用python先生成随机数据利用seed=207再定义函数再建立数据模型再利用训练集训练模型再使用R2对回归模型进行评价再利用python实现线性回归估计方程再可视化显示
时间: 2024-05-15 17:19:50 浏览: 8
生成随机数据:
```python
import numpy as np
np.random.seed(207) # 设置随机数种子,保证结果可重现
# 生成随机数据,x为输入,y为输出
x = np.random.rand(100, 1)
y = 2 * x + 3 + 0.2 * np.random.randn(100, 1)
```
定义函数:
```python
def linear_regression(x, y):
"""
线性回归模型
:param x: 输入,形状为(n_samples, n_features)
:param y: 输出,形状为(n_samples, 1)
:return: 模型参数,形状为(n_features + 1, 1)
"""
n_samples, n_features = x.shape
x = np.concatenate([x, np.ones((n_samples, 1))], axis=1) # 添加偏置项
# 计算模型参数
w = np.linalg.inv(x.T @ x) @ x.T @ y
return w
```
建立数据模型:
```python
w = linear_regression(x, y)
```
使用训练集训练模型:
```python
# 划分训练集和测试集
from sklearn.model_selection import train_test_split
x_train, x_test, y_train, y_test = train_test_split(x, y, test_size=0.2, random_state=42)
# 训练模型
w_train = linear_regression(x_train, y_train)
```
使用R2对回归模型进行评价:
```python
from sklearn.metrics import r2_score
y_pred = x_test @ w_train[:-1] + w_train[-1] # 预测输出
r2 = r2_score(y_test, y_pred) # 计算R2分数
print("R2 score:", r2)
```
使用python实现线性回归估计方程:
```python
print("估计方程:y = {:.2f}x + {:.2f}".format(w_train[0, 0], w_train[1, 0]))
```
可视化显示:
```python
import matplotlib.pyplot as plt
# 绘制训练集散点图
plt.scatter(x_train, y_train, color='blue', label='train data')
# 绘制拟合直线
x_min, x_max = x_train.min(), x_train.max()
y_min, y_max = x_min * w_train[0, 0] + w_train[1, 0], x_max * w_train[0, 0] + w_train[1, 0]
plt.plot([x_min, x_max], [y_min, y_max], color='red', label='fitting line')
# 添加标签和标题
plt.xlabel('x')
plt.ylabel('y')
plt.title('Linear Regression')
plt.legend()
plt.show()
```
相关推荐
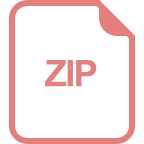
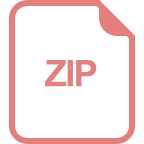
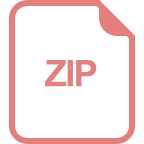













