解释x = Convolution2D(32, 3, 3, activation= relu , padding= same , name= block1_conv1 )(img_input)
时间: 2023-11-01 14:06:56 浏览: 198
这段代码使用 Keras 框架中提供的 Convolution2D 层对输入的图像进行卷积操作。具体来说,它将输入的 img_input 数据通过一个卷积核(kernel)进行卷积,得到一个新的特征图(feature map)。其中参数说明如下:
- 32:卷积核的数量,即输出的特征图个数。
- 3, 3:卷积核的大小,即卷积窗口的高度和宽度,这里为 3x3。
- activation=relu:激活函数,这里使用 ReLU 激活函数。
- padding=same:边缘填充方式,这里使用同样的填充方式,即在图像的边缘进行填充,使得卷积后的特征图大小不变。
- name=block1_conv1:该层的名称,用于在后续代码中引用该层。
相关问题
解释from keras.layers import Input, Conv2D, BatchNormalization, Activation, Addfrom keras.models import Modeldef res_block(inputs, filters, kernel_size=3, strides=1, padding='same'): x = Conv2D(filters, kernel_size, strides=strides, padding=padding)(inputs) x = BatchNormalization()(x) x = Activation('relu')(x) x = Conv2D(filters, kernel_size, strides=1, padding=padding)(x) x = BatchNormalization()(x) x = Add()([x, inputs]) x = Activation('relu')(x) return xinput_shape = (224, 224, 3)input1 = Input(input_shape)input2 = Input(input_shape)input3 = Input(input_shape)x = Conv2D(64, 7, strides=2, padding='same')(input1)x = BatchNormalization()(x)x = Activation('relu')(x)x = res_block(x, 64)x = res_block(x, 64)x = Conv2D(128, 3, strides=2, padding='same')(x)x = BatchNormalization()(x)x = Activation('relu')(x)x = res_block(x, 128)x = res_block(x, 128)x = Conv2D(256, 3, strides=2, padding='same')(x)x = BatchNormalization()(x)x = Activation('relu')(x)x = res_block(x, 256)x = res_block(x, 256)x = Conv2D(512, 3, strides=2, padding='same')(x)x = BatchNormalization()(x)x = Activation('relu')(x)x = res_block(x, 512)x = res_block(x, 512)x1 = Conv2D(1024, 3, strides=2, padding='same')(x)x1 = BatchNormalization()(x1)x1 = Activation('relu')(x1)x1 = res_block(x1, 1024)x1 = res_block(x1, 1024)x1 = Conv2D(2048, 3, strides=2, padding='same')(x1)x1 = BatchNormalization()(x1)x1 = Activation('relu')(x1)x1 = res_block(x1, 2048)x1 = res_block(x1, 2048)output1 = x1x2 = Conv2D(1024, 3, strides=2, padding='same')(x)x2 = BatchNormalization()(x2)x2 = Activation('relu')(x2)x2 = res_block(x2, 1024)x2 = res_block(x2, 1024)x2 = Conv2D(2048, 3, strides=2, padding='same')(x2)x2 = BatchNormalization()(x2)x2 = Activation('relu')(x2)x2 = res_block(x2, 2048)x2 = res_block(x2, 2048)output2 = x2x3 = Conv2D(1024, 3, strides=2, padding='same')(x)x3 = BatchNormalization()(x3)x3 = Activation('relu')(x3)x3 = res_block(x3, 1024)x3 = res_block(x3, 1024)x3 = Conv2D(2048, 3, strides=2, padding='same')(x3)x3 = BatchNormalization()(x3)x3 = Activation('relu')(x3)x3 = res_block(x3, 2048)x3 = res_block(x3, 2048)output3 = x3model = Model(inputs=[input1, input2, input3], outputs=[output1, output2, output3])
这是一个基于 Keras 搭建的 ResNet 网络模型,包含了多个 Residual Block。其中每个 Residual Block 内部的结构都相似,由两个 Convolution 层和两个 Batch Normalization 层组成,中间还包含了残差连接和 ReLU 激活函数,可以有效防止梯度消失和梯度爆炸问题。此外,该模型还包括了多个输出,并可以接收三个输入。
def block1(x, filters, kernel_size=3, stride=1, conv_shortcut=True, name=None): """A residual block. Arguments: x: input tensor. filters: integer, filters of the bottleneck layer. kernel_size: default 3, kernel size of the bottleneck layer. stride: default 1, stride of the first layer. conv_shortcut: default True, use convolution shortcut if True, otherwise identity shortcut. name: string, block label. Returns: Output tensor for the residual block. """ bn_axis = 3 if backend.image_data_format() == 'channels_last' else 1 if conv_shortcut: shortcut = layers.Conv2D( 4 * filters, 1, strides=stride, name=name + '_0_conv')(x) shortcut = layers.BatchNormalization( axis=bn_axis, epsilon=1.001e-5, name=name + '_0_bn')(shortcut) else: shortcut = x #第一个卷积结构 x = layers.Conv2D(filters, 1, strides=stride, name=name + '_1_conv')(x) x = layers.BatchNormalization( axis=bn_axis, epsilon=1.001e-5, name=name + '_1_bn')(x) x = layers.Activation('relu', name=name + '_1_relu')(x) #第二个卷积结构 x = layers.Conv2D( filters, kernel_size, padding='SAME', name=name + '_2_conv')(x) x = layers.BatchNormalization( axis=bn_axis, epsilon=1.001e-5, name=name + '_2_bn')(x) x = layers.Activation('relu', name=name + '_2_relu')(x) #第三个卷积结构 x = layers.Conv2D(4 * filters, 1, name=name + '_3_conv')(x) x = layers.BatchNormalization( axis=bn_axis, epsilon=1.001e-5, name=name + '_3_bn')(x) x = layers.Add(name=name + '_add')([shortcut, x]) x = layers.Activation('relu', name=name + '_out')(x) return x def stack1(x, filters, blocks, stride1=2, name=None): """A set of stacked residual blocks. Arguments: x: input tensor. filters: integer, filters of the bottleneck layer in a block. blocks: integer, blocks in the stacked blocks. stride1: default 2, stride of the first layer in the first block. name: string, stack label. Returns: Output tensor for the stacked blocks. """ x = block1(x, filters, stride=stride1, name=name + '_block1') for i in range(2, blocks + 1): x = block1(x, filters, conv_shortcut=False, name=name + '_block' + str(i)) return x
这是一个用于构建深度残差网络(ResNet)的函数,包含了两个子函数:block1和stack1。其中block1是一个残差块,stack1是一组堆叠的残差块。在ResNet中,每个残差块由三个卷积层组成,其中第一个卷积层可以使用1x1卷积进行下采样,第三个卷积层的输出通道数是第二个卷积层的四倍。每个残差块的输出是输入和最后一个卷积层的输出的和,并经过ReLU激活函数。stack1函数调用block1函数构建一个堆叠的残差块,可以使用stride1参数指定第一个残差块的下采样步长。
阅读全文
相关推荐
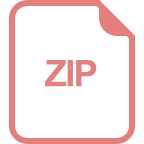
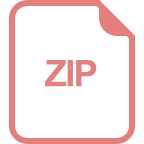













