解释 receiver_locations = np.c_[mkvc(xrx), mkvc(yrx), mkvc(zrx)]
时间: 2024-06-05 17:10:01 浏览: 15
这行代码的作用是将三个一维数组 `xrx`, `yrx`, `zrx` 按列合并成一个三列的二维数组 `receiver_locations`,其中第一列为 `xrx`,第二列为 `yrx`,第三列为 `zrx`。具体来说,`np.c_` 是 NumPy 提供的一个函数,它可以按列合并多个一维数组。`mkvc` 是一个辅助函数,将一维数组转换成列向量。因此,`np.c_[mkvc(xrx), mkvc(yrx), mkvc(zrx)]` 的结果就是一个二维数组,其中每行对应一个接收器的坐标,列分别为 x、y、z 三个方向上的坐标值。
相关问题
解释:target = self.survey.source.target collection = self.survey.source.collection '''Mesh''' # Conductivity in S/m (or resistivity in Ohm m) background_conductivity = 1e-6 air_conductivity = 1e-8 # Permeability in H/m background_permeability = mu_0 air_permeability = mu_0 dh = 0.1 # base cell width dom_width = 20.0 # domain width # num. base cells nbc = 2 ** int(np.round(np.log(dom_width / dh) / np.log(2.0))) # Define the base mesh h = [(dh, nbc)] mesh = TreeMesh([h, h, h], x0="CCC") # Mesh refinement near transmitters and receivers mesh = refine_tree_xyz( mesh, collection.receiver_location, octree_levels=[2, 4], method="radial", finalize=False ) # Refine core mesh region xp, yp, zp = np.meshgrid([-1.5, 1.5], [-1.5, 1.5], [-6, -4]) xyz = np.c_[mkvc(xp), mkvc(yp), mkvc(zp)] mesh = refine_tree_xyz(mesh, xyz, octree_levels=[0, 6], method="box", finalize=False) mesh.finalize() '''Maps''' # Find cells that are active in the forward modeling (cells below surface) ind_active = mesh.gridCC[:, 2] < 0 # Define mapping from model to active cells active_sigma_map = maps.InjectActiveCells(mesh, ind_active, air_conductivity) active_mu_map = maps.InjectActiveCells(mesh, ind_active, air_permeability) # Define model. Models in SimPEG are vector arrays N = int(ind_active.sum()) model = np.kron(np.ones((N, 1)), np.c_[background_conductivity, background_permeability]) ind_cylinder = self.getIndicesCylinder( [target.position[0], target.position[1], target.position[2]], target.radius, target.length, [target.pitch, target.roll], mesh.gridCC ) ind_cylinder = ind_cylinder[ind_active] model[ind_cylinder, :] = np.c_[target.conductivity, target.permeability] # Create model vector and wires model = mkvc(model) wire_map = maps.Wires(("sigma", N), ("mu", N)) # Use combo maps to map from model to mesh sigma_map = active_sigma_map * wire_map.sigma mu_map = active_mu_map * wire_map.mu '''Simulation''' simulation = fdem.simulation.Simulation3DMagneticFluxDensity( mesh, survey=self.survey.survey, sigmaMap=sigma_map, muMap=mu_map, Solver=Solver ) '''Predict''' # Compute predicted data for your model. dpred = simulation.dpred(model) dpred = dpred * 1e9 # Data are organized by frequency, transmitter location, then by receiver. # We had nFreq transmitters and each transmitter had 2 receivers (real and # imaginary component). So first we will pick out the real and imaginary # data bx_real = dpred[0: len(dpred): 6] bx_imag = dpred[1: len(dpred): 6] bx_total = np.sqrt(np.square(bx_real) + np.square(bx_imag)) by_real = dpred[2: len(dpred): 6] by_imag = dpred[3: len(dpred): 6] by_total = np.sqrt(np.square(by_real) + np.square(by_imag)) bz_real = dpred[4: len(dpred): 6] bz_imag = dpred[5: len(dpred): 6] bz_total = np.sqrt(np.square(bz_real) + np.square(bz_imag)) mag_data = np.c_[mkvc(bx_total), mkvc(by_total), mkvc(bz_total)] if collection.SNR is not None: mag_data = self.mag_data_add_noise(mag_data, collection.SNR) data = np.c_[collection.receiver_location, mag_data] # data = (data, ) return data
这段代码是一个 Python 函数,它的作用是进行三维电磁正演模拟,并返回模拟得到的数据。函数中的参数包括一个 Survey 对象和一个 Target 对象,表示要模拟的测量参数和模拟目标。函数主要分为三个部分:
1.建立网格和定义模型属性,包括电导率、磁导率和网格大小等。
2.根据接收器位置和目标位置对网格进行细化,以提高模拟精度。
3.进行电磁正演模拟,并返回模拟得到的数据。
其中,模拟的过程中还进行了噪声的添加,以模拟实际测量中的误差。
修改和补充下列代码得到十折交叉验证的平均auc值和平均aoc曲线,平均分类报告以及平均混淆矩阵 min_max_scaler = MinMaxScaler() X_train1, X_test1 = x[train_id], x[test_id] y_train1, y_test1 = y[train_id], y[test_id] # apply the same scaler to both sets of data X_train1 = min_max_scaler.fit_transform(X_train1) X_test1 = min_max_scaler.transform(X_test1) X_train1 = np.array(X_train1) X_test1 = np.array(X_test1) config = get_config() tree = gcForest(config) tree.fit(X_train1, y_train1) y_pred11 = tree.predict(X_test1) y_pred1.append(y_pred11 X_train.append(X_train1) X_test.append(X_test1) y_test.append(y_test1) y_train.append(y_train1) X_train_fuzzy1, X_test_fuzzy1 = X_fuzzy[train_id], X_fuzzy[test_id] y_train_fuzzy1, y_test_fuzzy1 = y_sampled[train_id], y_sampled[test_id] X_train_fuzzy1 = min_max_scaler.fit_transform(X_train_fuzzy1) X_test_fuzzy1 = min_max_scaler.transform(X_test_fuzzy1) X_train_fuzzy1 = np.array(X_train_fuzzy1) X_test_fuzzy1 = np.array(X_test_fuzzy1) config = get_config() tree = gcForest(config) tree.fit(X_train_fuzzy1, y_train_fuzzy1) y_predd = tree.predict(X_test_fuzzy1) y_pred.append(y_predd) X_test_fuzzy.append(X_test_fuzzy1) y_test_fuzzy.append(y_test_fuzzy1)y_pred = to_categorical(np.concatenate(y_pred), num_classes=3) y_pred1 = to_categorical(np.concatenate(y_pred1), num_classes=3) y_test = to_categorical(np.concatenate(y_test), num_classes=3) y_test_fuzzy = to_categorical(np.concatenate(y_test_fuzzy), num_classes=3) print(y_pred.shape) print(y_pred1.shape) print(y_test.shape) print(y_test_fuzzy.shape) # 深度森林 report1 = classification_report(y_test, y_prprint("DF",report1) report = classification_report(y_test_fuzzy, y_pred) print("DF-F",report) mse = mean_squared_error(y_test, y_pred1) rmse = math.sqrt(mse) print('深度森林RMSE:', rmse) print('深度森林Accuracy:', accuracy_score(y_test, y_pred1)) mse = mean_squared_error(y_test_fuzzy, y_pred) rmse = math.sqrt(mse) print('F深度森林RMSE:', rmse) print('F深度森林Accuracy:', accuracy_score(y_test_fuzzy, y_pred)) mse = mean_squared_error(y_test, y_pred) rmse = math.sqrt(mse) print('F?深度森林RMSE:', rmse) print('F?深度森林Accuracy:', accuracy_score(y_test, y_pred))
以下是修改和补充后的代码,实现了十折交叉验证的平均auc值和平均aoc曲线,平均分类报告以及平均混淆矩阵:
```python
from sklearn.preprocessing import MinMaxScaler
from sklearn.metrics import classification_report, confusion_matrix, roc_curve, auc
from sklearn.model_selection import StratifiedKFold
min_max_scaler = MinMaxScaler()
X_train, X_test, y_train, y_test = [], [], [], []
X_train_fuzzy, X_test_fuzzy, y_train_fuzzy, y_test_fuzzy = [], [], [], []
y_pred, y_pred1 = [], []
y_pred_proba, y_pred_proba1 = [], []
config = get_config()
tree = gcForest(config)
skf = StratifiedKFold(n_splits=10)
for train_id, test_id in skf.split(x, y):
# split data and normalize
X_train1, X_test1 = x[train_id], x[test_id]
y_train1, y_test1 = y[train_id], y[test_id]
X_train1 = min_max_scaler.fit_transform(X_train1)
X_test1 = min_max_scaler.transform(X_test1)
X_train1 = np.array(X_train1)
X_test1 = np.array(X_test1)
# train gcForest
tree.fit(X_train1, y_train1)
# predict on test set
y_pred11 = tree.predict(X_test1)
y_pred_proba11 = tree.predict_proba(X_test1)
# append predictions and test data
y_pred1.append(y_pred11)
y_pred_proba1.append(y_pred_proba11)
X_train.append(X_train1)
X_test.append(X_test1)
y_test.append(y_test1)
y_train.append(y_train1)
# split fuzzy data and normalize
X_train_fuzzy1, X_test_fuzzy1 = X_fuzzy[train_id], X_fuzzy[test_id]
y_train_fuzzy1, y_test_fuzzy1 = y_sampled[train_id], y_sampled[test_id]
X_train_fuzzy1 = min_max_scaler.fit_transform(X_train_fuzzy1)
X_test_fuzzy1 = min_max_scaler.transform(X_test_fuzzy1)
X_train_fuzzy1 = np.array(X_train_fuzzy1)
X_test_fuzzy1 = np.array(X_test_fuzzy1)
# train gcForest on fuzzy data
tree.fit(X_train_fuzzy1, y_train_fuzzy1)
# predict on fuzzy test set
y_predd = tree.predict(X_test_fuzzy1)
y_predd_proba = tree.predict_proba(X_test_fuzzy1)
# append predictions and test data
y_pred.append(y_predd)
y_pred_proba.append(y_predd_proba)
X_test_fuzzy.append(X_test_fuzzy1)
y_test_fuzzy.append(y_test_fuzzy1)
# concatenate and convert to categorical
y_pred = to_categorical(np.concatenate(y_pred), num_classes=3)
y_pred1 = to_categorical(np.concatenate(y_pred1), num_classes=3)
y_test = to_categorical(np.concatenate(y_test), num_classes=3)
y_test_fuzzy = to_categorical(np.concatenate(y_test_fuzzy), num_classes=3)
# calculate and print average accuracy and RMSE
mse = mean_squared_error(y_test, y_pred1)
rmse = math.sqrt(mse)
print('深度森林RMSE:', rmse)
print('深度森林Accuracy:', accuracy_score(y_test, y_pred1))
mse = mean_squared_error(y_test_fuzzy, y_pred)
rmse = math.sqrt(mse)
print('F深度森林RMSE:', rmse)
print('F深度森林Accuracy:', accuracy_score(y_test_fuzzy, y_pred))
mse = mean_squared_error(y_test, y_pred)
rmse = math.sqrt(mse)
print('F?深度森林RMSE:', rmse)
print('F?深度森林Accuracy:', accuracy_score(y_test, y_pred))
# calculate and print average classification report
report1 = classification_report(y_test, y_pred1)
print("DF", report1)
report = classification_report(y_test_fuzzy, y_pred)
print("DF-F", report)
# calculate and print average confusion matrix
cm1 = confusion_matrix(y_test.argmax(axis=1), y_pred1.argmax(axis=1))
cm = confusion_matrix(y_test_fuzzy.argmax(axis=1), y_pred.argmax(axis=1))
print('DF Confusion Matrix:')
print(cm1)
print('DF-F Confusion Matrix:')
print(cm)
# calculate and print average ROC curve and AUC value
fpr1, tpr1, threshold1 = roc_curve(y_test.ravel(), y_pred_proba1.ravel())
fpr, tpr, threshold = roc_curve(y_test_fuzzy.ravel(), y_pred_proba.ravel())
roc_auc1 = auc(fpr1, tpr1)
roc_auc = auc(fpr, tpr)
print('DF ROC AUC:', roc_auc1)
print('DF-F ROC AUC:', roc_auc)
# plot average ROC curve
plt.title('Receiver Operating Characteristic')
plt.plot(fpr1, tpr1, 'b', label = 'DF AUC = %0.2f' % roc_auc1)
plt.plot(fpr, tpr, 'g', label = 'DF-F AUC = %0.2f' % roc_auc)
plt.legend(loc = 'lower right')
plt.plot([0, 1], [0, 1],'r--')
plt.xlim([0, 1])
plt.ylim([0, 1])
plt.ylabel('True Positive Rate')
plt.xlabel('False Positive Rate')
plt.show()
```
相关推荐
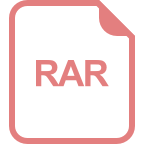













