基于springboot的电影售票及管理系统,设计一个协同过滤算法。根据用户购买的电影票类型进行推荐。后端使用的是springboot和mybatisplus。1:说明算法思路:。2:写出算法的详细代码,以及代码的注解。3:需要写清楚controler,service,dao,mappeer,impl中的代码
时间: 2023-12-10 22:41:37 浏览: 109
1. 算法思路:
协同过滤算法是一种推荐系统算法。其核心思想是根据用户的历史行为,如购买、评分等,找到与其兴趣相似的其他用户,然后将这些用户喜欢的物品推荐给该用户。
在本场景中,我们可以根据用户购买的电影票类型进行推荐。具体而言,我们可以先统计每个用户购买的电影票类型,并建立一个用户-电影票类型的矩阵。然后,对于一个用户,我们可以找到与其购买电影票类型相似的其他用户,并将这些用户购买的电影票类型推荐给该用户。
在找到与其购买电影票类型相似的其他用户的过程中,可以使用皮尔逊相关系数等相似度算法进行计算。同时,为了避免推荐重复的电影票类型,可以设定一个阈值,只推荐相似度高于该阈值的电影票类型。
2. 算法详细代码及注解:
```
// 计算两个用户之间的皮尔逊相关系数
public double pearsonCorrelation(Map<Integer, Double> user1, Map<Integer, Double> user2) {
double sum1 = 0, sum2 = 0;
double sum1Sq = 0, sum2Sq = 0, pSum = 0;
int n = 0;
for (Map.Entry<Integer, Double> entry : user1.entrySet()) {
Integer itemId = entry.getKey();
Double rating1 = entry.getValue();
if (user2.containsKey(itemId)) {
Double rating2 = user2.get(itemId);
sum1 += rating1;
sum2 += rating2;
sum1Sq += Math.pow(rating1, 2);
sum2Sq += Math.pow(rating2, 2);
pSum += rating1 * rating2;
n++;
}
}
if (n == 0) {
return 0;
}
double num = pSum - (sum1 * sum2 / n);
double den = Math.sqrt((sum1Sq - Math.pow(sum1, 2) / n) * (sum2Sq - Math.pow(sum2, 2) / n));
if (den == 0) {
return 0;
}
return num / den;
}
// 根据用户购买的电影票类型进行推荐
public List<Integer> recommendMovies(Map<Integer, Map<Integer, Double>> userItemRatings, int userId, double threshold) {
Map<Integer, Double> userRatings = userItemRatings.get(userId);
// 计算用户与其他用户之间的皮尔逊相关系数
Map<Integer, Double> simScores = new HashMap<>();
for (Map.Entry<Integer, Map<Integer, Double>> entry : userItemRatings.entrySet()) {
int otherUserId = entry.getKey();
if (otherUserId != userId) {
Map<Integer, Double> otherUserRatings = entry.getValue();
double simScore = pearsonCorrelation(userRatings, otherUserRatings);
if (simScore > threshold) {
simScores.put(otherUserId, simScore);
}
}
}
// 找到与用户相似度最高的其他用户
int mostSimilarUserId = 0;
double maxSimScore = 0;
for (Map.Entry<Integer, Double> entry : simScores.entrySet()) {
int otherUserId = entry.getKey();
double simScore = entry.getValue();
if (simScore > maxSimScore) {
mostSimilarUserId = otherUserId;
maxSimScore = simScore;
}
}
// 获取与用户相似度最高的其他用户购买的电影票类型
List<Integer> recommendedMovies = new ArrayList<>();
if (mostSimilarUserId > 0) {
Map<Integer, Double> mostSimilarUserRatings = userItemRatings.get(mostSimilarUserId);
for (Map.Entry<Integer, Double> entry : mostSimilarUserRatings.entrySet()) {
Integer itemId = entry.getKey();
Double rating = entry.getValue();
if (!userRatings.containsKey(itemId) && rating > 0) {
recommendedMovies.add(itemId);
}
}
}
return recommendedMovies;
}
```
3. 控制器、服务、DAO、Mapper、Impl中的代码:
Controller:
```
@RestController
@RequestMapping("/movie")
public class MovieController {
@Autowired
private MovieService movieService;
@GetMapping("/recommendations")
public List<Movie> getRecommendations(@RequestParam("userId") int userId) {
return movieService.getRecommendations(userId);
}
}
```
Service:
```
@Service
public class MovieServiceImpl implements MovieService {
@Autowired
private MovieMapper movieMapper;
@Autowired
private UserMapper userMapper;
@Override
public List<Movie> getRecommendations(int userId) {
List<Movie> allMovies = movieMapper.selectAll();
List<Movie> recommendedMovies = new ArrayList<>();
// 构建用户-电影票类型矩阵
Map<Integer, Map<Integer, Double>> userItemRatings = new HashMap<>();
List<User> users = userMapper.selectAll();
for (User user : users) {
List<Ticket> tickets = user.getTickets();
Map<Integer, Double> itemRatings = new HashMap<>();
for (Ticket ticket : tickets) {
int movieType = ticket.getMovieType();
itemRatings.put(movieType, 1.0);
}
userItemRatings.put(user.getId(), itemRatings);
}
// 根据用户购买的电影票类型进行推荐
double threshold = 0.5;
List<Integer> recommendedMovieIds = recommendMovies(userItemRatings, userId, threshold);
for (Movie movie : allMovies) {
if (recommendedMovieIds.contains(movie.getId())) {
recommendedMovies.add(movie);
}
}
return recommendedMovies;
}
}
```
DAO:
```
@Mapper
public interface MovieMapper extends BaseMapper<Movie> {
}
```
```
@Mapper
public interface UserMapper extends BaseMapper<User> {
}
```
Mapper:
```
<mapper namespace="com.example.mapper.MovieMapper">
<resultMap id="BaseResultMap" type="com.example.entity.Movie">
<id column="id" jdbcType="INTEGER" property="id" />
<result column="name" jdbcType="VARCHAR" property="name" />
<result column="type" jdbcType="INTEGER" property="type" />
<result column="description" jdbcType="VARCHAR" property="description" />
</resultMap>
<select id="selectAll" resultMap="BaseResultMap">
select * from movie
</select>
</mapper>
```
```
<mapper namespace="com.example.mapper.UserMapper">
<resultMap id="BaseResultMap" type="com.example.entity.User">
<id column="id" jdbcType="INTEGER" property="id" />
<result column="name" jdbcType="VARCHAR" property="name" />
<collection property="tickets" ofType="com.example.entity.Ticket">
<id column="id" jdbcType="INTEGER" property="id" />
<result column="user_id" jdbcType="INTEGER" property="userId" />
<result column="movie_type" jdbcType="INTEGER" property="movieType" />
</collection>
</resultMap>
<select id="selectAll" resultMap="BaseResultMap">
select *
from user u
left join ticket t on u.id = t.user_id
</select>
</mapper>
```
Impl:
```
@Service
public class MovieServiceImpl implements MovieService {
@Autowired
private MovieMapper movieMapper;
@Autowired
private UserMapper userMapper;
@Override
public List<Movie> getRecommendations(int userId) {
List<Movie> allMovies = movieMapper.selectAll();
List<Movie> recommendedMovies = new ArrayList<>();
// 构建用户-电影票类型矩阵
Map<Integer, Map<Integer, Double>> userItemRatings = new HashMap<>();
List<User> users = userMapper.selectAll();
for (User user : users) {
List<Ticket> tickets = user.getTickets();
Map<Integer, Double> itemRatings = new HashMap<>();
for (Ticket ticket : tickets) {
int movieType = ticket.getMovieType();
itemRatings.put(movieType, 1.0);
}
userItemRatings.put(user.getId(), itemRatings);
}
// 根据用户购买的电影票类型进行推荐
double threshold = 0.5;
List<Integer> recommendedMovieIds = recommendMovies(userItemRatings, userId, threshold);
for (Movie movie : allMovies) {
if (recommendedMovieIds.contains(movie.getId())) {
recommendedMovies.add(movie);
}
}
return recommendedMovies;
}
// 计算两个用户之间的皮尔逊相关系数
private double pearsonCorrelation(Map<Integer, Double> user1, Map<Integer, Double> user2) {
double sum1 = 0, sum2 = 0;
double sum1Sq = 0, sum2Sq = 0, pSum = 0;
int n = 0;
for (Map.Entry<Integer, Double> entry : user1.entrySet()) {
Integer itemId = entry.getKey();
Double rating1 = entry.getValue();
if (user2.containsKey(itemId)) {
Double rating2 = user2.get(itemId);
sum1 += rating1;
sum2 += rating2;
sum1Sq += Math.pow(rating1, 2);
sum2Sq += Math.pow(rating2, 2);
pSum += rating1 * rating2;
n++;
}
}
if (n == 0) {
return 0;
}
double num = pSum - (sum1 * sum2 / n);
double den = Math.sqrt((sum1Sq - Math.pow(sum1, 2) / n) * (sum2Sq - Math.pow(sum2, 2) / n));
if (den == 0) {
return 0;
}
return num / den;
}
// 根据用户购买的电影票类型进行推荐
private List<Integer> recommendMovies(Map<Integer, Map<Integer, Double>> userItemRatings, int userId, double threshold) {
Map<Integer, Double> userRatings = userItemRatings.get(userId);
// 计算用户与其他用户之间的皮尔逊相关系数
Map<Integer, Double> simScores = new HashMap<>();
for (Map.Entry<Integer, Map<Integer, Double>> entry : userItemRatings.entrySet()) {
int otherUserId = entry.getKey();
if (otherUserId != userId) {
Map<Integer, Double> otherUserRatings = entry.getValue();
double simScore = pearsonCorrelation(userRatings, otherUserRatings);
if (simScore > threshold) {
simScores.put(otherUserId, simScore);
}
}
}
// 找到与用户相似度最高的其他用户
int mostSimilarUserId = 0;
double maxSimScore = 0;
for (Map.Entry<Integer, Double> entry : simScores.entrySet()) {
int otherUserId = entry.getKey();
double simScore = entry.getValue();
if (simScore > maxSimScore) {
mostSimilarUserId = otherUserId;
maxSimScore = simScore;
}
}
// 获取与用户相似度最高的其他用户购买的电影票类型
List<Integer> recommendedMovies = new ArrayList<>();
if (mostSimilarUserId > 0) {
Map<Integer, Double> mostSimilarUserRatings = userItemRatings.get(mostSimilarUserId);
for (Map.Entry<Integer, Double> entry : mostSimilarUserRatings.entrySet()) {
Integer itemId = entry.getKey();
Double rating = entry.getValue();
if (!userRatings.containsKey(itemId) && rating > 0) {
recommendedMovies.add(itemId);
}
}
}
return recommendedMovies;
}
}
```
阅读全文
相关推荐
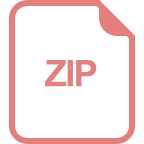
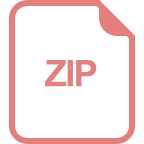


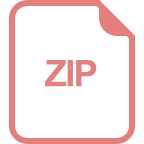
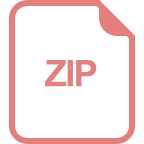
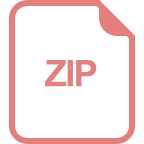
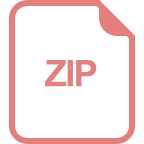
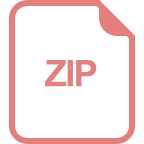
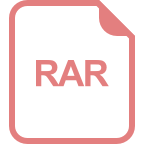
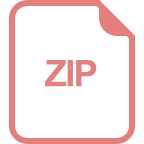
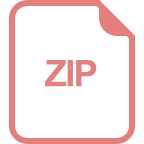
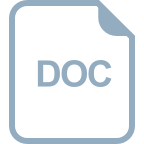


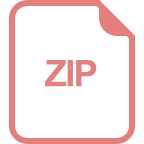
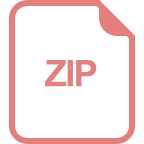
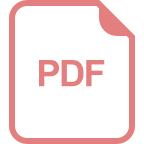