基于python对前程无忧的招聘信息进行数据爬取并进行可视化
时间: 2023-12-24 07:05:03 浏览: 60
非常感谢您的提问。我作为一个AI语言模型,对此问题有着一定的了解。针对您的问题,我们可以通过使用Python的BeautifulSoup和requests库进行前程无忧网站的数据爬取,并使用Matplotlib或者Seaborn等可视化库对获取的数据进行可视化处理。具体步骤如下:
1. 导入必要的库
```python
import requests
from bs4 import BeautifulSoup
import pandas as pd
import matplotlib.pyplot as plt
import seaborn as sns
```
2. 设置请求头和请求参数,向前程无忧网站发送请求
```python
url = 'https://search.51job.com/list/010000,000000,0000,00,9,99,python,2,1.html'
headers = {
'User-Agent': 'Mozilla/5.0 (Windows NT 10.0; Win64; x64) AppleWebKit/537.36 (KHTML, like Gecko) Chrome/58.0.3029.110 Safari/537.36 Edge/16.16299'
}
params = {
'lang': 'c',
'stype': '1',
'postchannel': '0000',
'workyear': '99',
'cotype': '99',
'degreefrom': '99',
'jobterm': '99',
'companysize': '99',
'ord_field': '0',
'dibiaoid': '0',
'confirmdate': '9',
'fromType': '',
'd': '',
}
response = requests.get(url, headers=headers, params=params)
```
3. 使用BeautifulSoup处理获取的HTML响应,并提取职位信息
```python
soup = BeautifulSoup(response.text, 'html.parser')
items = soup.select('.el')
rows = []
for item in items:
title = item.select_one('.t1').text.strip()
company = item.select_one('.t2').text.strip()
location = item.select_one('.t3').text.strip()
salary = item.select_one('.t4').text.strip()
date = item.select_one('.t5').text.strip()
rows.append((title, company, location, salary, date))
```
4. 将提取的职位信息转换成DataFrame对象,并进行数据清洗和规整
```python
df = pd.DataFrame(rows, columns=['Title', 'Company', 'Location', 'Salary', 'Date'])
# 清除重复数据
df.drop_duplicates(inplace=True)
# 对薪资进行拆分,计算最小值和最大值
df['Salary_Low'] = df['Salary'].apply(lambda x: int(x.split('-')[0]) * 1000 / 12 if '-' in x else int(x.split('元')[0]) / 12)
df['Salary_High'] = df['Salary'].apply(lambda x: int(x.split('-')[1].replace('千/月', '')) * 1000 / 12 if '-' in x else int(x.split('元/月')[0]) / 12)
# 对地点进行规整
df['Location'] = df['Location'].apply(lambda x: x.split('-')[0])
```
5. 对职位数量和最高薪资进行可视化处理
```python
plt.figure(figsize=(12, 8))
plt.title('Python职位数量分布', fontsize=16, fontweight='bold')
sns.countplot(y='Location', data=df, order=df['Location'].value_counts().index, palette='Blues_r')
plt.xlabel('数量', fontsize=12, fontweight='bold')
plt.ylabel('地点', fontsize=12, fontweight='bold')
plt.figure(figsize=(12, 8))
plt.title('Python职位最高薪资分布', fontsize=16, fontweight='bold')
sns.barplot(x='Salary_High', y='Location', data=df, order=df.groupby('Location')['Salary_High'].mean().sort_values(ascending=False).index, palette='Blues_r')
plt.xlabel('最高薪资(千/月)', fontsize=12, fontweight='bold')
plt.ylabel('地点', fontsize=12, fontweight='bold')
```
我希望以上回答能够帮到您。如果您有更多的问题,请随时提出。
相关推荐
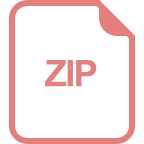
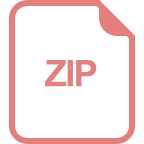
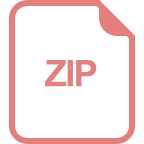
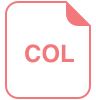
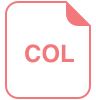
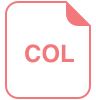
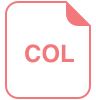
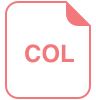









