运行上面的代码出现RuntimeError: stack expects each tensor to be equal size, but got [3, 200, 149] at entry 0 and [3, 149, 200] at entry 2
时间: 2023-12-24 16:02:35 浏览: 37
这个错误是由于输入到模型中的张量大小不一致导致的。可以通过调整输入张量的大小来解决这个问题。具体来说,可以在输入数据时进行padding,使得每个batch中的张量大小一致。如果你使用的是PyTorch,可以使用torch.nn.utils.rnn.pad_sequence()函数对输入数据进行padding。例如,假设你有一个大小为(3, 200, 149)的张量,你可以使用以下代码进行padding:
```python
import torch.nn.utils.rnn as rnn_utils
# 假设你的张量名为tensor
tensor_padded = rnn_utils.pad_sequence([tensor[i] for i in range(tensor.shape[0])], batch_first=True)
```
这样就可以得到一个大小为(3, 200, 200)的张量,然后再将其输入到模型中即可。
相关问题
RuntimeError: stack expects each tensor to be equal size, but got [96, 3] at entry 0 and [64, 3] at entry 17
这个错误通常发生在使用堆栈函数(如torch.stack)时,由于输入张量的大小不同而导致无法堆叠。在您的情况下,输入张量的大小分别为[96, 3]和[64, 3],它们的第二维不同,因此无法堆叠。
您需要确保所有输入张量的形状都相同,或者根据您的需求调整它们的形状,以使它们具有相同的大小。您可以使用函数如torch.reshape或torch.unsqueeze来改变张量的形状。
RuntimeError: stack expects each tensor to be equal size, but got [0, 26] at entry 0 and [1, 26] at entry 1
这个错误通常是由于输入的张量形状不一致导致的。`torch.stack`函数要求输入的张量具有相同的形状,以便能够沿着指定的维度进行堆叠。
在您的情况下,错误信息显示了两个不同形状的张量:一个形状为 `[0, 26]` 的张量和一个形状为 `[1, 26]` 的张量。这意味着在堆叠这两个张量时,形状不匹配,因此引发了错误。
要解决这个问题,您需要确保在进行`torch.stack`操作之前,所有的输入张量具有相同的形状。您可以使用`torch.unsqueeze`函数在维度上扩展其中一个张量,使其与另一个张量的形状匹配,然后再进行堆叠。
以下是一个示例:
```python
import torch
# 假设有两个张量 tensor1 和 tensor2
tensor1 = torch.tensor([1, 2, 3])
tensor2 = torch.tensor([4, 5, 6])
# 在维度0上扩展 tensor1 的形状,使其与 tensor2 的形状匹配
tensor1 = torch.unsqueeze(tensor1, 0)
# 进行堆叠操作
stacked_tensor = torch.stack([tensor1, tensor2], dim=0)
```
在上述示例中,我们使用`torch.unsqueeze`函数在维度0上扩展了`tensor1`的形状,使其成为形状为 `[1, 3]` 的张量。然后,我们可以使用`torch.stack`将两个形状相同的张量堆叠在一起。
确保在使用`torch.stack`函数之前,所有输入的张量具有相同的形状,这样就可以避免出现错误。
相关推荐
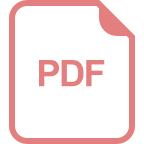
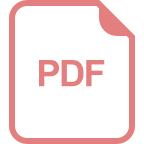
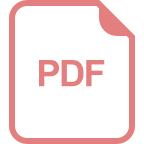












