Duplicate weights for temporal attention and temporal norm # new_state_dict = state_dict.copy() # for key in state_dict: # if 'blocks' in key and 'attn' in key and 'relative' not in key and 'mask' not in key: # new_key = key.replace('attn','temporal_attn') # if not new_key in state_dict: # new_state_dict[new_key] = state_dict[key] # else: # new_state_dict[new_key] = state_dict[new_key] # if 'blocks' in key and 'norm1' in key and 'relative' not in key and 'mask' not in key: # new_key = key.replace('norm1','temporal_norm') # if not new_key in state_dict: # new_state_dict[new_key] = state_dict[key] # else: # new_state_dict[new_key] = state_dict[new_key] # state_dict = new_state_dict
时间: 2023-06-19 17:06:44 浏览: 175
这段代码是在加载预训练模型的过程中进行的一些操作,主要是为了避免在模型中出现重复的权重。在这段代码中,首先将原始的权重字典进行复制,然后通过遍历原始字典中的键,将其中与时间相关的注意力和归一化操作的键名进行修改,修改后的键名中将'attn'替换为'temporal_attn',将'norm1'替换为'temporal_norm'。如果修改后的键名不在新的字典中,则将其添加到新字典中;否则,将其权重值更新为新的字典中已有的键的权重值。这样就保证了模型中不会出现重复的权重。
相关问题
if isinstance(self.pretrained, str): self.apply(_init_weights) logger = get_root_logger() logger.info(f'load model from: {self.pretrained}') checkpoint = torch.load(self.pretrained, map_location='cpu') state_dict = checkpoint['model'] state_dict['patch_embed.proj.weight'] = state_dict['patch_embed.proj.weight'].unsqueeze(2).repeat(1,1,self.patch_size[0],1,1) / self.patch_size[0] ## Duplicate weights for temporal attention and temporal norm # new_state_dict = state_dict.copy() # for key in state_dict: # if 'blocks' in key and 'attn' in key and 'relative' not in key and 'mask' not in key: # new_key = key.replace('attn','temporal_attn') # if not new_key in state_dict: # new_state_dict[new_key] = state_dict[key] # else: # new_state_dict[new_key] = state_dict[new_key] # if 'blocks' in key and 'norm1' in key and 'relative' not in key and 'mask' not in key: # new_key = key.replace('norm1','temporal_norm') # if not new_key in state_dict: # new_state_dict[new_key] = state_dict[key] # else: # new_state_dict[new_key] = state_dict[new_key] # state_dict = new_state_dict msg = self.load_state_dict(state_dict, strict=False) logger.info('Missing keys: {}'.format(msg.missing_keys)) logger.info('Unexpected keys: {}'.format(msg.unexpected_keys)) logger.info(f"=> loaded successfully '{self.pretrained}'") del checkpoint torch.cuda.empty_cache() elif self.pretrained is None: self.apply(_init_weights) else: raise TypeError('pretrained must be a str or None')
这段代码是初始化模型的代码。首先判断是否需要加载预训练模型,如果需要,则从指定的路径加载预训练模型的参数,并将模型的 patch_embed.proj.weight 层的权重进行重新计算,以适应输入的 patch 大小。然后将加载的参数应用到当前模型中。如果没有指定预训练模型,则直接进行权重初始化。如果预训练参数既不是字符串也不是 None,则会报错。
高通Linux内核sn65dsi84芯片驱动定义了这个结构体:static const struct drm_bridge_funcs sn65dsi83_funcs = { .attach = sn65dsi83_attach, .atomic_pre_enable = sn65dsi83_atomic_pre_enable, .atomic_enable = sn65dsi83_atomic_enable, .atomic_disable = sn65dsi83_atomic_disable, .atomic_post_disable = sn65dsi83_atomic_post_disable, .mode_valid = sn65dsi83_mode_valid, .atomic_duplicate_state = drm_atomic_helper_bridge_duplicate_state, .atomic_destroy_state = drm_atomic_helper_bridge_destroy_state, .atomic_reset = drm_atomic_helper_bridge_reset, .atomic_get_input_bus_fmts = sn65dsi83_atomic_get_input_bus_fmts, };它的执行顺序是什么
这段代码是定义了一个名为sn65dsi83_funcs的静态常量结构体,其中包含了一些函数指针,这些函数指针指向了具体的函数实现。这些函数指针按照定义顺序依次对应以下函数:attach、atomic_pre_enable、atomic_enable、atomic_disable、atomic_post_disable、mode_valid、atomic_duplicate_state、atomic_destroy_state、atomic_reset以及atomic_get_input_bus_fmts。在实际使用中,这些函数可能会被驱动程序中的其他函数调用,以完成具体的功能。
阅读全文
相关推荐
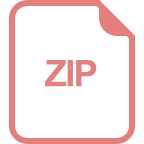
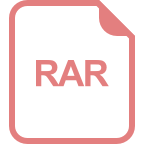
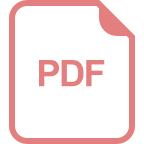













