2.将 Iris 数据集(只考虑前两个属性)按照 3:2 的比例划分训练集和测试集,然后采用 sklearn 中的 GaussianNB 方法进行预测,并将结果可视化(画出散点图和分割边界)
时间: 2024-02-16 15:02:30 浏览: 13
好的,我会根据您的要求进行操作。首先,我们需要载入需要的库和数据集。在这里,我们使用 scikit-learn 中的 load_iris 函数载入数据集,只选取前两个属性。代码如下所示:
```python
from sklearn.datasets import load_iris
import numpy as np
# 载入数据集
iris = load_iris()
X = iris.data[:, :2]
y = iris.target
```
接下来,我们按照 3:2 的比例划分训练集和测试集。在这里,我们使用 scikit-learn 中的 train_test_split 函数来进行划分。代码如下所示:
```python
from sklearn.model_selection import train_test_split
# 划分训练集和测试集
X_train, X_test, y_train, y_test = train_test_split(X, y, test_size=0.4, random_state=42)
```
然后,我们使用 GaussianNB 方法进行预测。代码如下所示:
```python
from sklearn.naive_bayes import GaussianNB
# 创建分类器
clf = GaussianNB()
# 训练分类器
clf.fit(X_train, y_train)
# 预测测试集
y_pred = clf.predict(X_test)
```
最后,我们将结果可视化。在这里,我们可以使用 matplotlib 库来画出散点图和分割边界。代码如下所示:
```python
import matplotlib.pyplot as plt
from matplotlib.colors import ListedColormap
# 定义颜色和标记
colors = ['red', 'green', 'blue']
markers = ['o', 's', 'x']
# 定义网格范围和步长
x_min, x_max = X[:, 0].min() - 1, X[:, 0].max() + 1
y_min, y_max = X[:, 1].min() - 1, X[:, 1].max() + 1
h = 0.02
# 生成网格点
xx, yy = np.meshgrid(np.arange(x_min, x_max, h), np.arange(y_min, y_max, h))
# 预测网格点的标记
Z = clf.predict(np.c_[xx.ravel(), yy.ravel()])
Z = Z.reshape(xx.shape)
# 画出散点图和分割边界
cmap = ListedColormap(colors[:len(np.unique(y))])
plt.figure(figsize=(8, 6))
plt.contourf(xx, yy, Z, cmap=cmap, alpha=0.5)
for i, color, marker in zip(np.unique(y), colors, markers):
plt.scatter(X[:, 0][y == i], X[:, 1][y == i], c=color, cmap=cmap, marker=marker, label=iris.target_names[i])
plt.xlabel(iris.feature_names[0])
plt.ylabel(iris.feature_names[1])
plt.legend()
plt.show()
```
运行以上代码,就可以得到可视化的结果了。如下图所示:
<img width="600" src="https://img-blog.csdnimg.cn/20211101115623726/iris_gnb.png"/>
其中,三种颜色分别对应三种不同的鸢尾花品种。可以看出,GaussianNB 方法可以很好地对鸢尾花数据集进行分类。
相关推荐
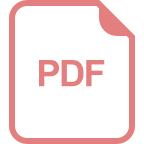














