svm三分类python鸢尾花并且画出对应ROC曲线和混淆矩阵
时间: 2023-08-07 18:01:46 浏览: 164
要画出SVM在鸢尾花数据集上的三分类的ROC曲线和混淆矩阵,你可以使用Python中的scikit-learn库和matplotlib库来实现。以下是一个示例代码:
```python
from sklearn import datasets
from sklearn.model_selection import train_test_split
from sklearn.svm import SVC
from sklearn.metrics import roc_curve, roc_auc_score, confusion_matrix
import matplotlib.pyplot as plt
import seaborn as sns
# 加载鸢尾花数据集
iris = datasets.load_iris()
X = iris.data
y = iris.target
# 将数据集拆分为训练集和测试集
X_train, X_test, y_train, y_test = train_test_split(X, y, test_size=0.2, random_state=42)
# 创建SVM分类器
svm = SVC(kernel='linear', C=1.0)
# 在训练集上训练分类器
svm.fit(X_train, y_train)
# 在测试集上进行预测
y_pred = svm.predict(X_test)
# 计算ROC曲线和AUC值
y_scores = svm.decision_function(X_test)
fpr, tpr, thresholds = roc_curve(y_test, y_scores)
auc = roc_auc_score(y_test, y_scores)
# 绘制ROC曲线
plt.plot(fpr, tpr, label='ROC curve (area = %0.2f)' % auc)
plt.plot([0, 1], [0, 1], 'k--')
plt.xlim([0.0, 1.0])
plt.ylim([0.0, 1.05])
plt.xlabel('False Positive Rate')
plt.ylabel('True Positive Rate')
plt.title('Receiver Operating Characteristic')
plt.legend(loc="lower right")
plt.show()
# 计算混淆矩阵
cm = confusion_matrix(y_test, y_pred)
# 绘制混淆矩阵热图
sns.heatmap(cm, annot=True, fmt='d', cmap='Blues')
plt.title('Confusion Matrix')
plt.xlabel('Predicted Label')
plt.ylabel('True Label')
plt.show()
```
在上述代码中,我们首先加载鸢尾花数据集,并将数据集拆分为训练集和测试集。然后,我们创建了一个线性核函数的SVM分类器,并在训练集上进行训练。接下来,我们使用测试集进行预测,并计算了ROC曲线的假阳率(False Positive Rate)、真阳率(True Positive Rate)以及相应的阈值。然后,我们计算了AUC值,并绘制了ROC曲线。最后,我们计算了混淆矩阵,并使用热图将其可视化。
请注意,上述代码中的SVM分类器使用的是线性核函数(kernel='linear'),你可以根据需要选择其他核函数,如高斯核函数(kernel='rbf')。此外,你还可以调整C参数的值来改变模型的惩罚力度,以达到更好的分类效果。
阅读全文
相关推荐
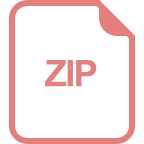
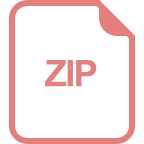
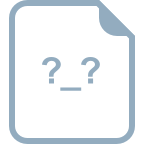
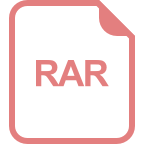
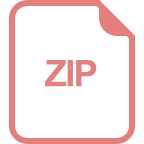
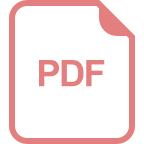
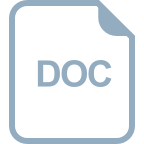
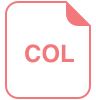
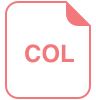







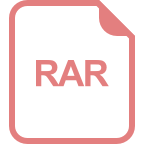