self.net = nn.Sequential( nn.Linear(input_dim, 256), nn.ReLU(), nn.Linear(256, 128), nn.ReLU(), nn.Linear(128, 64), nn.ReLU(), nn.Linear(64, 1), )
时间: 2024-04-20 08:23:34 浏览: 182
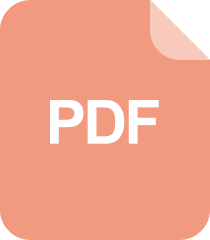
对Pytorch中nn.ModuleList 和 nn.Sequential详解
您给出的代码定义了一个神经网络的结构。这个网络有4个线性层(linear layers),每个线性层后面都跟着一个ReLU激活函数(rectified linear unit)。
输入层的维度是input_dim,输出层的维度是1。其中,第一个线性层的输入维度是input_dim,输出维度是256。第二个线性层的输入维度是256,输出维度是128。第三个线性层的输入维度是128,输出维度是64。第四个线性层的输入维度是64,输出维度是1。
这个网络的作用是将输入数据通过一系列线性变换和非线性变换映射到一个单一的输出值。每个线性层都会将输入数据进行线性变换,而ReLU激活函数则会引入非线性,使得网络能够学习更加复杂的模式和特征。
希望这个回答能帮到您!如果您有任何其他问题,请随时提问。
阅读全文
相关推荐
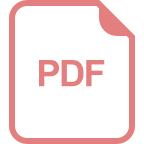
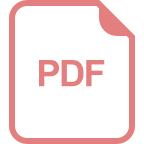















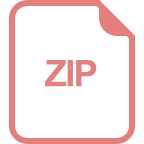