new_param[i.replace('student.', '')] =ckpt[i]
时间: 2024-01-24 09:05:12 浏览: 14
This line of code takes each key in the dictionary 'ckpt' that starts with the string 'student.' and removes that string from the key using the string method 'replace()'. The resulting key is then added to a new dictionary called 'new_param' with the corresponding value from 'ckpt'.
For example, if 'ckpt' had a key-value pair of 'student.name': 'John', this line of code would add a new key-value pair to 'new_param' of 'name': 'John'. This allows for easier access to the values in 'ckpt' without needing to include the 'student.' string in the key every time.
相关问题
解释代码:new_param[i.replace('student.', '')] =ckpt[i]
这段代码的功能是将一个字典ckpt中的键值对以新的键添加到另一个字典new_param中。
具体来说,代码首先遍历ckpt字典的所有键,使用replace方法将其中的'student.'替换为空字符串,得到新的键名。然后将新的键名和对应的值ckpt[i]以键值对的形式添加到new_param字典中。
换句话说,这段代码的作用是将ckpt字典中以'student.'开头的键名去掉前缀后,添加到new_param字典中,以构造一个新的字典。
case PM660_SUBTYPE: chip->chg.chg_param.smb_version = PM660_SUBTYPE; chip->chg.wa_flags |= BOOST_BACK_WA | OTG_WA | OV_IRQ_WA_BIT | TYPEC_PBS_WA_BIT; chg->param.freq_buck = pm660_params.freq_buck; chg->param.freq_boost = pm660_params.freq_boost; chg->chg_freq.freq_5V = 650; chg->chg_freq.freq_6V_8V = 850; chg->chg_freq.freq_9V = 1050; chg->chg_freq.freq_12V = 1200; chg->chg_freq.freq_removal = 1050; chg->chg_freq.freq_below_otg_threshold = 1600; chg->chg_freq.freq_above_otg_threshold = 800; break;代码分析
这段代码是在处理 PM660 芯片类型的充电参数。具体来说,它完成了以下操作:
1. 将 SMB 版本号设置为 PM660_SUBTYPE。
2. 开启一些工作模式和异常保护标志,包括 BOOST_BACK_WA、OTG_WA、OV_IRQ_WA_BIT 和 TYPEC_PBS_WA_BIT。
3. 设置一些频率参数,包括充电时的不同电压下的频率(chg_freq.freq_5V、chg_freq.freq_6V_8V、chg_freq.freq_9V、chg_freq.freq_12V),以及移除充电器时的频率(chg_freq.freq_removal)等。
4. 设置 chg_param.freq_buck 和 chg_param.freq_boost 参数,这些参数在 PM660 芯片类型中都是固定的。
这些参数的设置将影响 PM660 芯片的充电行为,从而保证充电的效率和安全性。
相关推荐
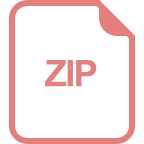













