lstm直接多步预测代码
时间: 2023-08-04 13:02:43 浏览: 53
以下是使用LSTM进行多步预测的代码示例:
```python
import numpy as np
from keras.models import Sequential
from keras.layers import LSTM, Dense
# 定义函数以生成样本数据
def generate_data(n):
X = np.arange(n).reshape(n, 1)
y = np.sin(X)
return X, y
# 定义参数
n_steps_in = 3
n_steps_out = 2
n_features = 1
# 生成样本数据
X, y = generate_data(10)
# 将数据转换为LSTM模型所需的格式
X = X.reshape((X.shape[0], X.shape[1], n_features))
# 构建LSTM模型
model = Sequential()
model.add(LSTM(50, activation='relu', input_shape=(n_steps_in, n_features)))
model.add(Dense(n_steps_out))
model.compile(optimizer='adam', loss='mse')
# 训练模型
model.fit(X, y, epochs=200, verbose=0)
# 生成输入序列
x_input = np.array([7, 8, 9]).reshape((1, n_steps_in, n_features))
# 预测未来两个时间步的值
yhat = model.predict(x_input, verbose=0)
print(yhat)
```
在这个示例中,我们首先定义了一个函数来生成样本数据,并设置了多步预测所需的参数。然后我们生成了一些样本数据,并将其转换为LSTM模型所需的格式。接下来,我们构建了一个简单的LSTM模型,并对其进行了训练。最后,我们使用模型来预测未来两个时间步的值,并将其打印出来。
相关推荐
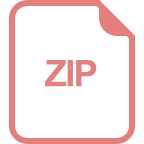














