compressed_image.show()
时间: 2023-09-16 22:10:01 浏览: 109
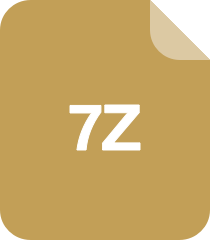
CompressImage
如果你在使用 `compressed_image.show()` 时遇到了 `AttributeError: 'NoneType' object has no attribute 'show'` 的错误,这可能是因为 PIL Image 对象没有正确地创建。
在 `compress_image` 函数中,我们使用了以下代码来将压缩后的图像数据转换为 PIL Image 对象:
```python
compressed_image = Image.fromarray(np.uint8(compressed_data))
```
如果该代码没有正确地执行,那么 `compressed_image` 将是一个空值(即 NoneType),这会导致 `compressed_image.show()` 报错。
解决这个问题的方法是,在调用 `compressed_image.show()` 之前,添加以下代码来检查 `compressed_image` 是否为空:
```python
if compressed_image is not None:
compressed_image.show()
```
如果 `compressed_image` 不为空,那么就会显示压缩后的图像;否则,就不会进行显示。这样可以避免出现 `AttributeError: 'NoneType' object has no attribute 'show'` 的错误。
阅读全文
相关推荐
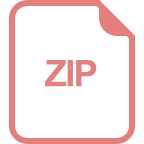








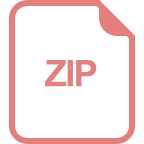
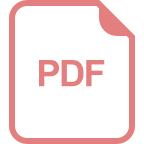
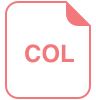
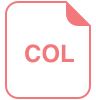
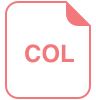
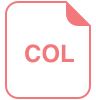
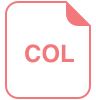
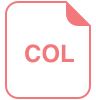
