if isinstance(self.pretrained, str): self.apply(_init_weights) logger = get_root_logger() logger.info(f'load model from: {self.pretrained}') checkpoint = torch.load(self.pretrained, map_location='cpu') state_dict = checkpoint['model'] state_dict['patch_embed.proj.weight'] = state_dict['patch_embed.proj.weight'].unsqueeze(2).repeat(1,1,self.patch_size[0],1,1) / self.patch_size[0]
时间: 2023-06-18 14:04:20 浏览: 227
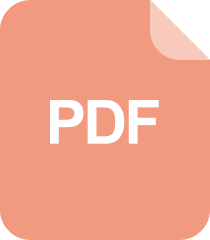
python中 _、__、__xx__()区别及使用场景
这段代码看起来是在加载预训练模型的权重,并将其中的 patch_embed 层的权重进行了修改。具体来说,它将原始的 patch_embed 层的权重(shape 为 [num_patches, embed_dim])重复复制了 self.patch_size[0] 次,变成了 [num_patches, embed_dim, patch_size[0], 1, 1] 的形状,并将每个元素除以了 self.patch_size[0]。这个操作可能是为了对输入的图像进行 patch 分割时,将每个 patch 内部的像素值均匀地分配到 patch 内部各个位置的嵌入向量中。
阅读全文
相关推荐
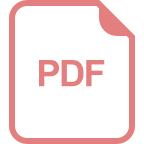
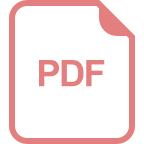

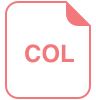
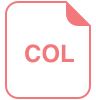
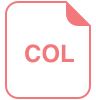
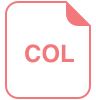
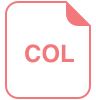
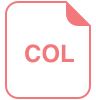
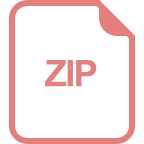
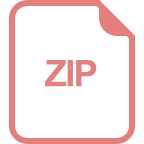
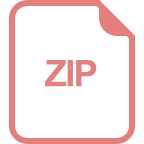
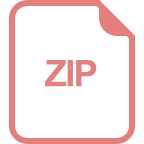