logger.info(f'load model from: {self.pretrained}')
时间: 2023-06-19 08:06:41 浏览: 44
这行代码是使用 Python 的 logging 模块记录程序运行信息。在这里,记录了模型加载的信息,即从哪里加载预训练模型。其中,f-string 是一种Python 3.6及以上版本中的字符串格式化方式,用于将表达式嵌入到字符串中,以方便输出变量等信息。在这里,使用了 f-string 来输出 self.pretrained 变量的值。
相关问题
logger = get_root_logger() logger.info(f'load model from: {self.pretrained}')
这段代码是使用Python logging模块中的get_root_logger函数来获取一个logger对象,然后使用该logger对象记录一条INFO级别的日志,日志内容为"load model from: {self.pretrained}",其中self.pretrained是一个变量,表示预训练模型的路径。这个日志信息可以用来跟踪程序运行过程中的关键信息,以便于后续的调试和分析。
if pretrained: self.pretrained = pretrained if isinstance(self.pretrained, str): self.apply(_init_weights) logger = get_root_logger() logger.info(f'load model from: {self.pretrained}') checkpoint = torch.load(self.pretrained, map_location='cpu') state_dict = checkpoint['model'] state_dict['patch_embed.proj.weight'] = state_dict['patch_embed.proj.weight'].unsqueeze(2).repeat(1,1,self.patch_size[0],1,1) / self.patch_size[0]
这段代码是在构建模型时,如果预训练参数存在,则加载预训练参数。首先检查预训练参数是否为字符串类型,如果是,则调用_init_weights函数对模型参数进行初始化,并打印日志信息。然后使用torch.load函数加载预训练参数,其中map_location参数指定了将预训练参数加载到CPU上。接下来获取预训练参数中的模型参数,并将patch_embed.proj.weight参数重复扩展到与输入图像的分辨率相同,以便进行卷积操作。最后将参数除以patch_size[0]以进行归一化处理。
相关推荐
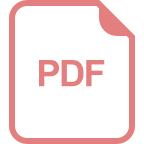
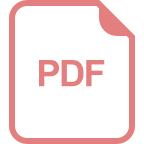









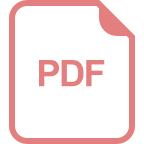
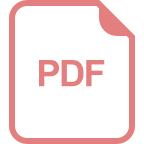
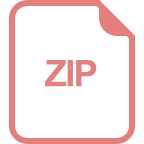
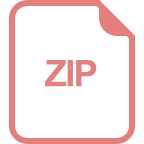