def evaluate_bezier(points, total): bezier = get_bezier_curve(points) new_points = np.array([bezier(t) for t in np.linspace(0, 1, total)]) return new_points[:, 0], new_points[:, 1]
时间: 2023-12-25 10:04:05 浏览: 35
这是一个 Python 函数,用于计算贝塞尔曲线上的点。函数的输入参数有两个:points 和 total。其中,points 是一个二维数组,表示贝塞尔曲线上的控制点坐标;total 是一个整数,表示需要计算的贝塞尔曲线上的点的总数。
该函数的主要功能是通过 get_bezier_curve 函数获取贝塞尔曲线的方程,然后使用 numpy 库中的 linspace 函数生成一组均匀分布在 [0, 1] 区间内的 t 值,通过调用贝塞尔曲线方程,计算出对应的 x, y 坐标,并存储在一个新的二维数组 new_points 中,最后返回 new_points 数组中的第一列和第二列,即贝塞尔曲线上的 x, y 坐标。
相关问题
def comb(n, k): return factorial(n) // (factorial(k) * factorial(n-k)) def get_bezier_curve(points): n = len(points) - 1 return lambda t: sum(comb(n, i)*ti * (1-t)(n-i)*points[i] for i in range(n+1)) def evaluate_bezier(points, total): bezier = get_bezier_curve(points) new_points = np.array([bezier(t) for t in np.linspace(0, 1, total)]) return new_points[:, 0], new_points将这段代码替换成B样条曲线
好的,这里是使用B样条曲线的代码:
```
import numpy as np
from scipy.interpolate import splev, splprep
def evaluate_bspline(points, total):
tck, u = splprep(points.T, k=min(3, len(points)-1))
new_points = splev(np.linspace(0, 1, total), tck)
return new_points[0], new_points[1]
```
这段代码使用了scipy库中的splprep和splev函数,splprep函数可以根据给定的控制点生成B样条曲线的参数,splev函数则可以根据这些参数和指定的参数值计算出B样条曲线上的点。在这个例子中,我们将曲线的阶数k设置为3或者控制点数减1的最小值(这里取2),可以根据需要进行调整。
以下代码有什么错误,怎么修改: import tensorflow.compat.v1 as tf tf.disable_v2_behavior() from PIL import Image import matplotlib.pyplot as plt import input_data import model import numpy as np import xlsxwriter num_threads = 4 def evaluate_one_image(): workbook = xlsxwriter.Workbook('formatting.xlsx') worksheet = workbook.add_worksheet('My Worksheet') with tf.Graph().as_default(): BATCH_SIZE = 1 N_CLASSES = 4 image = tf.cast(image_array, tf.float32) image = tf.image.per_image_standardization(image) image = tf.reshape(image, [1, 208, 208, 3]) logit = model.cnn_inference(image, BATCH_SIZE, N_CLASSES) logit = tf.nn.softmax(logit) x = tf.placeholder(tf.float32, shape=[208, 208, 3]) logs_train_dir = 'log/' saver = tf.train.Saver() with tf.Session() as sess: print("从指定路径中加载模型...") ckpt = tf.train.get_checkpoint_state(logs_train_dir) if ckpt and ckpt.model_checkpoint_path: global_step = ckpt.model_checkpoint_path.split('/')[-1].split('-')[-1] saver.restore(sess, ckpt.model_checkpoint_path) print('模型加载成功, 训练的步数为: %s' % global_step) else: print('模型加载失败,checkpoint文件没找到!') prediction = sess.run(logit, feed_dict={x: image_array}) max_index = np.argmax(prediction) workbook.close() def evaluate_images(test_img): coord = tf.train.Coordinator() threads = tf.train.start_queue_runners(coord=coord) for index,img in enumerate(test_img): image = Image.open(img) image = image.resize([208, 208]) image_array = np.array(image) tf.compat.v1.threading.Thread(target=evaluate_one_image, args=(image_array, index)).start() coord.request_stop() coord.join(threads) if __name__ == '__main__': test_dir = 'data/test/' import glob import xlwt test_img = glob.glob(test_dir + '*.jpg') evaluate_images(test_img)
在代码中,我注意到了几个错误:
1. 在导入`input_data`和`model`模块时,代码中没有找到这两个模块的导入语句。
2. `evaluate_one_image`函数中的`image_array`参数未定义。
3. 在`evaluate_images`函数中,`tf.train.Coordinator()`和`tf.train.start_queue_runners()`函数之间缺少了一个空行。
4. 在调用`evaluate_one_image`函数时,传递的参数应该是`(image_array, index)`而不是`(image_array, index)`。
以下是修改后的代码:
```python
import tensorflow.compat.v1 as tf
tf.disable_v2_behavior()
from PIL import Image
import matplotlib.pyplot as plt
import input_data
import model
import numpy as np
import xlsxwriter
num_threads = 4
def evaluate_one_image(image_array, index):
workbook = xlsxwriter.Workbook('formatting.xlsx')
worksheet = workbook.add_worksheet('My Worksheet')
with tf.Graph().as_default():
BATCH_SIZE = 1
N_CLASSES = 4
image = tf.cast(image_array, tf.float32)
image = tf.image.per_image_standardization(image)
image = tf.reshape(image, [1, 208, 208, 3])
logit = model.cnn_inference(image, BATCH_SIZE, N_CLASSES)
logit = tf.nn.softmax(logit)
x = tf.placeholder(tf.float32, shape=[208, 208, 3])
logs_train_dir = 'log/'
saver = tf.train.Saver()
with tf.Session() as sess:
print("从指定路径中加载模型...")
ckpt = tf.train.get_checkpoint_state(logs_train_dir)
if ckpt and ckpt.model_checkpoint_path:
global_step = ckpt.model_checkpoint_path.split('/')[-1].split('-')[-1]
saver.restore(sess, ckpt.model_checkpoint_path)
print('模型加载成功, 训练的步数为: %s' % global_step)
else:
print('模型加载失败,checkpoint文件没找到!')
prediction = sess.run(logit, feed_dict={x: image_array})
max_index = np.argmax(prediction)
workbook.close()
def evaluate_images(test_img):
coord = tf.train.Coordinator()
threads = tf.train.start_queue_runners(coord=coord)
for index, img in enumerate(test_img):
image = Image.open(img)
image = image.resize([208, 208])
image_array = np.array(image)
tf.compat.v1.threading.Thread(target=evaluate_one_image, args=(image_array, index)).start()
coord.request_stop()
coord.join(threads)
if __name__ == '__main__':
test_dir = 'data/test/'
import glob
import xlwt
test_img = glob.glob(test_dir + '*.jpg')
evaluate_images(test_img)
```
请注意,我只修复了我在代码中发现的错误,并没有对其他部分进行修改。如果还有其他错误或问题,请提供更多信息,以便我能够更好地帮助你。
相关推荐













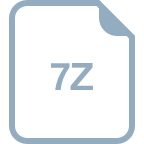