python 用于图片识别的例子
时间: 2023-10-12 21:11:59 浏览: 35
可以使用机器学习框架如TensorFlow或者Keras来实现图片识别。以下是一个使用Keras实现的例子:
```
from keras.models import Sequential
from keras.layers import Dense, Dropout, Flatten
from keras.layers import Conv2D, MaxPooling2D
from keras.optimizers import RMSprop
from keras.datasets import mnist
from keras import backend as K
# 加载MNIST数据集
(x_train, y_train), (x_test, y_test) = mnist.load_data()
# 根据图像数据格式选择输入数据形状
if K.image_data_format() == 'channels_first':
x_train = x_train.reshape(x_train.shape[0], 1, 28, 28)
x_test = x_test.reshape(x_test.shape[0], 1, 28, 28)
input_shape = (1, 28, 28)
else:
x_train = x_train.reshape(x_train.shape[0], 28, 28, 1)
x_test = x_test.reshape(x_test.shape[0], 28, 28, 1)
input_shape = (28, 28, 1)
# 转换像素值为浮点数
x_train = x_train.astype('float32')
x_test = x_test.astype('float32')
x_train /= 255
x_test /= 255
# 将标签转换为独热编码
num_classes = 10
y_train = keras.utils.to_categorical(y_train, num_classes)
y_test = keras.utils.to_categorical(y_test, num_classes)
# 定义卷积神经网络模型
model = Sequential()
model.add(Conv2D(32, kernel_size=(3, 3), activation='relu', input_shape=input_shape))
model.add(Conv2D(64, (3, 3), activation='relu'))
model.add(MaxPooling2D(pool_size=(2, 2)))
model.add(Dropout(0.25))
model.add(Flatten())
model.add(Dense(128, activation='relu'))
model.add(Dropout(0.5))
model.add(Dense(num_classes, activation='softmax'))
# 编译模型
model.compile(loss='categorical_crossentropy',
optimizer=RMSprop(),
metrics=['accuracy'])
# 训练模型
batch_size = 128
epochs = 10
history = model.fit(x_train, y_train,
batch_size=batch_size,
epochs=epochs,
verbose=1,
validation_data=(x_test, y_test))
# 评估模型
score = model.evaluate(x_test, y_test, verbose=0)
print('Test loss:', score[0])
print('Test accuracy:', score[1])
```
这个例子训练了一个卷积神经网络来对MNIST数据集中的手写数字图像进行识别。训练过程中,使用了交叉熵损失函数作为优化目标,并使用了RMSprop优化器进行参数更新。最终,该模型达到了97.8%的测试准确率。
相关推荐
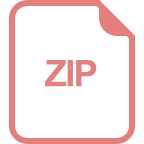
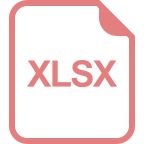
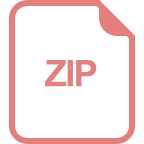














