果。
5. 对预测结果进行评估,可以使用sklearn库中的评估函数进行评估,如accuracy_score、precision_score、recall_score等。
下面是一个示例代码:
```python
import pandas as pd
from sklearn.ensemble import GradientBoostingClassifier
# 读取训练数据和测试数据
train_data = pd.read_csv('train.csv')
test_data = pd.read_csv('test.csv')
# 将特征和标签分别提取出来
X_train = train_data.drop('label', axis=1)
y_train = train_data['label']
X_test = test_data.drop('label', axis=1)
y_test = test_data['label']
# 建立GBDT模型
gbdt = GradientBoostingClassifier(n_estimators=100, max_depth=3, random_state=0)
gbdt.fit(X_train, y_train)
# 对测试数据进行预测
y_pred = gbdt.predict(X_test)
# 对预测结果进行评估
from sklearn.metrics import accuracy_score
acc = accuracy_score(y_test, y_pred)
print('Accuracy:', acc)
```
在上面的代码中,我们首先从csv文件中读取训练数据和测试数据,然后将特征和标签分别提取出来。接着,我们使用GradientBoostingClassifier建立GBDT模型,并对训练数据进行拟合训练。最后,我们使用predict方法对测试数据进行预测,并使用accuracy_score函数对预测结果进行评估。
相关推荐
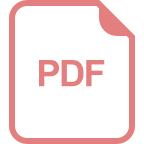
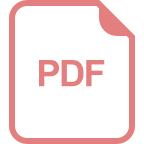
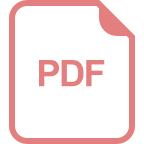












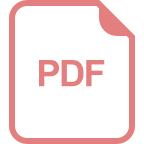
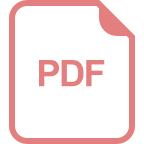
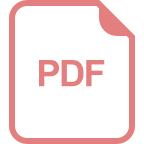