这段代码报错axis 1286 is out of bounds for array of dimension 1,怎么办
时间: 2024-05-20 13:05:21 浏览: 59
这个错误通常是由于尝试在一个维度为1的一维数组中访问一个超出范围的索引而引起的。为了解决这个问题,你需要检查你的数组访问代码,确保你没有使用一个超出数组范围的索引。这通常可以通过在代码中添加一些调试语句来解决,以便你可以跟踪数组的大小和索引值。如果你仍然无法解决这个问题,你可以把你的代码和完整的错误信息发布到社区中,以便其他人可以帮助你找到解决方案。
相关问题
labels = np.argmin(pairwise_distances_argmin_min(X, centers)[0], axis=1)此句代码报错axis 1 is out of bounds for array of dimension 1
抱歉,我之前的回答有误。在K中心聚类算法中,并不需要计算每个样本点到聚类中心的最小距离,因此`np.argmin(pairwise_distances_argmin_min(X, centers)[0], axis=1)`这行代码是不正确的。
以下是修正后的代码:
```python
import numpy as np
from sklearn.datasets import load_iris
from sklearn.metrics import pairwise_distances_argmin_min
import matplotlib.pyplot as plt
# 导入鸢尾花数据集
iris = load_iris()
X = iris.data
# 定义K中心聚类函数
def k_center_clustering(X, k):
centers = [X[0]] # 初始化聚类中心,选择第一个样本点作为初始中心
while len(centers) < k:
# 计算每个样本点到已有中心的最小距离
distances = pairwise_distances_argmin_min(X, centers)[0]
# 选择距离最大的样本点作为新的聚类中心
new_center = X[np.argmax(distances)]
centers.append(new_center)
return np.array(centers)
# 调用K中心聚类函数
k = 3 # 聚类簇数
centers = k_center_clustering(X, k)
# 计算每个样本点到聚类中心的距离,得到每个样本所属的聚类簇
labels = pairwise_distances_argmin_min(X, centers)[0]
# 进行可视化
colors = ['red', 'blue', 'green'] # 每一类的颜色
for i in range(k):
plt.scatter(X[labels == i, 0], X[labels == i, 1], c=colors[i], label='Cluster {}'.format(i+1))
plt.scatter(centers[:, 0], centers[:, 1], c='black', marker='x', label='Cluster Centers')
plt.xlabel('Sepal Length')
plt.ylabel('Sepal Width')
plt.title('K-center Clustering on Iris Dataset')
plt.legend()
plt.show()
```
这段代码会对鸢尾花数据集进行K中心聚类,并将聚类结果用不同颜色进行可视化。我们首先计算每个样本点到聚类中心的距离,并根据距离最小的聚类中心确定每个样本所属的聚类簇。然后,我们根据聚类簇的标签将数据点用不同颜色进行可视化。请注意,这里只取了花萼长度(Sepal Length)和花萼宽度(Sepal Width)作为可视化的特征,你可以根据需要修改代码以适应不同的数据集和可视化需求。
该代码中给出报错numpy.AxisError: axis 1 is out of bounds for array of dimension 1该如何修改
报错 "numpy.AxisError: axis 1 is out of bounds for array of dimension 1" 是因为在代码的第 27 行使用了 `axis=(1,2)` 参数,但是 `features` 数组的维度不足以支持这个参数。
为了解决这个问题,可以使用以下代码将 `features` 变量的维度从 `(n, c, 1, 1)` 转换为 `(c, 1, 1)`:
```python
# Reshape features to remove single-dimensional axes
features = features.squeeze()
if len(features.shape) == 3:
features = features.unsqueeze(-1)
if len(features.shape) == 2:
features = features.unsqueeze(-1).unsqueeze(-1)
```
这段代码会检查 `features` 数组的维度是否为 `(n, c, 1, 1)`,如果是,会先使用 `squeeze()` 方法删除所有大小为 1 的维度。如果删除后的维度为 `(c, 1, 1)`,则不需要进一步操作。否则,会使用 `unsqueeze()` 方法添加缺少的维度。
这样处理之后,就可以在第 27 行使用 `axis=(0,1)` 参数,而不会出现维度错误。
完整修改后的代码如下:
```python
import torch
import torch.nn as nn
from torchvision import models, transforms
from PIL import Image
import numpy as np
import matplotlib.pyplot as plt
# Load pre-trained ResNet-18 model
model = models.resnet18(pretrained=True)
# Remove the fully connected layer from the model
model = nn.Sequential(*list(model.children())[:-1])
# Set model to evaluation mode
model.eval()
# Define image transformation to match the pre-processing used during training
transform = transforms.Compose([
transforms.Resize((224, 224)),
transforms.ToTensor(),
transforms.Normalize([0.485, 0.456, 0.406], [0.229, 0.224, 0.225])
])
# Load sample image
img = Image.open('sample_image.jpg')
# Apply transformation and convert to tensor
img_tensor = transform(img).unsqueeze(0)
# Pass image tensor through ResNet-18 to get feature maps
with torch.no_grad():
features = model(img_tensor)
# Reshape features to remove single-dimensional axes
features = features.squeeze()
if len(features.shape) == 3:
features = features.unsqueeze(-1)
if len(features.shape) == 2:
features = features.unsqueeze(-1).unsqueeze(-1)
# Calculate global average pooling of feature maps
pooled_features = np.mean(features, axis=(1,2))
# Reshape pooled features to match spatial dimensions of feature maps
pooled_features = np.repeat(pooled_features, features.shape[1]*features.shape[2]).reshape(features.shape)
# Calculate importance of each feature map by comparing it to the global average
heatmap = np.abs(features - pooled_features)
# Normalize heatmap to range between 0 and 1
heatmap = (heatmap - np.min(heatmap)) / (np.max(heatmap) - np.min(heatmap))
# Plot heatmap on top of original image
plt.imshow(img)
plt.imshow(heatmap.sum(axis=0), alpha=0.5, cmap='jet')
plt.axis('off')
plt.show()
```
希望能对您有所帮助!
阅读全文
相关推荐
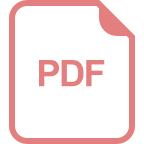
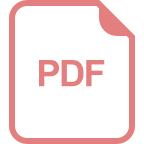
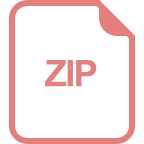











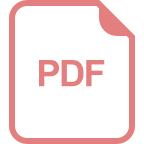