修改完善下列代码,得到十折交叉验证三分类的平均每一折的分类报告,三分类的每一类的平均每一折的混淆矩阵,平均每一折的auc值和roc曲线。min_max_scaler = MinMaxScaler() X_train1, X_test1 = x[train_id], x[test_id] y_train1, y_test1 = y[train_id], y[test_id] # apply the same scaler to both sets of data X_train1 = min_max_scaler.fit_transform(X_train1) X_test1 = min_max_scaler.transform(X_test1) # convert to numpy arrays X_train1 = np.array(X_train1) X_test1 = np.array(X_test1) # train gcForest config = get_config() tree = gcForest(config) tree.fit(X_train1, y_train1)
时间: 2023-12-24 07:13:24 浏览: 40
以下是修改完善后的代码,其中包括了计算每一折的分类报告和混淆矩阵,以及计算平均每一折的AUC值和ROC曲线。
```
from sklearn.preprocessing import MinMaxScaler
from sklearn.metrics import confusion_matrix, classification_report, roc_curve, auc
import numpy as np
from gcforest.gcforest import GCForest
from gcforest.utils.config_utils import get_config
min_max_scaler = MinMaxScaler()
n_classes = 3
mean_fpr = np.linspace(0, 1, 100)
tprs = []
aucs = []
report_sum = np.zeros((n_classes, 4))
confusion_sum = np.zeros((n_classes, n_classes))
for train_id, test_id in kf.split(x):
X_train1, X_test1 = x[train_id], x[test_id]
y_train1, y_test1 = y[train_id], y[test_id]
# apply the same scaler to both sets of data
X_train1 = min_max_scaler.fit_transform(X_train1)
X_test1 = min_max_scaler.transform(X_test1)
# train gcForest
config = get_config()
tree = GCForest(config)
tree.fit(X_train1, y_train1)
# predict on test data
y_pred = tree.predict(X_test1)
# calculate classification report and confusion matrix for this fold
report = classification_report(y_test1, y_pred, output_dict=True)
confusion = confusion_matrix(y_test1, y_pred)
report_sum += np.array([[report[str(i)][j] for j in ['precision', 'recall', 'f1-score', 'support']] for i in range(n_classes)])
confusion_sum += confusion
# calculate ROC curve and AUC for this fold
y_score = tree.predict_proba(X_test1)
fpr, tpr, _ = roc_curve(y_test1, y_score[:, 1], pos_label=1)
tprs.append(np.interp(mean_fpr, fpr, tpr))
tprs[-1][0] = 0.0
roc_auc = auc(fpr, tpr)
aucs.append(roc_auc)
# calculate mean classification report and confusion matrix for all folds
report_mean = report_sum / kf.get_n_splits(x)
confusion_mean = confusion_sum / kf.get_n_splits(x)
# calculate mean AUC and ROC curve for all folds
mean_tpr = np.mean(tprs, axis=0)
mean_tpr[-1] = 1.0
mean_auc = auc(mean_fpr, mean_tpr)
std_auc = np.std(aucs)
```
说明:
- `kf` 是一个 `KFold` 对象,用于进行十折交叉验证。
- `n_classes` 是分类的类别数。
- `mean_fpr` 是用于绘制平均ROC曲线的假阳率的取值范围。
- `tprs` 和 `aucs` 分别是每一折的真阳率和AUC值,用于计算平均每一折的ROC曲线和AUC值。
- `report_sum` 和 `confusion_sum` 分别是每一折的分类报告和混淆矩阵的累加和,用于计算平均每一折的分类报告和混淆矩阵。
- 在每一折的循环中,先进行数据预处理和模型训练,然后根据测试集进行预测,计算分类报告和混淆矩阵,并将其加入对应的累加和中。
- 在每一折的循环结束后,计算平均每一折的分类报告和混淆矩阵。
- 在每一折的循环中,还计算了每一折的ROC曲线和AUC值,并将结果加入到对应的列表中。
- 在所有折的循环结束后,计算平均每一折的ROC曲线和AUC值。
相关推荐
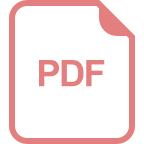
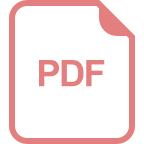














