逻辑回归python代码实现
时间: 2023-06-28 15:05:25 浏览: 128
逻辑回归是一种二分类算法,可以用于预测离散的输出结果。在Python中,我们可以使用Scikit-Learn库来实现逻辑回归。
以下是一个简单的逻辑回归的Python代码实现:
```python
import numpy as np
from sklearn.linear_model import LogisticRegression
# 定义训练数据
X_train = np.array([[1, 2], [3, 4], [5, 6], [7, 8]])
y_train = np.array([0, 0, 1, 1])
# 创建逻辑回归模型
model = LogisticRegression()
# 训练模型
model.fit(X_train, y_train)
# 定义测试数据
X_test = np.array([[2, 2], [4, 4], [6, 6], [8, 8]])
# 预测输出结果
y_pred = model.predict(X_test)
print(y_pred)
```
在这个例子中,我们首先定义了训练数据,其中X_train是一个二维数组,表示特征矩阵,y_train是一个一维数组,表示每个样本的输出结果。然后我们创建了一个逻辑回归模型,并使用fit()函数对模型进行训练。最后,我们定义了测试数据X_test,使用predict()函数对测试数据进行预测,并输出预测结果y_pred。
当然,这只是逻辑回归在Python中的基础实现,实际应用中还需要对数据进行预处理、特征工程等操作,以提高模型的准确性。
相关问题
逻辑回归python代码实现 迪哥
逻辑回归是一种机器学习算法,常用于二分类问题。下面是一个逻辑回归的Python代码实现的例子:
```python
import numpy as np
import pandas as pd
# Sigmoid函数,用于将预测结果转化为概率值
def sigmoid(z):
return 1 / (1 + np.exp(-z))
# 损失函数,用于评估模型的准确性
def cost(theta, X, y):
theta = np.matrix(theta)
X = np.matrix(X)
y = np.matrix(y)
first = np.multiply(-y, np.log(sigmoid(X * theta.T)))
second = np.multiply((1-y), np.log(1 - sigmoid(X * theta.T)))
return np.sum(first - second) / len(X)
# 梯度下降算法,用于最小化损失函数,得到最优参数
def gradientDescent(X, y, theta, alpha, iters):
temp = np.matrix(np.zeros(theta.shape))
parameters = int(theta.ravel().shape[1])
cost = np.zeros(iters)
for i in range(iters):
error = sigmoid(X * theta.T) - y
for j in range(parameters):
term = np.multiply(error, X[:,j])
temp[0,j] = theta[0,j] - (alpha / len(X)) * np.sum(term)
theta = temp
cost[i] = cost(theta, X, y)
return theta, cost
# 读取数据
data = pd.read_csv('data.csv')
# 添加一列全为1的特征列
data.insert(0, 'Ones', 1)
# 将数据转化为矩阵
cols = data.shape[1]
X = data.iloc[:,0:cols-1]
y = data.iloc[:,cols-1:cols]
X = np.matrix(X.values)
y = np.matrix(y.values)
theta = np.zeros([1,3])
# 设置学习率和迭代次数
alpha = 0.01
iters = 1000
# 执行梯度下降算法,得到最优参数
theta, cost = gradientDescent(X, y, theta, alpha, iters)
# 输出最优参数和损失函数值
print("最优参数:", theta)
print("损失函数值:", cost[-1])
```
贝叶斯参数优化逻辑回归Python代码实现
贝叶斯参数优化逻辑回归是一种使用贝叶斯优化算法来寻找逻辑回归模型最佳参数的方法。下面是一个简单的Python代码实现:
```python
import numpy as np
from sklearn.linear_model import LogisticRegression
from skopt import BayesSearchCV
# 准备数据
X = np.array([[1, 2], [3, 4], [5, 6], [7, 8]])
y = np.array([0, 0, 1, 1])
# 定义逻辑回归模型
model = LogisticRegression()
# 定义参数搜索空间
param_space = {
'C': (0.1, 10.0, 'log-uniform'),
'penalty': ['l1', 'l2'],
'fit_intercept': [True, False],
'solver': ['liblinear', 'saga']
}
# 使用贝叶斯参数优化进行参数搜索
opt_model = BayesSearchCV(model, param_space, n_iter=50, cv=3)
opt_model.fit(X, y)
# 输出最佳参数和得分
print("Best parameters found: ", opt_model.best_params_)
print("Best score found: ", opt_model.best_score_)
```
这段代码使用了`sklearn`库中的`LogisticRegression`类作为逻辑回归模型,并使用`skopt`库中的`BayesSearchCV`类进行贝叶斯参数优化。首先,我们准备了输入特征矩阵`X`和目标变量数组`y`。然后,定义了逻辑回归模型`model`和参数搜索空间`param_space`。最后,使用`BayesSearchCV`进行参数搜索,并输出最佳参数和得分。
阅读全文
相关推荐
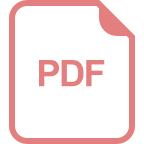
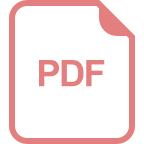











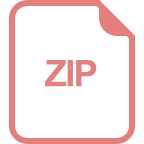