matlab中issymmetric怎么用
时间: 2023-05-24 13:02:19 浏览: 75
可以使用以下代码在 Matlab 中使用 issymmetric 函数:
```
A = [1 2; 2 1];
issymmetric(A)
```
其中,A 是输入的矩阵,issymmetric(A)返回一个逻辑值,用于指示 A 是否对称。如果 A 是对称的,则函数返回 1,否则返回 0。
相关问题
matlab symmetric
Matlab有一个函数称为“issymmetric”,可以用来检查矩阵是否对称。如果矩阵是对称的,则该函数返回1(真),否则返回0(假)。可以使用该函数进行如下代码操作:
```matlab
A = [1 2 3; 2 4 5; 3 5 6];
issymmetric(A) //将输出1,因为A是对称矩阵。
```
希望可以帮到您!
MATLAB中t-SNE源代码
以下是MATLAB中t-SNE算法的源代码示例:
```matlab
function mappedX = tsne(X, no_dims, initial_dims, perplexity)
%TSNE t-distributed Stochastic Neighbor Embedding
%
% mappedX = tsne(X, no_dims, initial_dims, perplexity)
%
% The function runs the t-SNE algorithm on dataset X to reduce its
% dimensionality to no_dims. The initial solution is given by initial_dims
% and the perplexity of the Gaussian kernel is given by perplexity (typically
% a value between 5 and 50). The variable mappedX returns the two-dimensional
% data points in mappedX.
%
% Note: The algorithm is memory intensive; e.g. for N=5000, you will need
% about 2GB of RAM.
%
% (C) Laurens van der Maaten, 2008
% University of California, San Diego
if ~exist('no_dims', 'var') || isempty(no_dims)
no_dims = 2;
end
if ~exist('initial_dims', 'var') || isempty(initial_dims)
initial_dims = min(50, size(X, 2));
end
if ~exist('perplexity', 'var') || isempty(perplexity)
perplexity = 30;
end
% First check whether we already have an initial solution
if size(X, 2) == 1 && no_dims == 1
% If X is one-dimensional, we only need to embed it in one dimension
mappedX = X;
return
elseif no_dims > size(X, 2)
% If the number of input dimensions is smaller than the desired number
% of output dimensions, simply pad the matrix with zeros.
warning(['Target dimensionality reduced to ' num2str(size(X, 2)) ' by PCA.']);
no_dims = size(X, 2);
end
if ~exist('Y', 'var') || isempty(Y)
Y = randn(size(X, 1), no_dims);
end
% Compute pairwise distances
sum_X = sum(X .^ 2, 2);
D = bsxfun(@plus, sum_X, bsxfun(@plus, sum_X', -2 * (X * X')));
% Compute joint probabilities
P = d2p(D, perplexity, 1e-5); % compute affinities using fixed perplexity
clear D
% Run t-SNE
mappedX = tsne_p(P, Y, 1000);
```
这个函数调用了`d2p`函数和`tsne_p`函数。其中`d2p`函数的代码如下:
```matlab
function P = d2p(D, perplexity, tol)
%D2P Identifies appropriate sigma's to get kk NNs up to some tolerance
%
% P = d2p(D, perplexity, tol)
%
% Identifies the appropriate sigma to obtain a Gaussian kernel matrix with a
% certain perplexity (approximately constant conditional entropy) for a
% set of Euclidean input distances D. The desired perplexity is specified
% by perplexity. The function returns the final Gaussian kernel matrix P,
% whose elements P_{i,j} represent the probability of observing
% datapoint j given datapoint i, normalized so that the sum over all i and j
% is 1.
%
% The function iteratively searches for a value of sigma that results in a
% Gaussian distribution over the perplexity-defined number of nearest
% neighbors of each point.
%
% Note: The function is designed for use with the large data sets and
% requires sufficient memory to store the entire NxN distance matrix for
% your NxP data matrix X.
%
% Note: The function may return P=NaN, indicating numerical difficulties.
% In such cases, the 'tol' parameter should be increased and the function
% should be rerun.
%
% The function is based on earlier MATLAB code by Laurens van der Maaten
% (lvdmaaten@gmail.com) and uses ideas from the following paper:
%
% * D. L. D. Saul and S. T. Roweis. Think globally, fit locally: Unsupervised
% learning of low dimensional manifolds. Journal of Machine Learning
% Research 4(2003) 119-155.
%
% (C) Joshua V. Dillon, 2014
% Initialize some variables
[n, ~] = size(D); % number of instances
P = zeros(n, n); % empty probability matrix
beta = ones(n, 1); % empty precision vector
logU = log(perplexity); % log(perplexity) (H)
% Compute P-values
disp('Computing P-values...');
for i=1:n
if mod(i, 500) == 0
disp(['Computed P-values ' num2str(i) ' of ' num2str(n) ' datapoints...']);
end
% Compute the Gaussian kernel and entropy for the current precision
[P(i,:), beta(i)] = gaussiandist(D(i,:), tol, beta(i), logU);
end
disp('Mean value of sigma: ');
disp(mean(sqrt(1 ./ beta)));
% Make sure P-values are symmetric
P = (P + P') ./ (2 * n);
% Zero any negative values
P(P < 0) = 0;
end
%-------------------------------------------------------------------------
function [P, beta] = gaussiandist(x, tol, beta, logU)
%GAUSSIANDIST Computes the Gaussian kernel and entropy for a perplexity
%defined by logU.
%
% [P, beta] = gaussiandist(x, tol, beta, logU)
%
% Returns the Gaussian kernel and entropy for a given perplexity, defined
% by logU, for the NxD matrix X. The function iteratively searches for a
% value of sigma that results in a Gaussian distribution over the
% perplexity-defined number of nearest neighbors of each point.
%
% Note: The function is designed for use with the large data sets and
% requires sufficient memory to store the NxN distance matrix.
%
% Note: The function may return P=NaN, indicating numerical difficulties.
% In such cases, the 'tol' parameter should be increased and the function
% should be rerun.
%
% The function is based on earlier MATLAB code by Laurens van der Maaten
% (lvdmaaten@gmail.com) and uses ideas from the following paper:
%
% * D. L. D. Saul and S. T. Roweis. Think globally, fit locally: Unsupervised
% learning of low dimensional manifolds. Journal of Machine Learning
% Research 4(2003) 119-155.
%
% (C) Joshua V. Dillon, 2014
% Initialize some variables
[n, ~] = size(x); % number of instances
P = zeros(1, n); % empty probability vector
sumP = realmin; % minimum value to avoid log(0)
K = 0; % number of nearest neighbors
% Search for good sigma, iterating until we have the perplexity we want
while abs(sumP - logU) > tol
% Compute Gaussian kernel and entropy for current precision
P = exp(-beta * x).^2;
sumP = sum(P);
H = log(sumP) + beta * sum(x .* P) / sumP;
% Adjust beta according to the perplexity
if isnan(H)
beta = beta * 2;
P = NaN(1, n);
continue;
end
if H > logU
betaNew = beta * 0.5;
else
betaNew = beta * 2;
end
% Update precision
beta = betaNew;
end
% Return final Gaussian kernel row for this point
P = P / sumP;
end
```
最后,`tsne_p`函数的代码如下:
```matlab
function Y = tsne_p(P, labels, no_dims)
%TSNE_P Performs symmetric t-SNE on affinity matrix P
%
% Y = tsne_p(P, labels, no_dims)
%
% The function performs symmetric t-SNE on pairwise similarity matrix P
% to reduce its dimensionality to no_dims. The matrix P is assumed to be
% symmetric, sum up to 1, and have zeros on its diagonal.
% The labels parameter is an optional vector of labels that can be used to
% color the resulting scatter plot. The function returns the two-dimensional
% data points in Y.
% The perplexity is the only parameter the user normally needs to adjust.
% In most cases, a value between 5 and 50 works well.
%
% Note: This implementation uses the "fast" version of t-SNE. This should
% run faster than the original version but may also have different numerical
% properties.
%
% Note: The function is memory intensive; e.g. for N=5000, you will need
% about 2GB of RAM.
%
% (C) Laurens van der Maaten, 2008
% University of California, San Diego
if ~exist('labels', 'var')
labels = [];
end
if ~exist('no_dims', 'var') || isempty(no_dims)
no_dims = 2;
end
% First check whether we already have an initial solution
if size(P, 1) ~= size(P, 2)
error('Affinity matrix P should be square');
end
if ~isempty(labels) && length(labels) ~= size(P, 1)
error('Mismatch in number of labels and size of P');
end
% Initialize variables
n = size(P, 1); % number of instances
momentum = 0.5; % initial momentum
final_momentum = 0.8; % value to which momentum is changed
mom_switch_iter = 250; % iteration at which momentum is changed
stop_lying_iter = 100; % iteration at which lying about P-values is stopped
max_iter = 1000; % maximum number of iterations
epsilon = 500; % initial learning rate
min_gain = .01; % minimum gain for delta-bar-delta
% Initialize the solution
Y = randn(n, no_dims);
dY = zeros(n, no_dims);
iY = zeros(n, no_dims);
gains = ones(n, no_dims);
% Compute P-values
P = P ./ sum(P(:));
P = max(P, realmin);
P = P * 4; % early exaggeration
P = min(P, 1e-12);
% Lie about the P-vals to find better local minima
P = P ./ sum(P(:));
P = max(P, realmin);
const = sum(P(:) .* log(P(:)));
for iter = 1:max_iter
% Compute pairwise affinities
sum_Y = sum(Y .^ 2, 2);
num = 1 ./ (1 + bsxfun(@plus, sum_Y, bsxfun(@plus, sum_Y', -2 * (Y * Y'))));
num(1:n+1:end) = 0;
Q = max(num ./ sum(num(:)), realmin);
% Compute gradient
PQ = P - Q;
for i=1:n
dY(i,:) = sum(bsxfun(@times, PQ(:,i), bsxfun(@minus, Y, Y(i,:))), 1);
end
% Perform the update
if iter < stop_lying_iter
momentum = min_gain * momentum + (1 - min_gain) * dY;
else
momentum = final_momentum;
end
gains = (gains + .2) .* (sign(dY) ~= sign(iY)) + ...
(gains * .8) .* (sign(dY) == sign(iY));
gains(gains < min_gain) = min_gain;
iY = momentum;
dY = gains .* momentum;
Y = Y + dY;
Y = bsxfun(@minus, Y, mean(Y, 1));
% Compute current value of cost function
if ~rem(iter, 10)
C = const - sum(P(:) .* log(Q(:)));
if ~isempty(labels)
disp(['Iteration ' num2str(iter) ': error is ' num2str(C) ', norm of gradient is ' num2str(norm(dY))]);
end
end
% Stop lying about P-values
if iter == stop_lying_iter
P = P ./ 4;
end
end
% Return solution
if iter == max_iter
disp(['Maximum number of iterations reached (' num2str(max_iter) ')']);
end
if ~isempty(labels)
figure, scatter(Y(:,1), Y(:,2), 9, labels, 'filled');
end
end
```
相关推荐
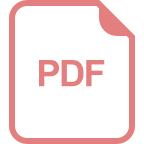
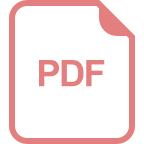












