np.linalg.matrix_rank
时间: 2023-04-27 15:04:27 浏览: 293
np.linalg.matrix_rank是numpy中的一个函数,用于计算矩阵的秩。矩阵的秩是指矩阵中线性无关的行或列的最大数量。如果矩阵的秩小于它的行数和列数中的较小值,则矩阵是奇异的,否则矩阵是非奇异的。
相关问题
在python中 通过 np.linalg.matrix_rank(A) 得到A 的秩不是满秩,但是 通过np.linalg.det(A) 得到A的行列式不等于零
是的,你提到的方法也是计算矩阵秩和行列式的常用方法之一。在Python中,使用numpy库中的np.linalg.matrix_rank函数可以计算矩阵的秩,而np.linalg.det函数可以计算矩阵的行列式。
下面是一个示例代码,演示如何通过numpy计算矩阵的秩和行列式:
```python
import numpy as np
# 定义一个矩阵
A = np.array([[1, 2, 3],
[4, 5, 6],
[7, 8, 9]])
# 计算矩阵的秩
rank = np.linalg.matrix_rank(A)
# 计算矩阵的行列式
det = np.linalg.det(A)
print("矩阵的秩为:", rank)
print("矩阵的行列式为:", det)
```
运行以上代码,将会输出矩阵A的秩和行列式。如果矩阵A的行列式不等于零,但秩小于矩阵的维度,则说明该矩阵不是满秩矩阵。
np.linalg.lstsq
`np.linalg.lstsq` is a NumPy function that solves the least-squares problem, which is finding the best-fitting linear equation for a set of data points. It takes as input a matrix `A` representing the data points, a vector `b` representing the target values, and an optional parameter `rcond` that specifies the cutoff for small singular values. The function returns a tuple `(x, residuals, rank, s)` where `x` is the solution to the least-squares problem, `residuals` is the sum of squared residuals, `rank` is the rank of matrix `A`, and `s` is an array containing the singular values of `A`.
The solution `x` represents the coefficients of the linear equation that best fits the data. If `A` has more columns than rows, the solution is an over-determined system and the best-fitting solution is obtained by minimizing the sum of squared residuals. If `A` has more rows than columns, the solution is an under-determined system and the least-norm solution is obtained by minimizing the norm of `x`.
阅读全文
相关推荐
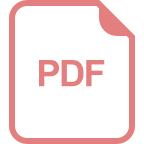
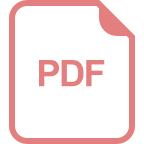
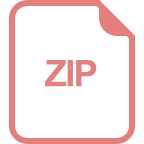



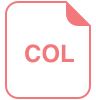
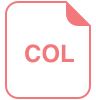
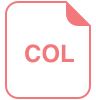
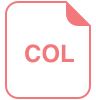
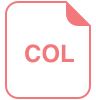
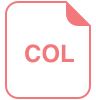
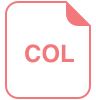
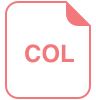



