batch runtimeerror: trying to backward through the graph a second time
时间: 2023-12-19 11:03:46 浏览: 26
这个错误通常是因为在计算图(computation graph)中进行了两次反向传播(backpropagation),而计算图只能被反向传播一次。在 PyTorch 中,你可以通过调用 `detach()` 或者 `with torch.no_grad():` 来避免梯度的传递,从而避免这个错误。如果你需要多次反向传播,可以使用 `torch.autograd.backward()` 函数手动清除梯度。
相关问题
allow_unreachable=True, accumulate_grad=True) # allow_unreachable flag RuntimeError: Trying to backward through the graph a second time (or directly access saved tensors after they have already been freed). Saved intermediate values of the graph are freed when you call .backward() or autograd.grad(). Specify retain_graph=True if you need to backward through the graph a second time or if you need to access saved tensors after calling backward.
allow_unreachable=True,accumulate_grad=True是深度学习中的两个参数选项。其中,allow_unreachable=True表示允许非法的操作,这主要用于避免特定环境下的错误。而accumulate_grad=True表示要对梯度进行累加操作,这通常用于多个mini-batch进行梯度下降时避免每个mini-batch更新后梯度的丢失。
RuntimeError:cuDNN error:CUDNN_STATUS_INTERNAL_ERROR LSTM batch size
根据你提供的引用内容,出现`RuntimeError: cuDNN error: CUDNN_STATUS_INTERNAL_ERROR`错误可能是由于显存不足导致的。你可以先检查一下显存使用情况,确保显存足够。
关于LSTM的batch size,LSTM是一种循环神经网络,用于处理序列数据。batch size是指每次训练时输入的样本数量。较大的batch size可以提高训练速度,但可能会占用更多的显存。较小的batch size可以节省显存,但训练速度可能会变慢。
通常情况下,选择合适的batch size需要根据具体的数据集和模型来决定。一般来说,如果显存足够,可以选择较大的batch size,例如32或64。如果显存有限,可以选择较小的batch size,例如8或16。
在PyTorch中,可以通过设置`batch_first=True`来指定输入数据的维度顺序为(batch_size, seq_length, input_size),其中`batch_size`就是指定的batch size。
下面是一个示例代码,演示了如何在PyTorch中设置LSTM的batch size为32:
```python
import torch
import torch.nn as nn
# 定义LSTM模型
class LSTMModel(nn.Module):
def __init__(self, input_size, hidden_size, num_layers):
super(LSTMModel, self).__init__()
self.hidden_size = hidden_size
self.num_layers = num_layers
self.lstm = nn.LSTM(input_size, hidden_size, num_layers, batch_first=True)
def forward(self, x):
h0 = torch.zeros(self.num_layers, x.size(0), self.hidden_size).to(x.device)
c0 = torch.zeros(self.num_layers, x.size(0), self.hidden_size).to(x.device)
out, _ = self.lstm(x, (h0, c0))
return out
# 创建输入数据
batch_size = 32
seq_length = 10
input_size = 5
input_data = torch.randn(batch_size, seq_length, input_size)
# 创建LSTM模型
hidden_size = 10
num_layers = 2
model = LSTMModel(input_size, hidden_size, num_layers)
# 前向传播
output = model(input_data)
print(output.shape) # 输出:torch.Size([32, 10, 10])
```
相关推荐
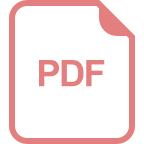
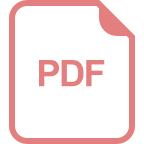
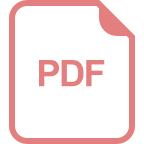












