tensorflow2.0怎么加载预训练权重文件
时间: 2023-09-21 12:11:02 浏览: 226
在 Tensorflow 2.0 中,可以使用 `tf.keras.models.load_model()` 函数来加载预训练权重文件。具体步骤如下:
1. 安装相应的预训练模型,例如 ResNet50,可以使用以下命令:
```python
!pip install tensorflow==2.0.0
!pip install tensorflow_hub==0.7.0
!pip install tensorflow_datasets==2.0.0
!pip install tensorflow_probability==0.8.0
```
2. 导入相应的模型和预处理函数:
```python
import tensorflow as tf
import tensorflow_hub as hub
import tensorflow_datasets as tfds
# 加载 ResNet50 模型
model = tf.keras.applications.ResNet50(weights='imagenet')
# 加载预处理函数
preprocess_input = tf.keras.applications.resnet50.preprocess_input
```
3. 使用 `tf.keras.models.load_model()` 函数加载预训练权重文件:
```python
# 加载预训练权重文件
model.load_weights('path/to/weights.h5')
```
4. 对输入数据进行预处理,并使用加载的模型进行预测:
```python
# 加载测试数据
test_data, test_info = tfds.load('cats_vs_dogs', split='test', with_info=True)
# 对测试数据进行预处理
test_data = test_data.map(lambda x: (preprocess_input(tf.image.resize(x['image'], (224, 224))), x['label']))
test_data = test_data.batch(32)
# 使用加载的模型进行预测
results = model.predict(test_data)
```
其中,`path/to/weights.h5` 是预训练权重文件的路径。在加载预训练权重文件之前,需要先加载相应的模型,以确保预训练权重文件与模型的结构相同。在预测时,需要对输入数据进行预处理,以符合模型的输入要求。
阅读全文
相关推荐
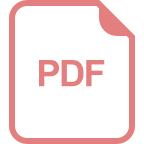
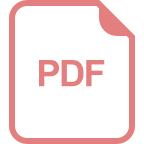
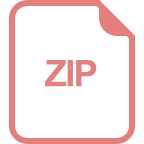
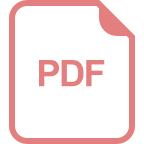
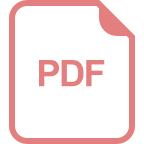
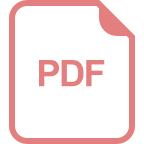
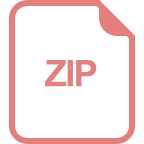
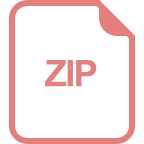
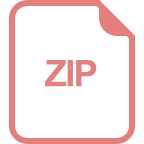
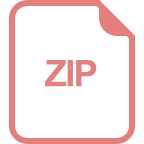
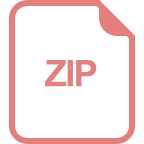
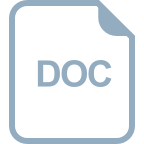
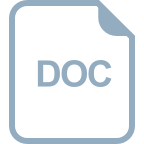





