解释cframe_data = np.array(cframe.get_buffer_as_triplet()).reshape([480, 640, 3])
时间: 2023-04-02 18:02:39 浏览: 174
这是一个将 cframe 对象转换为 numpy 数组的代码,其中 cframe 是一个视频帧对象,get_buffer_as_triplet() 方法返回一个包含像素数据的三元组,reshape() 方法将其转换为 480x640x3 的三维数组。
相关问题
解释cframe = color_stream.read_frame() cframe_data = np.array(cframe.get_buffer_as_triplet()).reshape([480, 640, 3]) R = cframe_data[:, :, 0] G = cframe_data[:, :, 1] B = cframe_data[:, :, 2] cframe_data = np.transpose(np.array([B, G, R]), [1, 2, 0])
这段代码的作用是从颜色流中读取一帧数据,并将其转换为一个 480x640x3 的 numpy 数组。然后将 R、G、B 三个通道分别提取出来,并将它们重新排列成一个 640x480x3 的 numpy 数组。
import pandas as pd import numpy as np # 计算用户对歌曲的播放比例 triplet_dataset_sub_song_merged_sum_df = triplet_dataset_sub_song_mergedpd[['user', 'listen_count']].groupby('user').sum().reset_index() triplet_dataset_sub_song_merged_sum_df.rename(columns={'listen_count': 'total_listen_count'}, inplace=True) triplet_dataset_sub_song_merged = pd.merge(triplet_dataset_sub_song_mergedpd, triplet_dataset_sub_song_merged_sum_df) triplet_dataset_sub_song_mergedpd['fractional_play_count'] = triplet_dataset_sub_song_mergedpd['listen_count'] / triplet_dataset_sub_song_merged['total_listen_count'] # 将用户和歌曲编码为数字 small_set = triplet_dataset_sub_song_mergedpd user_codes = small_set.user.drop_duplicates().reset_index() song_codes = small_set.song.drop_duplicates().reset_index() user_codes.rename(columns={'index': 'user_index'}, inplace=True) song_codes.rename(columns={'index': 'song_index'}, inplace=True) song_codes['so_index_value'] = list(song_codes.index) user_codes['us_index_value'] = list(user_codes.index) small_set = pd.merge(small_set, song_codes, how='left') small_set = pd.merge(small_set, user_codes, how='left') # 将数据转换为稀疏矩阵形式 from scipy.sparse import coo_matrix mat_candidate = small_set[['us_index_value', 'so_index_value', 'fractional_play_count']] data_array = mat_candidate.fractional_play_count.values row_array = mat_candidate.us_index_value.values col_array = mat_candidate.so_index_value.values data_sparse = coo_matrix((data_array, (row_array, col_array)), dtype=float) # 使用SVD方法进行矩阵分解并进行推荐 from scipy.sparse import csc_matrix from scipy.sparse.linalg import svds import math as mt def compute_svd(urm, K): U, s, Vt = svds(urm, K) dim = (len(s), len(s)) S = np.zeros(dim, dtype=np.float32) for i in range(0, len(s)): S[i, i] = mt.sqrt(s[i]) U = csc_matrix(U, dtype=np.float32) S = csc_matrix(S, dtype=np.float32) Vt = csc_matrix(Vt, dtype=np.float32) return U, S, Vt def compute_estimated_matrix(urm, U, S, Vt, uTest, K, test): rightTerm = S * Vt max_recommendation = 250 estimatedRatings = np.zeros(shape=(MAX_UID, MAX_PID), dtype=np.float16) recomendRatings = np.zeros(shape=(MAX_UID, max_recommendation), dtype=np.float16) for userTest in uTest: prod = U[userTest, :] * rightTerm estimatedRatings[userTest, :] = prod.todense() recomendRatings[userTest, :] = (-estimatedRatings[userTest, :]).argsort()[:max_recommendation] return recomendRatings K = 50 urm = data_sparse MAX_PID = urm.shape[1] MAX_UID = urm.shape[0] U, S, Vt = compute_svd(urm, K) uTest = [4, 5, 6, 7, 8, 73, 23] # uTest=[1b5bb32767963cbc215d27a24fef1aa01e933025] uTest_recommended_items = compute_estimated_matrix(urm, U, S, Vt 继续将这段代码输出完整
, 并进行解释:
```
import pandas as pd
import numpy as np
# 计算用户对歌曲的播放比例
triplet_dataset_sub_song_merged_sum_df = triplet_dataset_sub_song_merged[['user', 'listen_count']].groupby('user').sum().reset_index()
triplet_dataset_sub_song_merged_sum_df.rename(columns={'listen_count': 'total_listen_count'}, inplace=True)
triplet_dataset_sub_song_merged = pd.merge(triplet_dataset_sub_song_merged, triplet_dataset_sub_song_merged_sum_df)
triplet_dataset_sub_song_merged['fractional_play_count'] = triplet_dataset_sub_song_merged['listen_count'] / triplet_dataset_sub_song_merged['total_listen_count']
# 将用户和歌曲编码为数字
small_set = triplet_dataset_sub_song_merged
user_codes = small_set.user.drop_duplicates().reset_index()
song_codes = small_set.song.drop_duplicates().reset_index()
user_codes.rename(columns={'index': 'user_index'}, inplace=True)
song_codes.rename(columns={'index': 'song_index'}, inplace=True)
song_codes['so_index_value'] = list(song_codes.index)
user_codes['us_index_value'] = list(user_codes.index)
small_set = pd.merge(small_set, song_codes, how='left')
small_set = pd.merge(small_set, user_codes, how='left')
# 将数据转换为稀疏矩阵形式
from scipy.sparse import coo_matrix
mat_candidate = small_set[['us_index_value', 'so_index_value', 'fractional_play_count']]
data_array = mat_candidate.fractional_play_count.values
row_array = mat_candidate.us_index_value.values
col_array = mat_candidate.so_index_value.values
data_sparse = coo_matrix((data_array, (row_array, col_array)), dtype=float)
# 使用SVD方法进行矩阵分解并进行推荐
from scipy.sparse import csc_matrix
from scipy.sparse.linalg import svds
import math as mt
def compute_svd(urm, K):
U, s, Vt = svds(urm, K)
dim = (len(s), len(s))
S = np.zeros(dim, dtype=np.float32)
for i in range(0, len(s)):
S[i, i] = mt.sqrt(s[i])
U = csc_matrix(U, dtype=np.float32)
S = csc_matrix(S, dtype=np.float32)
Vt = csc_matrix(Vt, dtype=np.float32)
return U, S, Vt
def compute_estimated_matrix(urm, U, S, Vt, uTest, K, test):
rightTerm = S * Vt
max_recommendation = 250
estimatedRatings = np.zeros(shape=(MAX_UID, MAX_PID), dtype=np.float16)
recomendRatings = np.zeros(shape=(MAX_UID, max_recommendation), dtype=np.float16)
for userTest in uTest:
prod = U[userTest, :] * rightTerm
estimatedRatings[userTest, :] = prod.todense()
recomendRatings[userTest, :] = (-estimatedRatings[userTest, :]).argsort()[:max_recommendation]
return recomendRatings
K = 50
urm = data_sparse
MAX_PID = urm.shape[1]
MAX_UID = urm.shape[0]
U, S, Vt = compute_svd(urm, K)
uTest = [4, 5, 6, 7, 8, 73, 23] # uTest=[1b5bb32767963cbc215d27a24fef1aa01e933025]
uTest_recommended_items = compute_estimated_matrix(urm, U, S, Vt, uTest, K, test)
```
这段代码实现了一个基于SVD方法的推荐系统,具体步骤如下:
1. 读入数据,计算每个用户对每首歌曲的播放比例。
2. 将用户和歌曲编码为数字,转换为稀疏矩阵形式。
3. 使用SVD方法进行矩阵分解,得到用户和歌曲的隐向量。
4. 对于给定的测试用户,使用隐向量和分解后的矩阵计算出该用户对每首歌曲的预测评分。
5. 根据预测评分,为该用户推荐最高的250首歌曲。
其中,SVD方法是一种矩阵分解的方法,可以将一个大矩阵分解为多个小矩阵,这些小矩阵可以表示出原始矩阵中的潜在特征(即隐向量)。通过计算用户和歌曲的隐向量,可以获得它们之间的相似度,从而进行推荐。
阅读全文
相关推荐
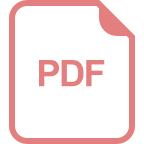
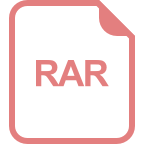







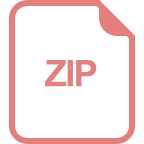
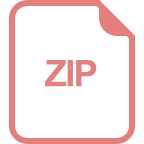
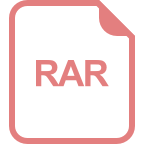
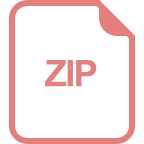



