DataLoader对数据集进行shuffle=True的抽取时,如何能使得一个batch中不出现相同label的数据。给出代码
时间: 2023-12-03 22:44:21 浏览: 68
要实现一个batch中不出现相同label的数据,可以在获取每个batch的数据时,先对数据集按label进行排序,然后按顺序取出每个label的数据,再将这些数据打乱顺序组成一个batch。
以下是一个示例代码:
```python
import torch.utils.data as data
class MyDataset(data.Dataset):
def __init__(self, data_list):
self.data_list = data_list
def __len__(self):
return len(self.data_list)
def __getitem__(self, index):
return self.data_list[index]
class BatchSampler(data.BatchSampler):
def __init__(self, sampler, batch_size, drop_last):
super().__init__(sampler, batch_size, drop_last)
self.sampler = sampler
self.batch_size = batch_size
self.drop_last = drop_last
def __iter__(self):
indices = list(self.sampler)
labels = [self.data_list[i][1] for i in indices]
sorted_indices = [x for _, x in sorted(zip(labels, indices))] # 按label排序
batches = []
batch = []
prev_label = None
for idx in sorted_indices:
label = self.data_list[idx][1]
if prev_label is None or label != prev_label:
# 如果是新的label,将之前的batch加入batches中
if batch:
batches.append(batch)
# 开始新的batch
batch = [idx]
prev_label = label
else:
# 如果是相同的label,继续加入当前batch中
batch.append(idx)
# 将最后一个batch加入batches中
if batch and (not self.drop_last or len(batch) == self.batch_size):
batches.append(batch)
# 打乱每个batch中的顺序
for batch in batches:
random.shuffle(batch)
return iter(batches)
# 使用示例
my_dataset = MyDataset(data_list)
sampler = data.SequentialSampler(my_dataset)
batch_sampler = BatchSampler(sampler, batch_size=4, drop_last=False)
data_loader = data.DataLoader(my_dataset, batch_sampler=batch_sampler)
for batch in data_loader:
# 这里的batch是一个list,包含多个样本,每个样本是一个tuple,第一个元素是数据,第二个元素是label
# 确保每个batch中的label不相同
labels = [x[1] for x in batch]
assert len(set(labels)) == len(labels)
```
这个示例代码中,我们首先定义了一个`MyDataset`类来表示数据集,其中`data_list`是一个元素为`(data, label)`的列表。然后定义了一个`BatchSampler`类来实现自定义的batch采样器,其中`sampler`是一个原始的采样器,`batch_size`是batch大小,`drop_last`表示如果最后一个batch的样本数不足`batch_size`是否丢弃。
在`__iter__`方法中,我们首先将数据集按label排序,然后将每个label的数据按顺序取出,组成一个batch。当遇到新的label时,将之前的batch加入`batches`中,并开始新的batch。最后,将每个batch中的样本顺序打乱后返回。
最后,我们使用`DataLoader`类来加载数据集,其中`batch_sampler`参数使用我们自定义的`BatchSampler`类。在训练时,可以确保每个batch中的label不相同。
阅读全文
相关推荐
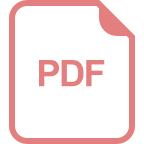
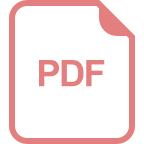
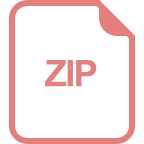

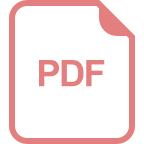
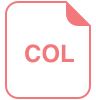
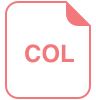
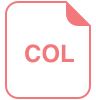
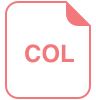
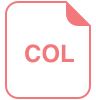
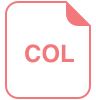
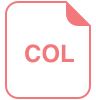

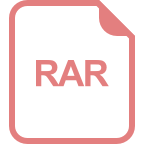
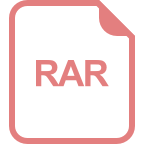
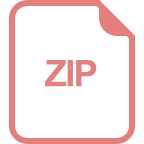