Python代码实现使用梯度提升树GBDT实现Boston房价预测
时间: 2023-11-29 18:46:08 浏览: 106
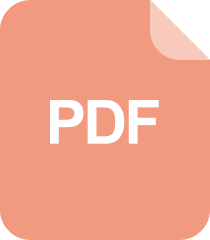
python数据挖掘预测Boston房价
以下是使用Python实现使用梯度提升树GBDT实现Boston房价预测的代码:
```python
# 导入必要的库
import pandas as pd
from sklearn.datasets import load_boston
from sklearn.model_selection import train_test_split
from sklearn.ensemble import GradientBoostingRegressor
from sklearn.metrics import mean_squared_error
# 导入数据集
boston = load_boston()
# 将数据集转换为DataFrame格式
df = pd.DataFrame(boston.data, columns=boston.feature_names)
# 将目标值MEDV添加到DataFrame中
df['MEDV'] = boston.target
# 将数据集分为训练集和测试集
x_train, x_test, y_train, y_test = train_test_split(df[boston.feature_names], df['MEDV'], test_size=0.2, random_state=42)
# 构建GBDT模型
gbdt = GradientBoostingRegressor(n_estimators=100, learning_rate=0.1, max_depth=3, random_state=42)
# 模型训练
gbdt.fit(x_train, y_train)
# 模型评估
y_pred = gbdt.predict(x_test)
mse = mean_squared_error(y_test, y_pred)
print('MSE:', mse)
# 预测房价
new_x = [[0.1, 18.0, 2.0, 0, 0.5, 6.0, 70.0, 3.0, 1.0, 305.0, 19.0, 390.0, 7.0]]
new_y = gbdt.predict(new_x)
print('Predicted MEDV:', new_y)
```
以上代码中,使用load_boston()函数导入Boston房价数据集,然后将数据集转换为DataFrame格式,并将目标值MEDV添加到DataFrame中。使用train_test_split()函数将数据集分为训练集和测试集。使用GradientBoostingRegressor类构建GBDT模型,并使用fit()函数进行模型训练。使用mean_squared_error()函数对训练好的模型进行评估。最后,使用predict()函数对新的房屋特征进行预测,得到预测的房价。
阅读全文
相关推荐
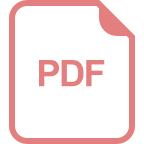






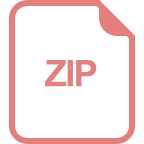
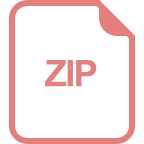
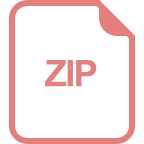
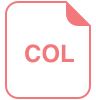
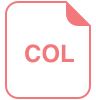
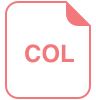
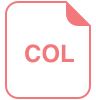


