用pytorch写一段代码:以西储大学CWRU轴承故障的振动信号数据库作为模型的训练集,故障类型标签包括:正常、内圈故障、外圈故障和滚动体故障四类。首先将一维故障信号转换为二维灰度图作为CNN模型的输...
时间: 2024-05-16 10:16:51 浏览: 170
以下是一个简单的示例代码,用于将一维信号转换为二维灰度图,并使用PyTorch中的CNN模型进行训练。
首先,需要导入必要的Python库和CWRU轴承故障的振动信号数据库。
```python
import numpy as np
import pandas as pd
import matplotlib.pyplot as plt
import torch
import torch.nn as nn
import torch.optim as optim
from torch.utils.data import Dataset, DataLoader
from sklearn.model_selection import train_test_split
# Load the CWRU bearing dataset
data = pd.read_csv("cwru_data.csv")
labels = pd.read_csv("cwru_labels.csv")
```
然后,我们将一维信号转换为二维灰度图。我们可以使用Python的OpenCV库进行这个过程,也可以手动编写代码来完成这个过程。这里,我们演示手动编写代码的过程。
```python
# Convert 1D signals to 2D grayscale images
def signal_to_image(signal):
image = np.zeros((256, 256))
for i in range(256):
for j in range(256):
image[i][j] = signal[i*256+j]
return image
# Convert all signals to images
images = []
for i in range(len(data)):
images.append(signal_to_image(data.iloc[i]))
# Convert images to PyTorch tensors
images = torch.tensor(images).unsqueeze(1)
```
接下来,我们需要将故障类型标签转换为数字标签。
```python
# Convert labels to numerical labels
label_map = {"Normal": 0, "Inner": 1, "Outer": 2, "Rolling": 3}
labels = labels.replace({"Fault": label_map})
```
然后,我们可以将数据集拆分为训练集和测试集。
```python
# Split the dataset into train and test sets
train_images, test_images, train_labels, test_labels = train_test_split(images, labels, test_size=0.2, random_state=42)
```
最后,我们可以定义CNN模型并进行训练。
```python
# Define the CNN model
class CNN(nn.Module):
def __init__(self):
super(CNN, self).__init__()
self.conv1 = nn.Conv2d(1, 32, 3, padding=1)
self.pool = nn.MaxPool2d(2, 2)
self.conv2 = nn.Conv2d(32, 64, 3, padding=1)
self.fc1 = nn.Linear(64 * 64 * 64, 128)
self.fc2 = nn.Linear(128, 4)
def forward(self, x):
x = self.pool(nn.functional.relu(self.conv1(x)))
x = self.pool(nn.functional.relu(self.conv2(x)))
x = x.view(-1, 64 * 64 * 64)
x = nn.functional.relu(self.fc1(x))
x = self.fc2(x)
return x
# Initialize the model and loss function
model = CNN()
criterion = nn.CrossEntropyLoss()
optimizer = optim.SGD(model.parameters(), lr=0.001, momentum=0.9)
# Train the model
for epoch in range(10):
running_loss = 0.0
for i, data in enumerate(trainloader, 0):
inputs, labels = data
optimizer.zero_grad()
outputs = model(inputs)
loss = criterion(outputs, labels)
loss.backward()
optimizer.step()
running_loss += loss.item()
if i % 200 == 199:
print('[%d, %5d] loss: %.3f' % (epoch + 1, i + 1, running_loss / 200))
running_loss = 0.0
# Test the model
correct = 0
total = 0
with torch.no_grad():
for data in testloader:
images, labels = data
outputs = model(images)
_, predicted = torch.max(outputs.data, 1)
total += labels.size(0)
correct += (predicted == labels).sum().item()
print('Accuracy of the network on the test images: %d %%' % (100 * correct / total))
```
这里,我们使用了一个简单的CNN模型,并在训练集上进行了10个epoch的训练。最后,我们在测试集上评估了模型的准确性。
阅读全文
相关推荐
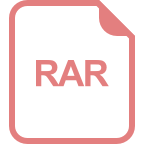
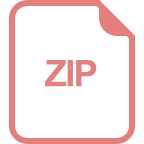
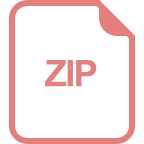
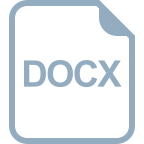
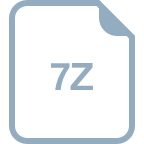
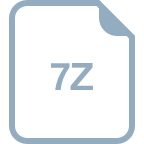
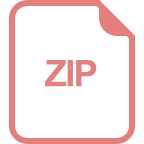
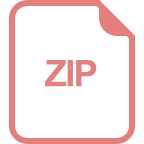
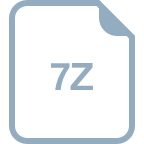
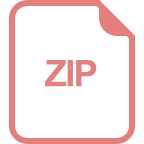