out=img1.permute(2,0,1)
时间: 2024-06-07 10:10:10 浏览: 13
这行代码是将一个三维的张量img1的维度进行变换,使得原来的第一维度(通道数)变成了新的第二个维度,原来的第二个维度(高度)变成了新的第三个维度,原来的第三个维度(宽度)变成了新的第一个维度。这样做的目的通常是将图像的通道维度放到最前面,以方便进行后续的处理。这里的permute函数是PyTorch中的函数,用于对张量的维度进行变换。
相关问题
把下面的代码用c++实现 import numpy as np import torch import cv2 result = np.fromfile('permute_1_0.raw', dtype=np.float32) img = result.reshape((2,480,480)) torch_img = torch.from_numpy(img) index = torch.max(torch_img, dim=0)[1].numpy().astype(np.uint8) index[index > 0] = 255 # 白色 cv2.imshow('out', index) cv2.waitKey(0)
以下是用C++实现的代码:
```
#include <opencv2/opencv.hpp>
#include <torch/torch.h>
int main() {
// 读取数据
std::ifstream input("permute_1_0.raw", std::ios::binary);
std::vector<float> data(2 * 480 * 480);
input.read(reinterpret_cast<char*>(data.data()), data.size() * sizeof(float));
input.close();
// 转换数据类型
torch::Tensor tensor = torch::from_blob(data.data(), {2, 480, 480}, torch::kFloat32).clone();
// 获取最大值索引
torch::Tensor max = std::get<0>(torch::max(tensor, 0));
torch::Tensor index = max.argmax(0).to(torch::kUInt8);
// 去除背景
index.masked_fill_(index == 0, 255);
// 转换为OpenCV格式
cv::Mat out(index.sizes()[0], index.sizes()[1], CV_8UC1, index.data_ptr());
// 显示图像
cv::imshow("out", out);
cv::waitKey(0);
return 0;
}
```
注意需要安装OpenCV和PyTorch C++ API,并且需要将`permute_1_0.raw`文件放在当前目录下。
yolov5 loss.py 代码详解
yolov5 loss.py 代码详解
yolov5 loss.py 是 YOLOv5 模型中的一个关键文件,主要负责计算模型的损失函数。下面是该文件的代码详解:
1. 导入必要的库
```python
import torch
import torch.nn.functional as F
from torch import nn
```
2. 定义损失函数类
```python
class YOLOv5Loss(nn.Module):
def __init__(self, anchors, strides, iou_threshold, num_classes, img_size):
super(YOLOv5Loss, self).__init__()
self.anchors = anchors
self.strides = strides
self.iou_threshold = iou_threshold
self.num_classes = num_classes
self.img_size = img_size
```
该类继承自 nn.Module,包含了一些必要的参数,如 anchors,strides,iou_threshold,num_classes 和 img_size。
3. 定义计算损失函数的方法
```python
def forward(self, x, targets=None):
bs, _, ny, nx = x.shape # batch size, channels, grid size
na = self.anchors.shape[] # number of anchors
stride = self.img_size / max(ny, nx) # compute stride
yolo_out, grid = [], []
for i in range(3):
yolo_out.append(x[i].view(bs, na, self.num_classes + 5, ny, nx).permute(, 1, 3, 4, 2).contiguous())
grid.append(torch.meshgrid(torch.arange(ny), torch.arange(nx)))
ny, nx = ny // 2, nx // 2
loss, nGT, nCorrect, mask = , , , torch.zeros(bs, na, ny, nx)
for i in range(3):
y, g = yolo_out[i], grid[i]
y[..., :2] = (y[..., :2].sigmoid() + g) * stride # xy
y[..., 2:4] = y[..., 2:4].exp() * self.anchors[i].to(x.device) # wh
y[..., :4] *= mask.unsqueeze(-1).to(x.device)
y[..., 4:] = y[..., 4:].sigmoid()
if targets is not None:
na_t, _, _, _, _ = targets.shape
t = targets[..., 2:6] * stride
gxy = g.unsqueeze().unsqueeze(-1).to(x.device)
gi, gj = gxy[..., ], gxy[..., 1]
b = t[..., :4]
iou = box_iou(b, y[..., :4]) # iou
iou_max, _ = iou.max(2)
# Match targets to anchors
a = torch.arange(na_t).view(-1, 1).repeat(1, na)
t = targets[a, iou_max >= self.iou_threshold] # select targets
# Compute losses
if len(t):
# xy loss
xy = y[..., :2] - gxy
xy_loss = (torch.abs(xy) - .5).pow(2) * mask.unsqueeze(-1).to(x.device)
# wh loss
wh = torch.log(y[..., 2:4] / self.anchors[i].to(x.device) + 1e-16)
wh_loss = F.huber_loss(wh, t[..., 2:4], reduction='none') * mask.unsqueeze(-1).to(x.device)
# class loss
tcls = t[..., ].long()
tcls_onehot = torch.zeros_like(y[..., 5:])
tcls_onehot[torch.arange(len(t)), tcls] = 1
cls_loss = F.binary_cross_entropy(y[..., 5:], tcls_onehot, reduction='none') * mask.unsqueeze(-1).to(x.device)
# objectness loss
obj_loss = F.binary_cross_entropy(y[..., 4:5], iou_max.unsqueeze(-1), reduction='none') * mask.to(x.device)
# total loss
loss += (xy_loss + wh_loss + cls_loss + obj_loss).sum()
nGT += len(t)
nCorrect += (iou_max >= self.iou_threshold).sum().item()
mask = torch.zeros(bs, na, ny, nx)
if targets is not None:
t = targets[..., 2:6] * stride
gi, gj = g[..., ], g[..., 1]
a = targets[..., 1].long()
mask[torch.arange(bs), a, gj, gi] = 1
return loss, nGT, nCorrect
```
该方法接受输入 x 和 targets,其中 x 是模型的输出,targets 是真实标签。该方法首先根据输入 x 的形状计算出 batch size,channels,grid size 和 number of anchors 等参数,然后根据这些参数计算出 stride 和 grid。接着,该方法将输入 x 分成三个部分,每个部分都包含了 na 个 anchors 和 self.num_classes + 5 个通道。然后,该方法将每个部分的输出转换成合适的形状,并计算出每个 anchor 的中心点坐标和宽高。接着,该方法根据 targets 计算出损失函数,包括 xy loss,wh loss,class loss 和 objectness loss。最后,该方法返回损失函数的值,以及 nGT 和 nCorrect。
4. 定义计算 box iou 的方法
```python
def box_iou(box1, box2):
"""
Returns the IoU of two bounding boxes
"""
b1_x1, b1_y1, b1_x2, b1_y2 = box1[..., ], box1[..., 1], box1[..., 2], box1[..., 3]
b2_x1, b2_y1, b2_x2, b2_y2 = box2[..., ], box2[..., 1], box2[..., 2], box2[..., 3]
inter_rect_x1 = torch.max(b1_x1, b2_x1)
inter_rect_y1 = torch.max(b1_y1, b2_y1)
inter_rect_x2 = torch.min(b1_x2, b2_x2)
inter_rect_y2 = torch.min(b1_y2, b2_y2)
inter_area = torch.clamp(inter_rect_x2 - inter_rect_x1 + 1, min=) * torch.clamp(inter_rect_y2 - inter_rect_y1 + 1, min=)
b1_area = (b1_x2 - b1_x1 + 1) * (b1_y2 - b1_y1 + 1)
b2_area = (b2_x2 - b2_x1 + 1) * (b2_y2 - b2_y1 + 1)
iou = inter_area / (b1_area + b2_area - inter_area + 1e-16)
return iou
```
该方法接受两个参数 box1 和 box2,分别表示两个 bounding box 的坐标。该方法首先计算出两个 bounding box 的交集和并集,然后计算出它们的 IoU。
以上就是 yolov5 loss.py 代码的详解。
相关推荐
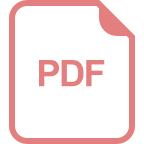
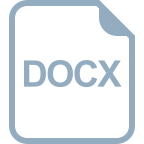












