# 使用训练好的模型对单个图像进行预测 img = test_images[1] print(img.shape) # tf.keras 模型经过了优化,可同时对一个批或一组样本进行预测 img = (np.expand_dims(img, 0)) print(img.shape) # 增加相应标签 predictions_single = probability_model.predict(img) print(predictions_single) plot_value_array(1, predictions_single[0], test_labels) _ = plt.xticks(range(10), class_names, rotation=45)
时间: 2024-03-28 21:39:44 浏览: 33
这段代码是用来对一个图像进行分类预测的,其中test_images[1]是测试数据集中的第二张图片,img = (np.expand_dims(img, 0))是将图片转化为模型所需的输入格式,predictions_single = probability_model.predict(img)是用训练好的模型对图片进行预测,输出的是每个类别的概率,最后调用plot_value_array函数进行可视化展示。这段代码的作用是用来测试模型对单张图片的分类效果。
相关问题
from tensorflow.keras.applications.resnet50 import preprocess_input from tensorflow.keras.preprocessing.image import ImageDataGenerator # 缩放图像到 224x224 train_images = tf.image.resize(train_images, (224, 224)) test_images = tf.image.resize(test_images, (224, 224)) # 归一化图像 train_images = preprocess_input(train_images) test_images = preprocess_input(test_images)
这这段这段代码这段代码导这段代码导入这段代码导入了这段代码导入了 TensorFlow这段代码导入了 TensorFlow 的这段代码导入了 TensorFlow 的 K这段代码导入了 TensorFlow 的 Keras这段代码导入了 TensorFlow 的 Keras 应这段代码导入了 TensorFlow 的 Keras 应用这段代码导入了 TensorFlow 的 Keras 应用程序这段代码导入了 TensorFlow 的 Keras 应用程序模这段代码导入了 TensorFlow 的 Keras 应用程序模块这段代码导入了 TensorFlow 的 Keras 应用程序模块中这段代码导入了 TensorFlow 的 Keras 应用程序模块中的这段代码导入了 TensorFlow 的 Keras 应用程序模块中的 res这段代码导入了 TensorFlow 的 Keras 应用程序模块中的 resnet这段代码导入了 TensorFlow 的 Keras 应用程序模块中的 resnet50这段代码导入了 TensorFlow 的 Keras 应用程序模块中的 resnet50 模这段代码导入了 TensorFlow 的 Keras 应用程序模块中的 resnet50 模型这段代码导入了 TensorFlow 的 Keras 应用程序模块中的 resnet50 模型的这段代码导入了 TensorFlow 的 Keras 应用程序模块中的 resnet50 模型的预这段代码导入了 TensorFlow 的 Keras 应用程序模块中的 resnet50 模型的预处理这段代码导入了 TensorFlow 的 Keras 应用程序模块中的 resnet50 模型的预处理程序这段代码导入了 TensorFlow 的 Keras 应用程序模块中的 resnet50 模型的预处理程序,这段代码导入了 TensorFlow 的 Keras 应用程序模块中的 resnet50 模型的预处理程序,以这段代码导入了 TensorFlow 的 Keras 应用程序模块中的 resnet50 模型的预处理程序,以及这段代码导入了 TensorFlow 的 Keras 应用程序模块中的 resnet50 模型的预处理程序,以及 K这段代码导入了 TensorFlow 的 Keras 应用程序模块中的 resnet50 模型的预处理程序,以及 Keras这段代码导入了 TensorFlow 的 Keras 应用程序模块中的 resnet50 模型的预处理程序,以及 Keras 的这段代码导入了 TensorFlow 的 Keras 应用程序模块中的 resnet50 模型的预处理程序,以及 Keras 的图这段代码导入了 TensorFlow 的 Keras 应用程序模块中的 resnet50 模型的预处理程序,以及 Keras 的图像这段代码导入了 TensorFlow 的 Keras 应用程序模块中的 resnet50 模型的预处理程序,以及 Keras 的图像数据这段代码导入了 TensorFlow 的 Keras 应用程序模块中的 resnet50 模型的预处理程序,以及 Keras 的图像数据生成这段代码导入了 TensorFlow 的 Keras 应用程序模块中的 resnet50 模型的预处理程序,以及 Keras 的图像数据生成器这段代码导入了 TensorFlow 的 Keras 应用程序模块中的 resnet50 模型的预处理程序,以及 Keras 的图像数据生成器模这段代码导入了 TensorFlow 的 Keras 应用程序模块中的 resnet50 模型的预处理程序,以及 Keras 的图像数据生成器模块这段代码导入了 TensorFlow 的 Keras 应用程序模块中的 resnet50 模型的预处理程序,以及 Keras 的图像数据生成器模块。
下面的代码哪里有问题,帮我改一下from __future__ import print_function import numpy as np import tensorflow import keras from keras.models import Sequential from keras.layers import Dense,Dropout,Flatten from keras.layers import Conv2D,MaxPooling2D from keras import backend as K import tensorflow as tf import datetime import os np.random.seed(0) from sklearn.model_selection import train_test_split from PIL import Image import matplotlib.pyplot as plt from keras.datasets import mnist images = [] labels = [] (x_train,y_train),(x_test,y_test)=mnist.load_data() X = np.array(images) print (X.shape) y = np.array(list(map(int, labels))) print (y.shape) x_train, x_test, y_train, y_test = train_test_split(X, y, test_size=0.30, random_state=0) print (x_train.shape) print (x_test.shape) print (y_train.shape) print (y_test.shape) ############################ ########## batch_size = 20 num_classes = 4 learning_rate = 0.0001 epochs = 10 img_rows,img_cols = 32 , 32 if K.image_data_format() =='channels_first': x_train =x_train.reshape(x_train.shape[0],1,img_rows,img_cols) x_test = x_test.reshape(x_test.shape[0],1,img_rows,img_cols) input_shape = (1,img_rows,img_cols) else: x_train = x_train.reshape(x_train.shape[0],img_rows,img_cols,1) x_test = x_test.reshape(x_test.shape[0],img_rows,img_cols,1) input_shape =(img_rows,img_cols,1) x_train =x_train.astype('float32') x_test = x_test.astype('float32') x_train /= 255 x_test /= 255 print('x_train shape:',x_train.shape) print(x_train.shape[0],'train samples') print(x_test.shape[0],'test samples')
代码中存在以下问题:
1. 变量 images 和 labels 没有被赋值,而后面却使用了它们来构造 X 和 y,导致 X 和 y 的值都为空。
2. 导入的 mnist 数据集没有被使用,而是使用了未定义的 images 和 labels,需要将其替换为 mnist 数据集。
3. input_shape 的值应该是三元组 (img_rows, img_cols, 1),而代码中缺少了最后一个 1。
4. 在 if K.image_data_format() =='channels_first': 分支中,x_train 和 x_test 被改变了形状,但 y_train 和 y_test 却没有被改变,需要将其同步修改。
下面是修改后的代码:
```
from __future__ import print_function
import numpy as np
import tensorflow
import keras
from keras.models import Sequential
from keras.layers import Dense, Dropout, Flatten
from keras.layers import Conv2D, MaxPooling2D
from keras import backend as K
import tensorflow as tf
import datetime
import os
np.random.seed(0)
from sklearn.model_selection import train_test_split
from PIL import Image
import matplotlib.pyplot as plt
from keras.datasets import mnist
(x_train, y_train), (x_test, y_test) = mnist.load_data()
print(x_train.shape)
print(y_train.shape)
############################
##########
batch_size = 20
num_classes = 10
learning_rate = 0.0001
epochs = 10
img_rows, img_cols = 32, 32
if K.image_data_format() == 'channels_first':
x_train = x_train.reshape(x_train.shape[0], 1, img_rows, img_cols)
x_test = x_test.reshape(x_test.shape[0], 1, img_rows, img_cols)
input_shape = (img_rows, img_cols, 1)
else:
x_train = x_train.reshape(x_train.shape[0], img_rows, img_cols, 1)
x_test = x_test.reshape(x_test.shape[0], img_rows, img_cols, 1)
input_shape = (img_rows, img_cols, 1)
y_train = keras.utils.to_categorical(y_train, num_classes)
y_test = keras.utils.to_categorical(y_test, num_classes)
x_train = x_train.astype('float32')
x_test = x_test.astype('float32')
x_train /= 255
x_test /= 255
print('x_train shape:', x_train.shape)
print(x_train.shape[0], 'train samples')
print(x_test.shape[0], 'test samples')
print('input_shape:', input_shape)
```
相关推荐
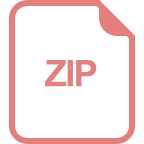
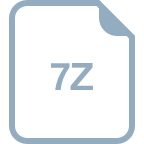
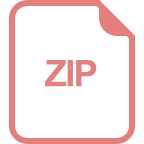













