shufflenet分类pytorch代码
时间: 2023-07-05 09:28:30 浏览: 48
下面是使用PyTorch实现ShuffleNet的分类代码:
```python
import torch
import torch.nn as nn
class ShuffleNetBlock(nn.Module):
def __init__(self, inp, oup, mid_channels, ksize, stride):
super(ShuffleNetBlock, self).__init__()
self.stride = stride
self.mid_channels = mid_channels
self.inp = inp
self.oup = oup
assert stride in [1, 2]
if stride == 2:
self.branch1 = nn.Sequential(
nn.Conv2d(inp, inp, 3, 2, 1, groups=inp, bias=False),
nn.BatchNorm2d(inp),
nn.Conv2d(inp, mid_channels, 1, 1, 0, bias=False),
nn.BatchNorm2d(mid_channels),
nn.ReLU(inplace=True),
)
self.branch2 = nn.Sequential(
nn.Conv2d(inp, mid_channels, 1, 1, 0, bias=False),
nn.BatchNorm2d(mid_channels),
nn.ReLU(inplace=True),
nn.Conv2d(mid_channels, mid_channels, ksize, stride, ksize//2, groups=mid_channels, bias=False),
nn.BatchNorm2d(mid_channels),
nn.Conv2d(mid_channels, mid_channels, 1, 1, 0, bias=False),
nn.BatchNorm2d(mid_channels),
nn.ReLU(inplace=True),
)
else:
assert inp == oup
self.branch1 = nn.Sequential()
self.branch2 = nn.Sequential(
nn.Conv2d(mid_channels, mid_channels, ksize, stride, ksize//2, groups=mid_channels, bias=False),
nn.BatchNorm2d(mid_channels),
nn.Conv2d(mid_channels, oup, 1, 1, 0, bias=False),
nn.BatchNorm2d(oup),
nn.ReLU(inplace=True),
)
def forward(self, x):
if self.stride == 1:
x1, x2 = x.chunk(2, dim=1)
out = torch.cat((x1, self.branch2(x2)), dim=1)
else:
out = torch.cat((self.branch1(x), self.branch2(x)), dim=1)
return out
class ShuffleNet(nn.Module):
def __init__(self, num_classes=1000):
super(ShuffleNet, self).__init__()
self.conv1 = nn.Conv2d(3, 24, 3, 2, 1, bias=False)
self.bn1 = nn.BatchNorm2d(24)
self.maxpool = nn.MaxPool2d(3, 2, 1)
self.stage2 = self._make_stage(24, 144, 3, 2)
self.stage3 = self._make_stage(144, 288, 7, 2)
self.stage4 = self._make_stage(288, 576, 3, 2)
self.conv5 = nn.Conv2d(576, 1024, 1, 1, 0, bias=False)
self.bn5 = nn.BatchNorm2d(1024)
self.fc = nn.Linear(1024, num_classes)
def _make_stage(self, inp, oup, ksize, stride):
layers = []
layers.append(ShuffleNetBlock(inp, oup, int(oup/2), ksize, stride))
for i in range(1, 4):
layers.append(ShuffleNetBlock(oup, oup, int(oup/2), ksize, 1))
return nn.Sequential(*layers)
def forward(self, x):
x = self.conv1(x)
x = self.bn1(x)
x = nn.ReLU(inplace=True)(x)
x = self.maxpool(x)
x = self.stage2(x)
x = self.stage3(x)
x = self.stage4(x)
x = self.conv5(x)
x = self.bn5(x)
x = nn.ReLU(inplace=True)(x)
x = x.mean([2, 3])
x = self.fc(x)
return x
```
其中,ShuffleNetBlock是ShuffleNet的基本模块,_make_stage是构建ShuffleNet每个阶段的函数,ShuffleNet是整个模型的定义。
可以使用以下代码来进行模型的实例化和训练:
```python
import torch.optim as optim
import torchvision.datasets as datasets
import torchvision.transforms as transforms
# 实例化模型
model = ShuffleNet()
# 定义损失函数和优化器
criterion = nn.CrossEntropyLoss()
optimizer = optim.SGD(model.parameters(), lr=0.1, momentum=0.9, weight_decay=0.0001)
# 加载数据集
train_dataset = datasets.ImageFolder(root='./train', transform=transforms.ToTensor())
train_loader = torch.utils.data.DataLoader(train_dataset, batch_size=32, shuffle=True, num_workers=4)
# 训练模型
for epoch in range(100):
for i, (inputs, targets) in enumerate(train_loader):
optimizer.zero_grad()
outputs = model(inputs)
loss = criterion(outputs, targets)
loss.backward()
optimizer.step()
if i % 10 == 0:
print('Epoch: %d, Batch: %d, Loss: %.3f' % (epoch+1, i, loss.item()))
```
其中,train文件夹下存放的是训练数据集,每个子文件夹代表一个类别。可以使用torchvision.transforms对数据进行预处理,例如将图像转换为tensor、对图像进行随机裁剪、随机翻转等。在训练过程中,使用优化器对模型的参数进行更新,同时计算损失函数,以监督模型的训练效果。
相关推荐
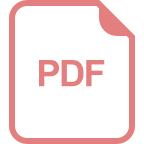
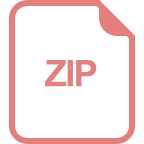















